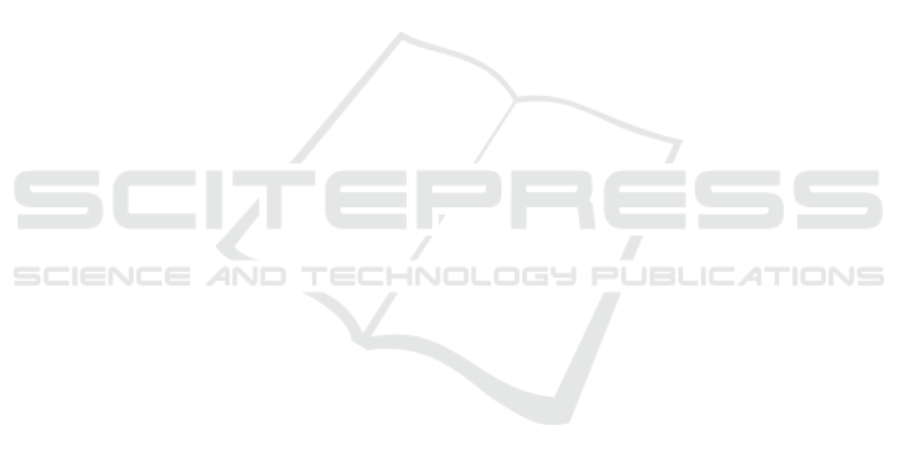
for an accurate radiomic analysis for the prediction,
based on radiological signs, of the clinical outcome
of patients affected by COVID-19 pneumonia.
ACKNOWLEDGEMENTS
This work has been carried out within the
Artificial Intelligence in Medicine (AIM)
project funded by INFN (CSN5, 2019-2021),
https://www.pi.infn.it/aim. We are grateful to the
staff of the Data Center of the INFN Division of
Pisa. We thank the CINECA Italian computing
center for making available part of the computing
resources used in this paper; in particular, Dr. Tom-
maso Boccali (INFN, Pisa) as PI of PRACE Project
Access #2018194658 and a 2021 ISCRA-C grant.
Moreover, we thank the EOS cluster of Department
of Mathematics ”F. Casorati” (Pavia) for computing
resources.
REFERENCES
Adams, H. J., Kwee, T. C., Yakar, D., Hope, M. D., and
Kwee, R. M. (2020). Chest CT Imaging Signature of
Coronavirus Disease 2019 Infection: In Pursuit of the
Scientific Evidence. Chest, 158(5):1885–1895.
An, P., Xu, S., Harmon, S. A., Turkbey, E. B., Sanford,
T. H., Amalou, A., Kassin, M., Varble, N., Blain,
M., Anderson, V., Patella, F., Carrafiello, G., Turkbey,
B. T., and Wood, B. J. (2020). CT Images in COVID-
19.
Carotti, M., Salaffi, F., Sarzi-Puttini, P., Agostini, A.,
Borgheresi, A., Minorati, D., Galli, M., Marotto,
D., and Giovagnoni, A. (2020). Chest CT features
of coronavirus disease 2019 (COVID-19) pneumo-
nia: key points for radiologists. Radiologia Medica,
125(7):636–646.
Desai, S., Baghal, A., Wongsurawat, T., Al-Shukri, S.,
Gates, K., Farmer, P., Rutherford, M., Blake, G.,
Nolan, T., Powell, T., Sexton, K., Bennett, W., and
Prior, F. (2020). Data from Chest Imaging with Clin-
ical and Genomic Correlates Representing a Rural
COVID-19 Positive Population [Data set].
Fang, X., Kruger, U., Homayounieh, F., Chao, H., Zhang, J.,
Digumarthy, S. R., Arru, C. D., Kalra, M. K., and Yan,
P. (2021). Association of AI quantified COVID-19
chest CT and patient outcome. International Journal
of Computer Assisted Radiology and Surgery.
GrandChallenge (2020). COVID-19 Lung CT Le-
sion Segmentation Challenge - 2020, https://covid-
segmentation.grand-challenge.org/COVID-19-20/.
G
¨
ulbay, M.,
¨
Ozbay, B. O., Mendi, B. A. R., Bas¸tu
˘
g,
A., and Bodur, H. (2021). A CT radiomics analy-
sis of COVID-19-related ground-glass opacities and
consolidation: Is it valuable in a differential diag-
nosis with other atypical pneumonias? PloS one,
16(3):e0246582.
Huang, C., Wang, Y., Li, X., Ren, L., Zhao, J., Hu, Y.,
Zhang, L., Fan, G., Xu, J., Gu, X., Cheng, Z., Yu,
T., Xia, J., Wei, Y., Wu, W., Xie, X., Yin, W., Li, H.,
Liu, M., Xiao, Y., Gao, H., Guo, L., Xie, J., Wang,
G., Jiang, R., Gao, Z., Jin, Q., Wang, J., and Cao,
B. (2020). Clinical features of patients infected with
2019 novel coronavirus in Wuhan, China. The Lancet,
395(10223):497–506.
Ishiguro, T., Kobayashi, Y., Uozumi, R., Takata, N.,
Takaku, Y., Kagiyama, N., Kanauchi, T., Shimizu,
Y., and Takayanagi, N. (2019). Viral pneumonia
requiring differentiation from acute and progressive
diffuse interstitial lung diseases. Internal Medicine,
58(24):3509–3519.
Ma, J., Wang, Y., An, X., Ge, C., Yu, Z., Chen, J., Zhu,
Q., Dong, G., He, J., He, Z., Nie, Z., and Yang, X.
(2020). Towards Efficient COVID-19 CT Annotation:
A Benchmark for Lung and Infection Segmentation.
pages 1–7.
Moore, S. M., Maffitt, D. R., Smith, K. E., Kirby, J. S.,
Clark, K. W., Freymann, J. B., Vendt, B. A., Tarbox,
L. R., and Prior, F. W. (2015). De-identification of
medical images with retention of scientific research
value. Radiographics, 35(3):727–735.
Morozov, S. P., Andreychenko, A. E., Pavlov, N. A.,
Vladzymyrskyy, A. V., Ledikhova, N. V., Gom-
bolevskiy, V. A., Blokhin, I. A., Gelezhe, P. B., Gon-
char, A. V., and Chernina, V. (2020). MosMedData:
Chest CT Scans with COVID-19 Related Findings
Dataset. medRxiv, page 2020.05.20.20100362.
MosMed (2020). MosMed dataset website,
https://mosmed.ai/en/.
Ronneberger, O., Fischer, P., and Brox, T. (2015). U-
net: Convolutional networks for biomedical im-
age segmentation. Lecture Notes in Computer Sci-
ence (including subseries Lecture Notes in Artificial
Intelligence and Lecture Notes in Bioinformatics),
9351:234–241.
Scientiae, D. C.-S., Adams, H. J. A., Kwee, T. C., Hope,
M. D., Kwee, R. M., Hja, A., Tc, K., and Yakar, D.
(2020). Systematic Review and Meta- in the Diagno-
sis of Coronavirus. (December):1342–1350.
Standard DICOM (2021). DICOM standard.
World Health Organization (2020). WHO Interim guidance
20 March 2020 - Global Surveillance for COVID-19
disease caused by human infection with novel coron-
avirus (COVID-19). Who, (January):1–4.
Making Data Big for a Deep-learning Analysis: Aggregation of Public COVID-19 Datasets of Lung Computed Tomography Scans
321