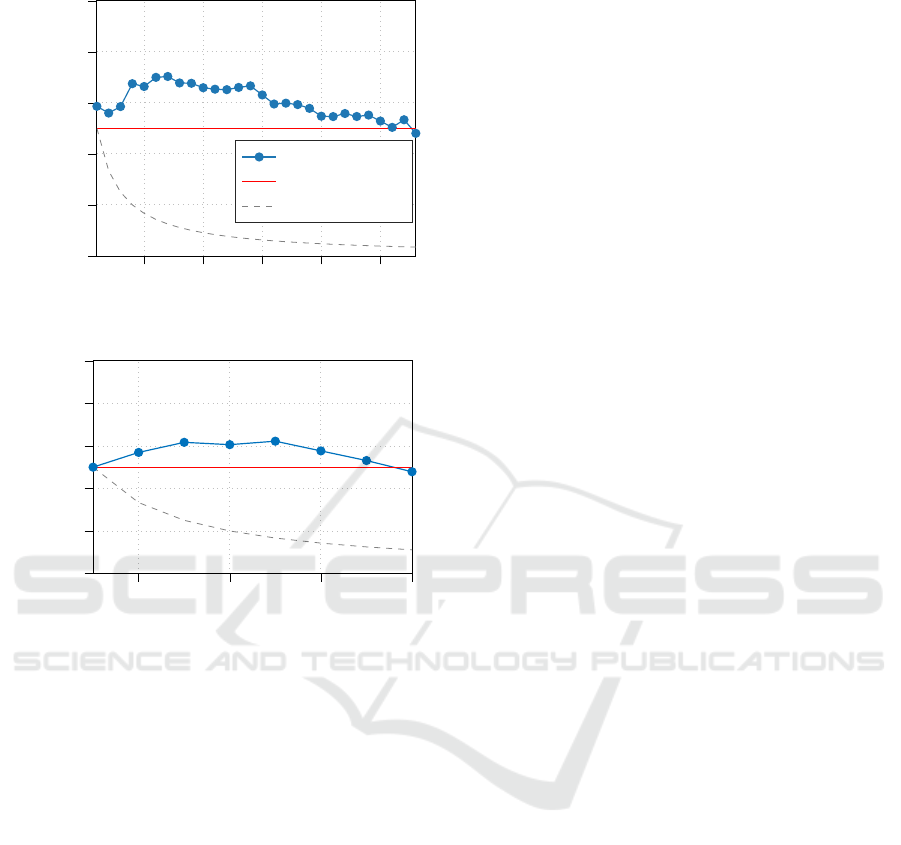
5
10
15
20
25
0
0.2
0.4
0.6
0.8
1
Number of users (N)
P
succ|0.5
Dataset D1
Score method
Naive rejection
Naive guessing
2 4
6
8
0
0.2
0.4
0.6
0.8
1
Number of users (N)
P
succ|0.5
Dataset D2
Figure 4: Success probability of the score method in sce-
nario 2, estimated for varying number N of users and fixed
threshold 10. The probability of inclusion for the target is
fixed at p = 0.5.
ness of anonymization techniques as countermeasures
for linking attacks. Applying straightforwardly con-
cepts like k-anonymity or differential privacy to time
series data will most of the times cause an excessive
loss of information, making the anonymized data un-
usable, so a main aspect to be addressed is the tradeoff
between utility and privacy when protecting PFI data.
8 CONCLUSIONS
Throughout this paper, we investigated the problem of
leveraging personal fitness information to find a target
in a set of N people. Our results show that using just
steps and calories it is possible to identify the user
with higher probability compared to random guess-
ing. We focused on a specific threat model where an
attacker tries to find a target user in an aggregated
dataset. However, identifiable data are prone to a
wider pool of threats that need to be addressed. We
hope that this will raise awareness on the sensitivity
of personal fitness information, and that it will lay the
foundation for future works aimed at protecting it.
ACKNOWLEDGEMENTS
This project has received funding from the Euro-
pean Union’s Horizon 2020 research and innovation
programme under the Marie Skłodowska-Curie grant
agreement No 813162. The content of this paper re-
flects the views only of their author (s). The European
Commission/ Research Executive Agency are not re-
sponsible for any use that may be made of the infor-
mation it contains.
REFERENCES
Al-Makhadmeh, Z. and Tolba, A. (2019). Utilizing iot
wearable medical device for heart disease prediction
using higher order boltzmann model: A classification
approach. Measurement, 147:106815.
Alqhatani, A. and Lipford, H. R. (2019). “there is nothing
that i need to keep secret”: Sharing practices and con-
cerns of wearable fitness data. In Fifteenth Symposium
on Usable Privacy and Security ({SOUPS} 2019).
Christovich, M. M. (2016). Why should we care what fit-
bit shares-a proposed statutroy solution to protect sen-
sative personal fitness information. Hastings Comm.
& Ent. LJ, 38:91.
Hearst, M. A., Dumais, S. T., Osuna, E., Platt, J., and
Scholkopf, B. (1998). Support vector machines. IEEE
Intelligent Systems and their applications, 13(4):18–
28.
Ho, T. K. (1995). Random decision forests. In Proceedings
of 3rd international conference on document analysis
and recognition, volume 1, pages 278–282. IEEE.
Kuncheva, L. I. and Jain, L. C. (1999). Nearest neighbor
classifier: Simultaneous editing and feature selection.
Pattern recognition letters, 20(11-13):1149–1156.
Parzen, E. (1962). On estimation of a probability density
function and mode. The annals of mathematical statis-
tics, 33(3):1065–1076.
Sathyanarayana, A., Joty, S., Fernandez-Luque, L., Ofli, F.,
Srivastava, J., Elmagarmid, A., Arora, T., and Taheri,
S. (2016). Sleep quality prediction from wearable
data using deep learning. JMIR mHealth and uHealth,
4(4):e125.
Thambawita, V., Hicks, S., Borgli, H., Pettersen, S. A., Jo-
hansen, D., Johansen, H., Kupka, T., Stensland, H. K.,
Jha, D., Grønli, T.-M., and et al. (2020). Pmdata: A
sports logging dataset.
Zhu, G., Li, J., Meng, Z., Yu, Y., Li, Y., Tang, X., Dong, Y.,
Sun, G., Zhou, R., Wang, H., et al. (2020). Learning
from large-scale wearable device data for predicting
epidemics trend of covid-19. Discrete Dynamics in
Nature and Society, 2020.
User Identification from Time Series of Fitness Data
811