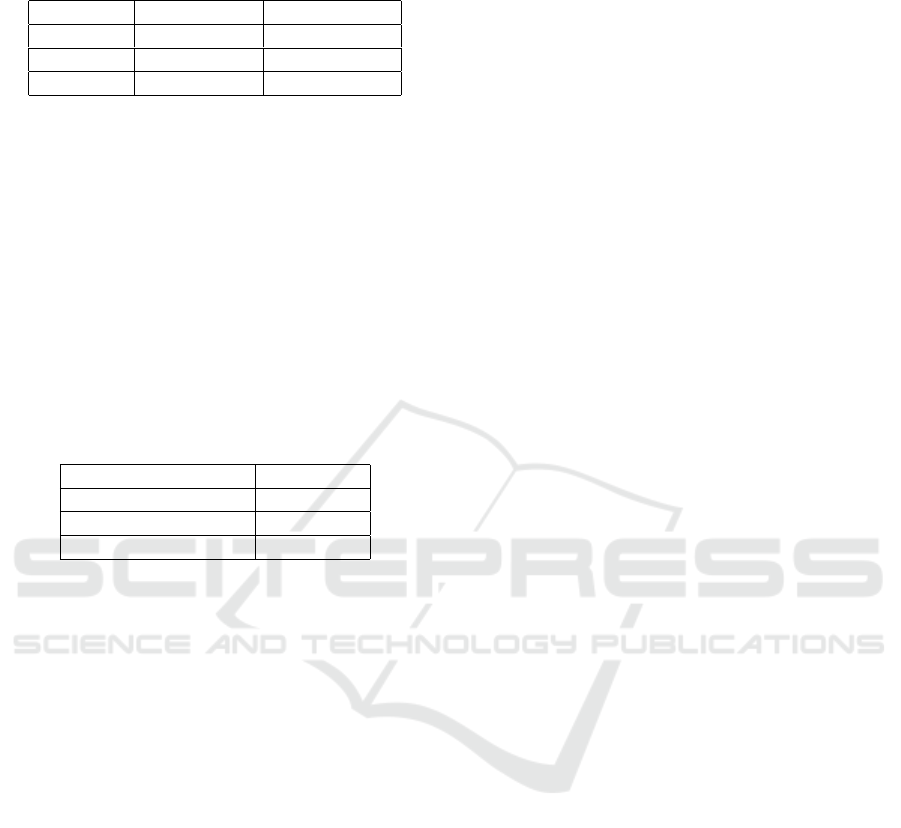
Table 1: Value of the consensus value (GSR) for the 3
matching algorithms and for the 2 scenarios.
Matching Scenario 3/5 Scenario 3/10
S
1
79,8% 78,9%
S
2
80,4% 80,4%
S
3
79,4% 78,7%
We tried to improve the previous results by optimizing
the decision thresholds. The question we wanted to
answer is to know if it was possible to define common
values of T
1
and T
2
for the 5 passwords. We tested dif-
ferent threshold values between the minimal and max-
imal values for the 5 passwords. Table 2 presents the
obtained results by optimizing the thresholds. Note
that we used the testing scenario 3/10 as we saw pre-
viously that there was no difference with the other.
We obtain a nice gain of the GSR value showing that
it is possible to enhance slightly the performance of
the proposed method.
Table 2: Value of the consensus value (GSR) for the 3
matching algorithms with optimized thresholds.
Matching algorithm GSR value
S
1
82.4%
S
2
83,6%
S
3
82.7%
6 CONCLUSION AND
PERSPECTIVES
In this work, we addressed the problem of user clas-
sification in the biometric menagerie. Such a method
could have many applications in biometrics mainly to
adapt the processing in function of the behavior of the
user while using a biometric system. The proposed
approach is based on the definition of a signature re-
lated to the stability and performance associated to a
user. The proposed framework makes it possible to
predict user class in an operational mode by a sim-
ple decision rule. Obtained results on a keystroke dy-
namics dataset composed of biometric data for dif-
ferent passwords permits to measure the consensus of
the prediction. We obtained quantitative results up-
per than 82%. Perspectives of this study concern the
application of the proposed method on other biomet-
ric modalities. We believe that the Doddington zoo is
particularly interesting for behavioral ones. We also
intend to apply the prediction results to enhance/adapt
the performance of biometric systems.
REFERENCES
Barron, U. G., Corkery, G., Barry, B., Butler, F., McDon-
nell, K., and Ward, S. (2008). Assessment of reti-
nal recognition technology as a biometric method for
sheep identification. Computers and electronics in
agriculture, 60(2):156–166.
Blanco-Gonzalo, R., Sanchez-Reillo, R., Liu-Jimenez, J.,
and Sanchez-Redondo, C. (2017). How to assess user
interaction effects in biometric performance. In 2017
IEEE International Conference on Identity, Security
and Behavior Analysis (ISBA), pages 1–6. IEEE.
Doddington, G., Liggett, W., Martin, A., Przybocki, M.,
and Reynolds, D. (1998). Sheep, goats, lambs and
wolves: A statistical analysis of speaker performance
in the nist 1998 speaker recognition evaluation. Tech-
nical report, National Inst of Standards and Technol-
ogy Gaithersburg Md.
Gaines, R. S., Lisowski, W., Press, S. J., and Shapiro, N.
(1980). Authentication by keystroke timing: Some
preliminary results. Technical report, Rand Corp
Santa Monica CA.
Giot, R., El-Abed, M., and Rosenberger, C. (2011).
Keystroke dynamics authentication. In Biometrics,
page chapitre 8. InTech.
Idrus, S. Z. S., Cherrier, E., Rosenberger, C., and Bours, P.
(2013). Soft biometrics for keystroke dynamics. In
International Conference Image Analysis and Recog-
nition, pages 11–18. Springer.
Kirchgasser, S. and Uhl, A. (2016). Biometric menagerie
in time-span separated fingerprint data. In 2016 Inter-
national Conference of the Biometrics Special Interest
Group (BIOSIG), pages 1–7. IEEE.
Lopes Silva, P., Luz, E., Moreira, G., Moraes, L., and
Menotti, D. (2019). Chimerical dataset creation proto-
col based on doddington zoo: A biometric application
with face, eye, and ecg. Sensors, 19(13):2968.
Mhenni, A., Cherrier, E., Rosenberger, C., and Amara, N.
E. B. (2018). Adaptive biometric strategy using dod-
dington zoo classification of user’s keystroke dynam-
ics. In 2018 14th International Wireless Communi-
cations & Mobile Computing Conference (IWCMC),
pages 488–493. IEEE.
Migdal, D. (2019). Contributions to keystroke dynamics for
privacy and security on the Internet. PhD thesis, Nor-
mandie Universit
´
e.
Morales, A., Fierrez, J., and Ortega-Garcia, J. (2014). To-
wards predicting good users for biometric recognition
based on keystroke dynamics. In European Confer-
ence on Computer Vision, pages 711–724. Springer.
Phillips, P. J., Martin, A., Wilson, C. L., and Przybocki, M.
(2000). An introduction evaluating biometric systems.
Computer, 33(2):56–63.
Poh, N. (2010). User-specific score normalization and fu-
sion for biometric person recognition. Advanced Top-
ics in Biometrics, 16:401–418.
Ross, A., Rattani, A., and Tistarelli, M. (2009). Exploiting
the “doddington zoo” effect in biometric fusion. In
2009 IEEE 3rd International Conference on Biomet-
SECRYPT 2021 - 18th International Conference on Security and Cryptography
752