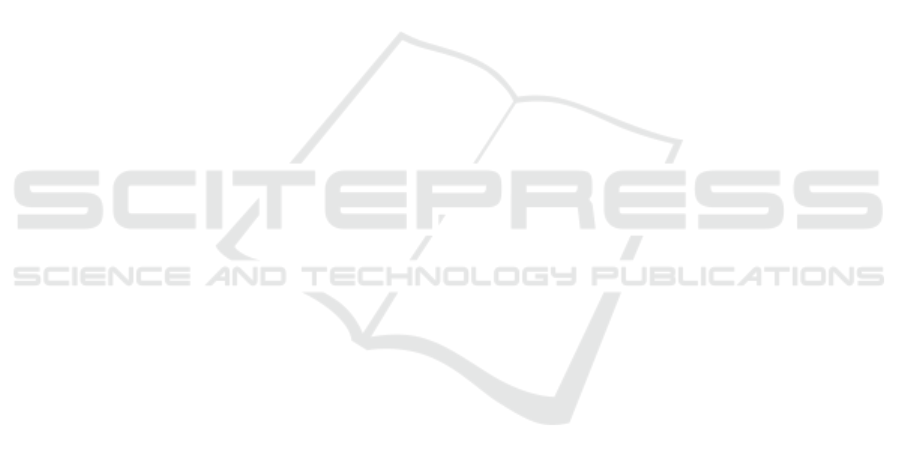
vacy and security risks. The results showed that they
confuse them, which may lead to non-compliance.
REFERENCES
Bellekens, X., Hamilton, A., Seeam, P., Nieradzinska,
K., Franssen, Q., and Seeam, A. (2016). Pervasive
eHealth services a security and privacy risk awareness
survey. In CyberSA. IEEE.
Brooks, S., Brooks, S., Garcia, M., Lefkovitz, N., Light-
man, S., and Nadeau, E. (2017). An introduction to
privacy engineering and risk management in federal
systems. NIST.
Camp, L. J. (2009). Mental models of privacy and security.
IEEE Technology and society magazine.
Commission Nationale de l’Informatique et des Libert
´
es.
How to carry out a PIA.
Commission Nationale de l’Informatique et des Libert
´
es
(2018). Privacy Impact Assessment: Knowledge
Base.
Dashti, S. and Ranise, S. (2019). Tool-assisted risk analysis
for data protection impact assessment. In IFIP SEC.
Springer.
D
´
ıaz Ferreyra, N. E., Kroll, T., A
¨
ımeur, E., Stieglitz, S.,
and Heisel, M. (2020). Preventative nudges: Intro-
ducing risk cues for supporting online self-disclosure
decisions. Information, 11.
Digmayer, C. and Jakobs, E.-M. (2016). Risk perception
of complex technology innovations: Perspectives of
experts and laymen. In ProComm. IEEE.
EDPB. Guidelines on data protection impact assessment
and determining whether processing is “likely to re-
sult in a high risk” for the purposes of regulation
2016/679.
Fischhoff, B., Slovic, P., Lichtenstein, S., Read, S., and
Combs, B. (1978). How safe is safe enough? a
psychometric study of attitudes towards technological
risks and benefits. Policy sciences.
Garg, V. and Camp, J. (2013). Heuristics and biases: im-
plications for security design. IEEE Technology and
Society Magazine.
Gerber, N., Reinheimer, B., and Volkamer, M. (2019). In-
vestigating people’s privacy risk perception. Proceed-
ings on PET, 2019.
Harbach, M., Fahl, S., and Smith, M. (2014). Who’s afraid
of which bad wolf? a survey of it security risk aware-
ness. In IEEE 27th CSF.
Kurasaki, K. S. (2000). Intercoder reliability for validating
conclusions drawn from open-ended interview data.
Field methods.
Lahlou, S., Langheinrich, M., and R
¨
ocker, C. (2005). Pri-
vacy and trust issues with invisible computers. CACM.
LeBlanc, D. and Biddle, R. (2012). Risk perception of
internet-related activities. In PST. IEEE.
Mohallick, I., De Moor, K.,
¨
Ozg
¨
obek,
¨
O., and Gulla, J. A.
(2018). Towards new privacy regulations in Europe:
Users’ privacy perception in recommender systems. In
SpaCCS. Springer.
Oomen, I. and Leenes, R. (2008). Privacy risk perceptions
and privacy protection strategies. In Policies and re-
search in identity management. Springer.
Schneier, B. (2006). Beyond fear: Thinking sensibly about
security in an uncertain world. Springer Science &
Business Media.
Shirazi, F. and Volkamer, M. (2014). What deters jane from
preventing identification and tracking on the web? In
Proceedings of the WPES.
Skirpan, M. W., Yeh, T., and Fiesler, C. (2018). What’s
at stake: Characterizing risk perceptions of emerging
technologies. In Proceedings of CHI.
Smith, H. J., Dinev, T., and Xu, H. (2011). Information pri-
vacy research: an interdisciplinary review. MIS quar-
terly.
Solove, D. J. (2020). The myth of the privacy paradox.
Available at SSRN.
Special Eurobarometer 359 (2011). Attitudes on data pro-
tection and electronic identity in the EU.
Special Eurobarometer 431 (2015). Data protection.
Special Eurobarometer 487a (2019). 87a. general data pro-
tection regulation.
Tahaei, M., Frik, A., and Vaniea, K. (2021). Privacy cham-
pions in software teams: Understanding their motiva-
tions, strategies, and challenges.
Turner, M. M., Skubisz, C., and Rimal, R. N. (2011). The-
ory and practice in risk communication: A review of
the literature and visions for the future. In The Rout-
ledge handbook of health communication. Routledge.
Van Slyke, C., Shim, J., Johnson, R., and Jiang, J. J. (2006).
Concern for information privacy and online consumer
purchasing. JAIS, 7.
Wohlin, C., Runeson, P., H
¨
ost, M., Ohlsson, M. C., Reg-
nell, B., and Wessl
´
en, A. (2012). Experimentation in
software engineering. Springer Science & Business
Media.
Youn, S. (2009). Determinants of online privacy con-
cern and its influence on privacy protection behaviors
among young adolescents. JCA, 43.
Zhang, P. and Jetter, A. (2016). Understanding risk percep-
tion using fuzzy cognitive maps. In PICMET. IEEE.
SECRYPT 2021 - 18th International Conference on Security and Cryptography
832