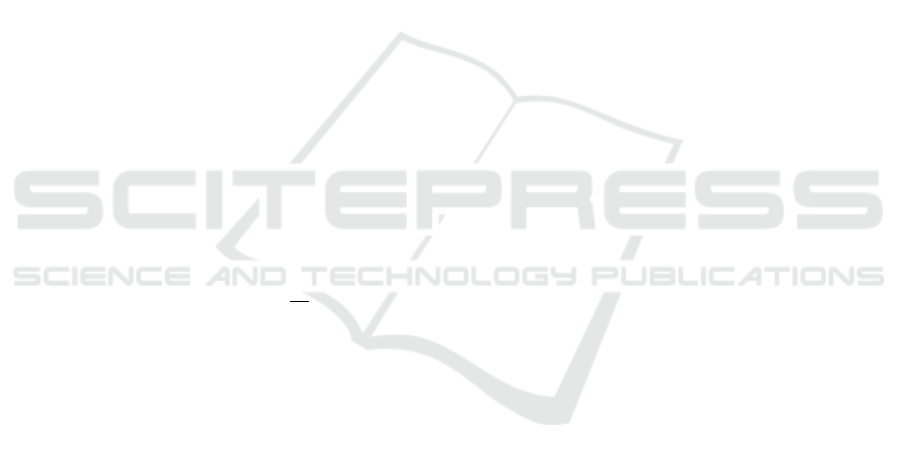
effects of the available vaccines and the clinical evo-
lution of people previously infected and healed.
An analysis of steady state behaviour of the model
is performed, both in the classical uncontrolled case
and, in view of the subsequent study, under the hy-
pothesis of non zero constant control.
All the computations aimed at the determination
of an expression for the reproduction number under
constant control actions, to be used for analysing the
impact of the controls and the reinfection on the virus
spread.
Along with the expected result that social distanc-
ing measurements are effective actions, with an in-
creasing relative increment as the level of restriction
increases, the relative small contribution of the test
campaign is observed.
The new result obtained is related to the effect
of the vaccination on the epidemic containment and,
possibly, extinction. The high impact against the virus
spread is proved, but once the possibility that the im-
munization given by the vaccine has a limited dura-
tion is considered, the real effectiveness of the vac-
cine reduces, and depends on the rate of people vac-
cination with respect to the rate of immunity lost. On
the basis of the computation performed, if the time
of vaccination of the entire population is the same
as the immunity duration, the reproduction number
is halved w.r.t. its original value, despite intuitively
it could seem that, under the same rates, the popula-
tion should be, at steady state, completely vaccinated.
For a disease with R
0
' 3.6 as given for Italy, re-
duction factor must be smaller than
1
3.6
= 0.277: with
the vaccine as the only intervention measurement, a
vaccination rate four times the reinfection one is nec-
essary (Figure 4). An alternative solution could be
represented by keeping social restrictions at the min-
imum level for which an additional reduction factor
is present (Figures 3 and 8). These results suggest to
maintain socially acceptable but non null contact lim-
itations, even if infection trends are satisfactory, and
to speed up the vaccination until levels of immunised
individual is fully compatible with a herd immunity
status.
REFERENCES
Billah, M. A., Miah, M. M., and Khan, M. N. (2020).
Reproductive number of coronavirus: A systematic
review and meta-analysis based on global level evi-
dence. PLoS ONE, 5(11):1–17.
Daley, D. J. and Gani, J. (1999). Epidemic Modelling: An
Introduction. Cambridge Studies in Mathematical Bi-
ology. Cambridge University Press.
Di Giamberardino, P. and Iacoviello, D. (2021). Evaluation
of the effect of different policies in the containment of
epidemic spreads for the COVID-19 case. Biomedical
signal processing and control, 65(102325):1–15.
Di Giamberardino, P., Iacoviello, D., Albano, F., and
Frasca, F. (2020). Age based modelling of SARS-
CoV-2 contagion: The Italian case. 24
th
Int. Conf.
on System Theory, Control and Computing (ICSTCC),
pages 274–279.
Diekmann, O., Heesterbeek, J., and Roberts, M. (2010).
The construction of next-generation matrices for com-
partmental epidemic models. J R Soc Interface,
7(47):873–885.
Diekmann, O., Heesterbeek, J. A. P., and Metz, J. A. J.
(1990). On the definition and the computation of
the basic reproduction ratio R
0
in models for infec-
tious diseases in heterogeneous populations. Journal
of Mathematical Biology, 28:365–382.
Dietz, K. (1993). The estimation of the basic reproduc-
tion number for infectious diseases. Stat Methods Med
Res., 2(1):23–41.
Gumel, A., Iboia, E., Ngonghala, C., and Elbasha, E.
(2021). A primer on using mathematics to understand
covid-19 dynamics: Modeling, analysis and simula-
tions. Infect Dis Model., 6:148–168.
Katul, G. G., Mrad, A., Bonetti, S., Manoli, G., and Paro-
lari, A. J. (2020). Global convergence of covid-19 ba-
sic reproduction number and estimation from early-
time sir dynamics. PLoS ONE, 15(9):1–22.
Kermack, W. O. and McKendrick, A. G. (1927). A contri-
bution to the mathematical theory of epidemics. Proc.
Roy. Soc. Lond. A, 115(772):700–721.
Martcheva, M. (2015). An introduction to mathematical
epidemiology. Text in Appl. Math. 61, Springer.
Perasso, A. (2018). An introduction to the basic reproduc-
tion number in mathematical epidemiology. ESAIM:
ProcS, 62:123–138.
Tang, B., Wang, X., Li, Q., Bragazzi, N. L., Tang, S., Xiao,
Y., and Wu, J. (2020). Estimation of the transmission
risk of the 2019-nCoV and its implication for public
health interventions. J. of Clinical Medicine, 9(2).
van den Driessche, P. (2017). Reproduction numbers of
infectious disease models. Infectious Disease Mod-
elling, 2:288–303.
Zhao, S., Lin, Q., Musa, J. R. S. S., Yang, G., Wang, W.,
Lou, Y., Gao, D., Yang, L., He, D., and Wang, M. H.
(2020). Preliminary estimation of the basic repro-
duction number of novel coronavirus (2019-nCoV) in
China, from 2019 to 2020: A data-driven analysis in
the early phase of the outbreak. Int. J. of Infectious
Diseases, 92:214–217.
Vaccination and Time Limited Immunization for SARS-CoV-2 Infection
619