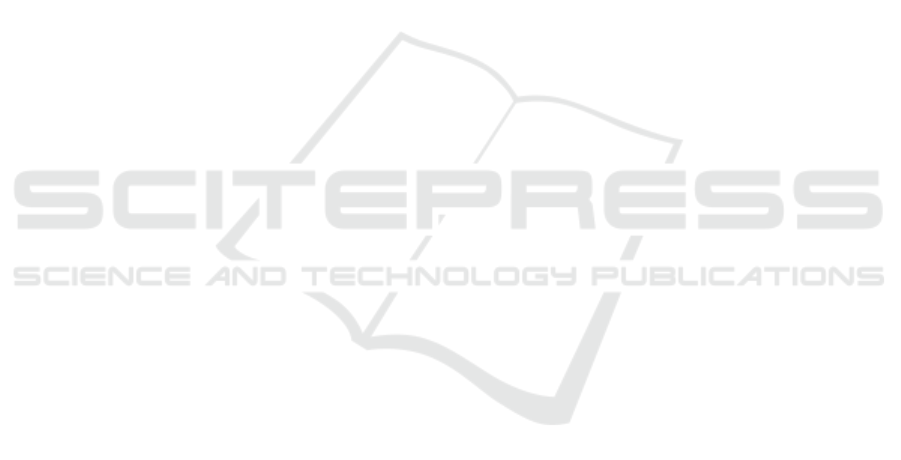
REFERENCES
Abadi, M., Barham, P., Chen, J., Chen, Z., Davis, A., Dean,
J., Devin, M., Ghemawat, S., Irving, G., Isard, M.,
and et al. (2016). Tensorflow: A system for large-
scale machine learning. In 12th USENIX symposium
on operating and systems design and implementation
(OSDI 16), page 265–283.
Anghelescu, M., Tonniges, J. R., Calomeni, E., Shamhart,
P. E., Agarwal, G., Gooch, K. J., and Trask, A. J.
(2015). Vascular mechanics in decellularized aortas
and coronary resistance microvessels in type 2 dia-
betic db/db mice. Annals of biomedical engineering,
43(11):2760–2770.
Blanchard, N., Kinnison, J., RichardWebster, B., Bashivan,
P., and Scheirer, W. J. (2019). A neurobiological eval-
uation metric for neural network model search. 2019
IEEE/CVF Conference on Computer Vision and Pat-
tern Recognition (CVPR).
Deng, J.-Q. and Kwok, Y.-K. (2017). Large vocabulary au-
tomatic chord estimation with an even chance training
scheme. In ISMIR, page 531–536.
Gooch, K. J. and Trask, A. J. (2015). Tissue-specific vascu-
lar remodeling and stiffness associated with metabolic
diseases.
Goodfellow, I., Bengio, Y., and Courville, A. (2016). Deep
Learning. MIT Press.
Husarek, K. E., Katz, P. S., Trask, A. J., Galantowicz, M. L.,
Cismowski, M. J., and Lucchesi, P. A. (2016). The an-
giotensin receptor blocker losartan reduces coronary
arteriole remodeling in type 2 diabetic mice. Vascular
pharmacology, 76:28–36.
Katz, P. S., Trask, A. J., Souza-Smith, F. M., Hutchin-
son, K. R., Galantowicz, M. L., Lord, K. C., Stew-
art, James A., J., Cismowski, M. J., Varner, K. J., and
Lucchesi, P. A. (2011). Coronary arterioles in type
2 diabetic (db/db) mice undergo a distinct pattern of
remodeling associated with decreased vessel stiffness.
Basic research in cardiology, 106(6):1123–1134.
Kingma, D. P. and Ba, J. (2014). Adam: A method for
stochastic optimization. arXiv.
Labazi, H. and Trask, A. J. (2017). Coronary microvascular
disease as an early culprit in the pathophysiology of
diabetes and metabolic syndrome. Pharmacological
research: the official journal of the Italian Pharmaco-
logical Society, 123:114–121.
Lee, S., Park, Y., Dellsperger, K. C., and Zhang, C. (2011a).
Exercise training improves endothelial function via
adiponectin-dependent and independent pathways in
type 2 diabetic mice. American journal of physiology.
Heart and circulatory physiology, 301(2):H306–14.
Lee, S., Park, Y., and Zhang, C. (2011b). Exercise train-
ing prevents coronary endothelial dysfunction in type
2 diabetic mice. American journal of biomedical sci-
ences, 3(4):241–252.
Liu, K., Song, J., Zhang, W., and Yang, X. (2018). Allevi-
ating over-fitting in attribute reduction: An early stop-
ping strategy. In 2018 International Conference on
Wavelet Analysis and Pattern Recognition (ICWAPR),
page 190–195.
Park, Y., Capobianco, S., Gao, X., Falck, J. R., Dellsperger,
K. C., and Zhang, C. (2008). Role of edhf in type 2
diabetes-induced endothelial dysfunction. American
journal of physiology. Heart and circulatory physiol-
ogy, 295(5):H1982–8.
Park, Y., Yang, J., Zhang, H., Chen, X., and Zhang, C.
(2011). Effect of par2 in regulating tnf-α and nad(p)h
oxidase in coronary arterioles in type 2 diabetic mice.
Basic research in cardiology, 106(1):111–123.
Pedregosa, F., Varoquaux, G., and Gramfort, A. (2011).
Scikit-learn: Machine learning in python. of machine
Learning . . . .
Prakash, S. S. and Visakha, K. (2020). Breast cancer malig-
nancy prediction using deep learning neural networks.
In 2020 Second International Conference on Inventive
Research in Computing Applications (ICIRCA), page
88–92.
Prechelt, L. (1998). Automatic early stopping using cross
validation: quantifying the criteria. Neural networks:
the official journal of the International Neural Net-
work Society, 11(4):761–767.
Sunyecz, I. L., McCallinhart, P. E., Patel, K. U., Mc-
Dermott, M. R., and Trask, A. J. (2018). Defining
coronary flow patterns: Comprehensive automation of
transthoracic doppler coronary blood flow. Scientific
reports, 8(1):17268.
Trask, A. J., Delbin, M. A., Katz, P. S., Zanesco, A., and
Lucchesi, P. A. (2012a). Differential coronary resis-
tance microvessel remodeling between type 1 and type
2 diabetic mice: impact of exercise training. Vascular
pharmacology, 57(5-6):187–193.
Trask, A. J., Groban, L., Westwood, B. M., Varagic, J.,
Ganten, D., Gallagher, P. E., Chappell, M. C., and
Ferrario, C. M. (2010). Inhibition of angiotensin-
converting enzyme 2 exacerbates cardiac hypertrophy
and fibrosis in ren-2 hypertensive rats. American jour-
nal of hypertension, 23(6):687–693.
Trask, A. J., Katz, P. S., Kelly, A. P., Galantowicz, M. L.,
Cismowski, M. J., West, T. A., Neeb, Z. P., Berwick,
Z. C., Goodwill, A. G., Alloosh, M., and et al.
(2012b). Dynamic micro- and macrovascular remod-
eling in coronary circulation of obese ossabaw pigs
with metabolic syndrome. Journal of applied physiol-
ogy, 113(7):1128–1140.
Wu, X. and Liu, J. (2009). A new early stopping algorithm
for improving neural network generalization. In 2009
Second International Conference on Intelligent Com-
putation Technology and Automation, volume 1, page
15–18.
Ying, X. (2019). An overview of overfitting and its
solutions. Journal of physics. Conference series,
1168(2):022022.
SIGMAP 2021 - 18th International Conference on Signal Processing and Multimedia Applications
80