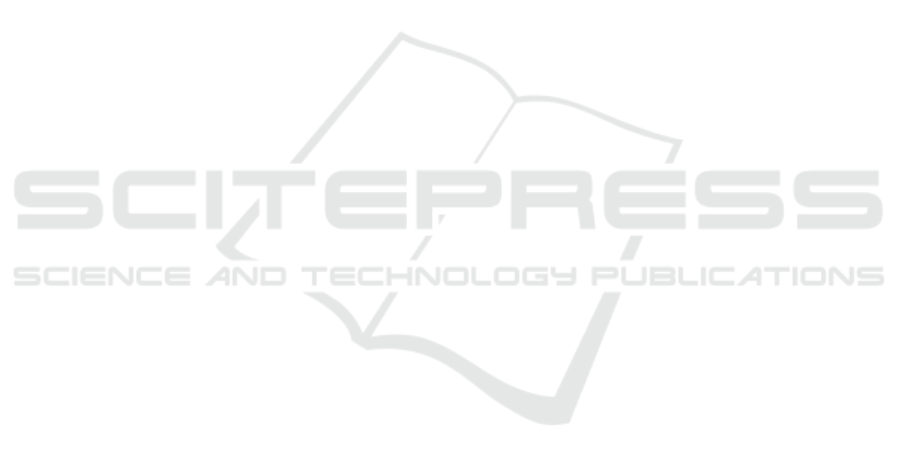
ease. Biomedical Signal Processing and Control,
52:414–419.
Lee, G., Nho, K., Kang, B., Sohn, K.-A., and Kim, D.
(2019). Predicting alzheimer’s disease progression us-
ing multi-modal deep learning approach. Scientific re-
ports, 9(1):1–12.
Li, F., Tran, L., Thung, K.-H., Ji, S., Shen, D., and Li, J.
(2015). A robust deep model for improved classifica-
tion of ad/mci patients. IEEE journal of biomedical
and health informatics, 19(5):1610–1616.
Liu, F., Zhou, L., Shen, C., and Yin, J. (2013). Multiple ker-
nel learning in the primal for multimodal alzheimer’s
disease classification. IEEE journal of biomedical and
health informatics, 18(3):984–990.
Liu, M., Zhang, J., Adeli, E., and Shen, D. (2018).
Joint classification and regression via deep multi-task
multi-channel learning for alzheimer’s disease diag-
nosis. IEEE Transactions on Biomedical Engineering,
66(5):1195–1206.
Lopez-de Ipina, K., Martinez-de Lizarduy, U., Calvo, P. M.,
Mekyska, J., Beitia, B., Barroso, N., Estanga, A.,
Tainta, M., and Ecay-Torres, M. (2018). Advances
on automatic speech analysis for early detection of
alzheimer disease: a non-linear multi-task approach.
Current Alzheimer Research, 15(2):139–148.
Nie, L., Zhang, L., Meng, L., Song, X., Chang, X., and
Li, X. (2016). Modeling disease progression via
multisource multitask learners: A case study with
alzheimer’s disease. IEEE transactions on neural net-
works and learning systems, 28(7):1508–1519.
Nie, L., Zhang, L., Yang, Y., Wang, M., Hong, R., and
Chua, T.-S. (2015). Beyond doctors: Future health
prediction from multimedia and multimodal observa-
tions. In Proceedings of the 23rd ACM international
conference on Multimedia, pages 591–600.
Pan, Y., Liu, M., Lian, C., Zhou, T., Xia, Y., and Shen,
D. (2018). Synthesizing missing pet from mri with
cycle-consistent generative adversarial networks for
alzheimer’s disease diagnosis. In International Con-
ference on Medical Image Computing and Computer-
Assisted Intervention, pages 455–463. Springer.
Pironkov, G., Dupont, S., and Dutoit, T. (2016). Multi-
task learning for speech recognition: an overview. In
ESANN.
Shi, B., Chen, Y., Zhang, P., Smith, C. D., Liu, J., Initiative,
A. D. N., et al. (2017). Nonlinear feature transforma-
tion and deep fusion for alzheimer’s disease staging
analysis. Pattern recognition, 63:487–498.
Spasov, S., Passamonti, L., Duggento, A., Li
`
o, P., Toschi,
N., Initiative, A. D. N., et al. (2019). A parameter-
efficient deep learning approach to predict conversion
from mild cognitive impairment to alzheimer’s dis-
ease. Neuroimage, 189:276–287.
Tabarestani, S., Aghili, M., Eslami, M., Cabrerizo, M., Bar-
reto, A., Rishe, N., Curiel, R. E., Loewenstein, D.,
Duara, R., and Adjouadi, M. (2020). A distributed
multitask multimodal approach for the prediction of
alzheimer’s disease in a longitudinal study. NeuroIm-
age, 206:116317.
Thung, K.-H., Yap, P.-T., and Shen, D. (2017). Multi-stage
diagnosis of alzheimer’s disease with incomplete mul-
timodal data via multi-task deep learning. In Deep
learning in medical image analysis and multimodal
learning for clinical decision support, pages 160–168.
Springer.
Weiner, M. W., Veitch, D. P., Aisen, P. S., Beckett, L. A.,
Cairns, N. J., Green, R. C., Harvey, D., Jack, C. R.,
Jagust, W., Liu, E., et al. (2013). The alzheimer’s dis-
ease neuroimaging initiative: a review of papers pub-
lished since its inception. Alzheimer’s & Dementia,
9(5):e111–e194.
Worsham, J. and Kalita, J. (2020). Multi-task learning for
natural language processing in the 2020s: Where are
we going? Pattern Recognition Letters, 136:120–126.
Zhang, D., Shen, D., Initiative, A. D. N., et al. (2012).
Multi-modal multi-task learning for joint prediction
of multiple regression and classification variables in
alzheimer’s disease. NeuroImage, 59(2):895–907.
Zhang, L., Wang, M., Liu, M., and Zhang, D. (2020). A
survey on deep learning for neuroimaging-based brain
disorder analysis. Frontiers in neuroscience, 14.
Zhang, Y. and Yang, Q. (2018). An overview of multi-task
learning. National Science Review, 5(1):30–43.
DATA 2021 - 10th International Conference on Data Science, Technology and Applications
328