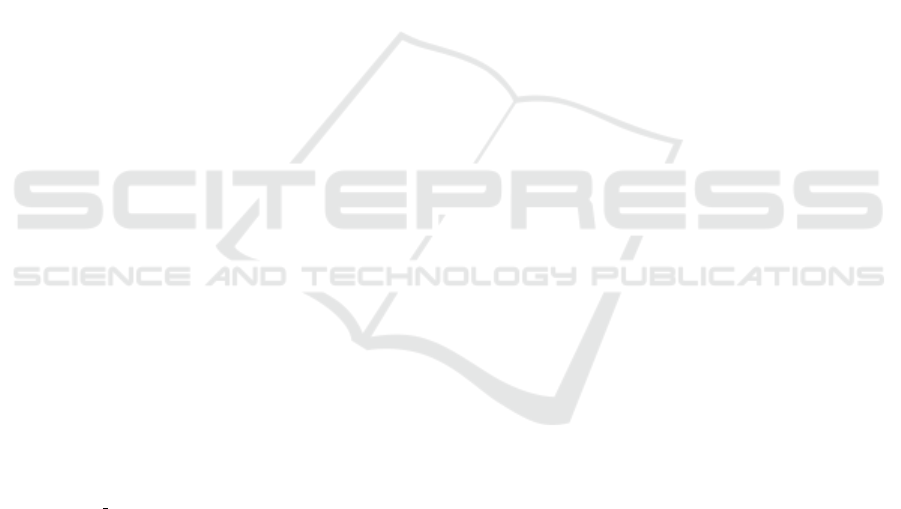
REFERENCES
Agresti, A., (2012). Categorical Data Analysis. 3rd Ed. John
Wiley & Sons.
Agresti, A., (2018). An Introduction to Categorical Data
Analysis. 3rd Ed. Wiley, 2018.
Anderson, D. R., Sweeney, D. J., Williams, T. A., Camm,
J. D., Cochran, J. J., (2017). Essentials of Modern
Business Statistics with Microsoft Office Excel (Book
Only) 7th Edition. Cengage Learning.
Armstrong, B.G., Gasparrini, A., Tobias, A., (2014). Condi-
tional Poisson models: a flexible alternative to condi-
tional logistic case cross-over analysis. BMC Medical
Research Methodology, 14:122.
Bejleri, V., Nandram, B., (2018). Bayesian and frequentist
prediction limits for the Poisson distribution. Commu-
nications in Statistics - Theory and Methods, 47:17,
4254-4271.
Congdon, P., (2005). Bayesian Models for Categorical Data.
John Wiley & Sons.
Dash, R., Paramguru, R., Dash, R., (2011). Comparative
analysis of supervised and unsupervised discretization
techniques. International Journal of Advances in Sci-
ence and Technology, 2(3): 29:37.
Diallo, A. O., Diop, A., Dupuy, J.-F., (2018). Analysis of
multinomial counts with joint zero-inflation, with an
application to health economics, Journal of Statistical
Planning and Inference, vol. 194, p. 85-105.
Doane, D., Seward, L. (2010). Applied Statistics in Busi-
ness and Economics, 3rd Edition, Mcgraw-Hill.
Donnelly Jr., R., (2019). Business Statistics 3rd Edition,
Pearson.
Falissard, B., (2012). Analysis of Questionnaire Data with
R. Chapman & Hall/CRC, Boca Raton.
Forsyth, D., (2019). Applied Machine Learning. Springer.
Gelman, A., Carlin, J. B., Stern, H. S., Dunson, D. B., Ve-
htari, A., Rubin, D. B., (2013). Bayesian Data Ana-
lysis (Chapman & Hall/CRC Texts in Statistical Sci-
ence), 3rd ed., Chapman and Hall/CRC.
Guenni L.B., (2011). Poisson Distribution and Its Appli-
cation in Statistics. In: Lovric M. (eds) International
Encyclopedia of Statistical Science. Springer, Berlin,
Heidelberg. https://doi.org/10.1007/978-3-642-
04898-2 448
Gupta, A., Mehrotra, K., Mohan, C. K., (2010). A
clustering-based discretization for supervised learn-
ing. Statistics & Probability Letters, 80(9-10): 816-
824.
Gupta, M. R. and Chen, Y., (2011). Theory and Use of
the EM Method. (Foundations and Trends(r) in Sig-
nal Processing). Now Publishers Inc.
Heeringa, S.G., West, B.T., Berglung, P.A., (2010). Applied
Survey Data Analysis. Chapman & Hall/CRC.
Jaggia, S., Kelly, A., (2018). Business Statistics: Communi-
cating with Numbers. 3rd Edition. McGraw-Hill Edu-
cation.
Jozov
´
a,
ˇ
S., Uglickich, E., Nagy, I., (2021). Bayesian mix-
ture estimation without tears. In: Proceedings of the
18th International Conference on Informatics in Con-
trol, Automation and Robotics (ICINCO 2021), ac-
cepted.
K
´
arn
´
y, M., B
¨
ohm, J., Guy, T.V., Jirsa, L., Nagy, I., Nedoma,
P., Tesa
ˇ
r, L., (2006). Optimized Bayesian Dynamic
Advising: Theory and Algorithms. Springer, London.
K
´
arn
´
y, M., Kadlec, J., Sutanto, E.L., (1998). Quasi-Bayes
estimation applied to normal mixture. Preprints of the
3rd European IEEE Workshop on Computer-Intensive
Methods in Control and Data Processing, Eds: J.
Roj
´
ı
ˇ
cek, M. Vale
ˇ
ckov
´
a, M. K
´
arn
´
y, K. Warwick, p. 77–
82, CMP ’98 /3./, Prague, CZ, 07.09.1998–09.09.
Kianmehr, K., Alshalalfa, M., Alhajj, R., (2010). Fuzzy
clustering-based discretization for gene expression
classification. Knowledge and Information Systems,
vol. 24, 441-465.
Levine, D. M., Stephan, D.F., Krehbiel, T.C., Berenson,
M.L., (2011). Statistics for Managers Using Microsoft
r
Excel, Sixth Edition. Boston, MA: Prentice Hall.
Li, Y., Sha, Y., Zhao, R., (2010). Poisson prediction of the
loss of teachers in high schools. In: Proceedings of
2010 International Conference on Multimedia Tech-
nology, Ningbo, China, pp. 1-3.
Lim, H. K., Li, W. K., Yu, P. L.H., (2014). Zero-inflated
Poisson regression mixture model, Computational
Statistics & Data Analysis, vol. 71, p. 151-158.
Long, J. S., Freese, J., (2014). Regression Models for Cat-
egorical Dependent Variables Using Stata. 3rd Ed.
Stata Press.
Nagy, I., Suzdaleva, E., 2017. Algorithms and Programs
of Dynamic Mixture Estimation. Unified Approach
to Different Types of Components, SpringerBriefs in
Statistics. Springer International Publishing, 2017.
Nagy, I., Suzdaleva, E., K
´
arn
´
y, M., Mlyn
´
a
ˇ
rov
´
a, T., (2011).
Bayesian estimation of dynamic finite mixtures. Inter-
national Journal of Adaptive Control and Signal Pro-
cessing. 25(9): 765-787.
Nagy, I., Suzdaleva, E. and Pecherkov
´
a, P., (2016). Com-
parison of various definitions of proximity in mix-
ture estimation. In: Proceedings of the 13th Interna-
tional Conference on Informatics in Control, Automa-
tion and Robotics (ICINCO 2016), pp. 527-534.
Perrakis, K., Karlis, D., Cools, M., Janssens, D.,
(2015). Bayesian inference for transportation origin-
destination matrices: the Poisson-inverse Gaussian
and other Poisson mixtures. Journal of the Royal Sta-
tistical Society: Series A (Statistics in Society), vol.
178, p. 271-296.
Peterka, V., (1981). Bayesian system identification, in
Eykhoff, P. (Ed.), Trends and Progress in System Iden-
tification. Oxford, Pergamon Press, pp. 239-304.
Petrou
ˇ
s, M., Suzdaleva, E., Nagy, I., (2019). Modeling of
passenger demand using mixture of Poisson compo-
nents. In: Proceedings of the 16th International Con-
ference on Informatics in Control, Automation and
Robotics (ICINCO 2019), p. 617-624.
Petrou
ˇ
s, M. and Uglickich, E., (2020). Modeling of mixed
data for Poisson prediction. In: Proceedings of IEEE
14th International Symposium on Applied Computa-
Prediction of Multimodal Poisson Variable using Discretization of Gaussian Data
607