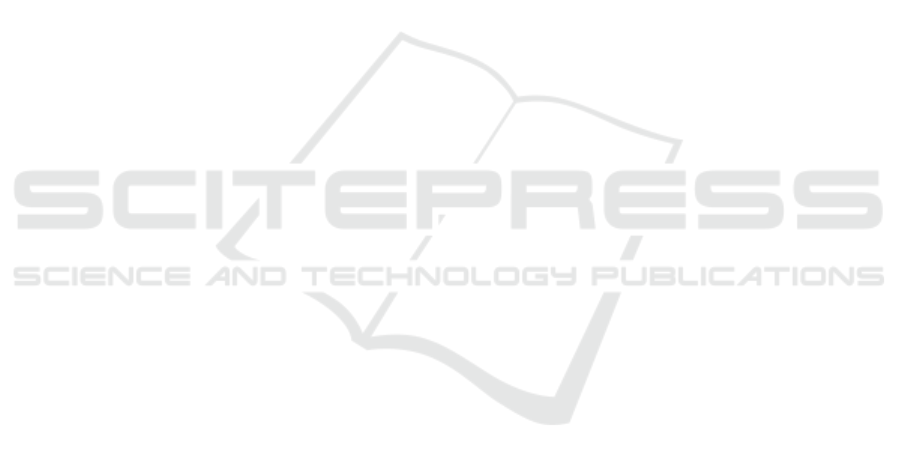
Continuous human activity recognition model based
on deep neural network using imu sensors of smart-
watch. IEEE Sensors Journal, 20(15):8757–8770.
Casale, P., Pujol, O., and Radeva, P. (2011). Human activity
recognition from accelerometer data using a wearable
device. In Iberian Conference on Pattern Recognition
and Image Analysis, pages 289–296. Springer.
Chereshnev, R. and Kert
´
esz-Farkas, A. (2017). Hugadb:
Human gait database for activity recognition from
wearable inertial sensor networks. In International
Conference on Analysis of Images, Social Networks
and Texts, pages 131–141. Springer.
Du, X., Farrahi, K., and Niranjan, M. (2019). Trans-
fer learning across human activities using a cascade
neural network architecture. In Proceedings of the
23rd international symposium on wearable comput-
ers, pages 35–44.
Feng, Z., Mo, L., and Li, M. (2015). A random forest-based
ensemble method for activity recognition. In 2015
37th Annual International Conference of the IEEE En-
gineering in Medicine and Biology Society (EMBC),
pages 5074–5077. IEEE.
Fu, Z., He, X., Wang, E., Huo, J., Huang, J., and Wu,
D. (2021). Personalized human activity recogni-
tion based on integrated wearable sensor and transfer
learning. Sensors, 21(3):885.
Garofalo, G., Argones R
´
ua, E., Preuveneers, D., Joosen, W.,
et al. (2019). A systematic comparison of age and gen-
der prediction on imu sensor-based gait traces. Sen-
sors, 19(13):2945.
Gomaa, W., Elbasiony, R., and Ashry, S. (2017). Adl
classification based on autocorrelation function of
inertial signals. In 2017 16th IEEE International
Conference on Machine Learning and Applications
(ICMLA), pages 833–837.
Jain, A. and Kanhangad, V. (2016). Investigating gen-
der recognition in smartphones using accelerometer
and gyroscope sensor readings. In 2016 International
Conference on Computational Techniques in Infor-
mation and Communication Technologies (ICCTICT),
pages 597–602.
Markitantov, M. (2020). Transfer learning in speaker’s age
and gender recognition. In Karpov, A. and Potapova,
R., editors, Speech and Computer, pages 326–335,
Cham. Springer International Publishing.
Mehrang, S., Pietil
¨
a, J., and Korhonen, I. (2018). An activ-
ity recognition framework deploying the random for-
est classifier and a single optical heart rate monitor-
ing and triaxial accelerometer wrist-band. Sensors,
18(2):613.
Miraldo, D., Watanabe, R., and Duarte, M. (2020). An open
dataset of inertial, magnetic, foot-ground contact, and
electromyographic signals from wearable sensors dur-
ing walking.
Mostafa, A., Barghash, T. O., Assaf, A. A.-S., and Go-
maa, W. (2020). Multi-sensor gait analysis for gender
recognition.
Ngo, T. T., Ahad, M. A. R., Antar, A. D., Ahmed, M., Mura-
matsu, D., Makihara, Y., Yagi, Y., Inoue, S., Hossain,
T., and Hattori, Y. (2019). Ou-isir wearable sensor-
based gait challenge: Age and gender. In Proceedings
of the 12th IAPR International Conference on Biomet-
rics, ICB.
Ngo, T. T., Makihara, Y., Nagahara, H., Mukaigawa, Y.,
and Yagi, Y. (2014). The largest inertial sensor-
based gait database and performance evaluation of
gait-based personal authentication. Pattern Recogni-
tion, 47(1):228–237.
Riaz, Q., V
¨
ogele, A., Kr
¨
uger, B., and Weber, A. (2015). One
small step for a man: Estimation of gender, age and
height from recordings of one step by a single inertial
sensor. Sensors, 15(12):31999–32019.
Rosli, N. A. I. M., Rahman, M. A. A., Balakrishnan, M.,
Komeda, T., Mazlan, S. A., and Zamzuri, H. (2017).
Improved gender recognition during stepping activity
for rehab application using the combinatorial fusion
approach of emg and hrv. Applied Sciences, 7(4):348.
Sun, M., Li, C., and Zha, H. (2017). Inferring private de-
mographics of new users in recommender systems. In
Proceedings of the 20th ACM International Confer-
ence on Modelling, Analysis and Simulation of Wire-
less and Mobile Systems, pages 237–244.
BioDeep: A Deep Learning System for IMU-based Human Biometrics Recognition
629