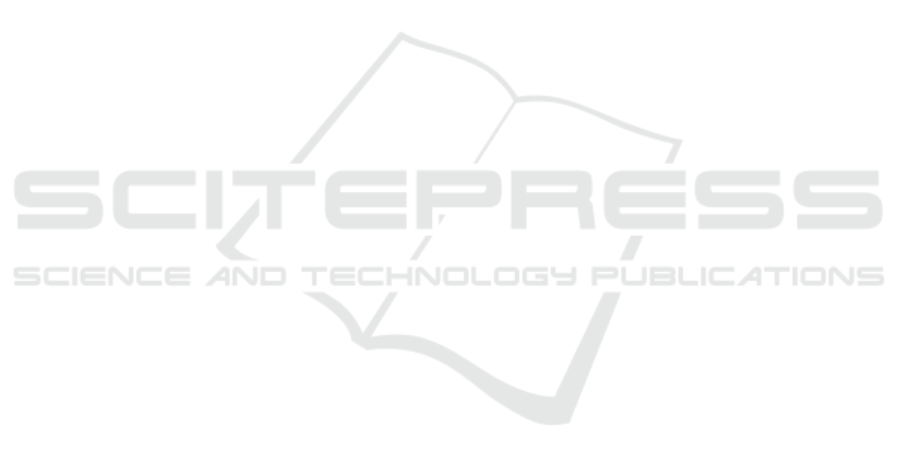
codes or zip codes are further grouped to form a
more prominent territorial region to contain more
risk exposures. This can better reflect the actual loss
pattern and stabilize the risk relativities to minimize
the fluctuation among the calculations using data
from different accident years. A spatial clustering
does this, and a more suitable number of clusters
to be formed to act as new pricing units. Since
postal codes or zip codes are nested in the city or
town, there may be another effect based on different
cities or towns. Those potential effects on insurance
loss may be, in fact, due to some factors associated
with the city or town. For instance, in a city where
commuting buses lack or public transportation is
relatively limited, people tend to drive more to work.
In this work, we propose a method of using Gen-
eralized Linear Mixed Models (GLMM) (Antonio
and Beirlant, 2007) to derive the risk relativity for
different clusters produced by a spatially constrained
clustering(Xie, 2019). GLMM is an extension of
GLM in which the model contains both fixed and
random effects. GLMM can further capture the
impact due to differences among cities or towns
such that the difference in risk relativity associated
with different cities can be better reflected. GLMM
has been successfully used in actuarial science as a
non-life rate-making technique(Jeong et al., 2017),
and a model for credibility(Antonio and Beirlant,
2007). It has also been applied to spatial analysis of
disease spread (Kleinschmidt et al., 2001). We apply
GLMM to model territorial risk in a novelty way and
estimate regional risk relativities. It is considered to
be an extension of the current approach that appeared
in(Xie and Lawniczak, 2018) by further addressing
the impact from other correlated factors on the
territorial risk relativities estimates.
This paper is organized as follows. In Section
2, the data and its basic processing are briefly intro-
duced. In Section 3, the proposed generalized linear
mixed models is discussed. In Section 4, the summary
of the main results are presented. In Section 5, we
conclude our findings and provide further remarks.
2 DATA
In this work, we apply our proposed method to a real
dataset coming from an auto insurance regulator in
Canada. This dataset includes the reported loss in-
formation from all auto insurance companies within
a province for accident years 2009 to 2011. It con-
sists of geographical loss information including postal
codes, cities, reported average loss cost and earned
exposures. The geographical information refers to the
residential places of insured drivers who had reported
the loss, rather than the place where the insured suf-
fered the accident. The reported average loss cost
is the projected ultimate expected loss. The earned
exposures refer to the total number of insured vehi-
cles within a policy year. In this dataset, we first re-
trieved all postal codes that are associated with the
same FSA, where FSA is the first three characters of
postal codes. For each FSA, the postal codes were
further geo-coded using a geo-coder. The obtained
geo-coding contains both latitude and longitude val-
ues that are used to represent the center of a given
FSA. The centroid of FSA is used to identify its loca-
tion.
3 METHODS
This work’s main objective is to estimate each clus-
ter’s risk relativity obtained from a spatial clustering.
At a given level, the relativity of a risk factor is the
risk level relative to the overall averages for all levels
that we consider. In this work, the loss cost at a given
level is divided by the average loss cost across all lev-
els within a risk factor to calculate the risk relativity.
Here we consider the territory risk. The level of terri-
tory risk is at the FSA level, and we try to derive the
relativity associated with each FSA. Data input to the
spatial clustering is three-dimensional, consisting of
normalized lost cost, normalized latitude and normal-
ized longitude. Although the optimal number of clus-
ters is important, it has been fully addressed in (Xie,
2019) using an entropy-based approach. This work is
considered a follow-up study after a spatial clustering
of loss data to determine each FSA’s relativity.
In rate-making, Generalized Linear Models
(GLM) have been widely used because an exponen-
tial family distribution is a better choice for the error
distribution instead of a normal distribution assump-
tion, which is the case in linear models. The main idea
of using GLM for territory risk analysis is to model a
transformation of the expected value of loss cost so
that the predictors have a linear relationship with the
transformed loss cost values. In territory analysis, the
loss cost is defined as the average loss level per vehi-
cle for a defined basic rating unit such as the postal
code. In this work, we extend GLM to the general-
ized linear mixed model (GLMM) (Antonio and Beir-
lant, 2007) to further explain the random effect from a
considered rating variable. Since a city has its own in-
frastructure and public transportation, the underlying
risk of causing accidents is dependent of a city. To
explain the GLMM, let us assume that the loss data
DATA 2021 - 10th International Conference on Data Science, Technology and Applications
330