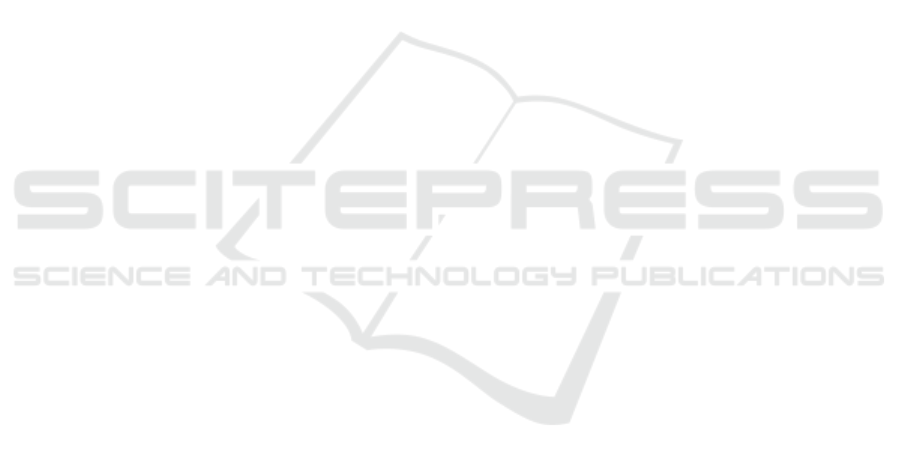
Eddine, C. C., Salem, M. O. B., Khalgui, M., Kahloul, L.,
and Ougouti, N. S. (2020). On the improvement of
r-tncess verification using distributed cloud-based ar-
chitecture.
Ghosh, A., Chakraborty, D., and Law, A. (2018). Artificial
intelligence in internet of things. CAAI Transactions
on Intelligence Technology, 3(4):208–218.
Gu, B., Kong, J., Munir, A., and Kim, Y. G. (2019). A
framework for distributed deep neural network train-
ing with heterogeneous computing platforms. In 2019
IEEE 25th International Conference on Parallel and
Distributed Systems (ICPADS), pages 430–437. IEEE.
Kiran, K. S., Azman, M., Nandu, E., and Prakash, S. S.
(2021a). Real-time detection and prediction of heart
diseases from ecg data using neural networks. In
Proceedings of International Conference on Recent
Trends in Machine Learning, IoT, Smart Cities and
Applications, pages 93–119. Springer.
Kiran, K. S., Azman, M., Nandu, E., and Prakash, S. S.
(2021b). Real-time detection and prediction of heart
diseases from ecg data using neural networks. In
Proceedings of International Conference on Recent
Trends in Machine Learning, IoT, Smart Cities and
Applications, pages 93–119. Springer.
Kolbusz, J., Rozycki, P., Lysenko, O., and Wilamowski,
B. M. (2019). Error back propagation algorithm with
adaptive learning rate. In 2019 International Confer-
ence on Information and Digital Technologies (IDT),
pages 216–222.
Le, D.-N., Parvathy, V. S., Gupta, D., Khanna, A., Ro-
drigues, J. J., and Shankar, K. (2021). Iot enabled
depthwise separable convolution neural network with
deep support vector machine for covid-19 diagnosis
and classification. International Journal of Machine
Learning and Cybernetics, pages 1–14.
Lin, J., Yu, W., Zhang, N., Yang, X., Zhang, H., and Zhao,
W. (2017). A survey on internet of things: Archi-
tecture, enabling technologies, security and privacy,
and applications. IEEE Internet of Things Journal,
4(5):1125–1142.
Liu, X. and Baiocchi, O. (2016). A comparison of the def-
initions for smart sensors, smart objects and things in
iot. In 2016 IEEE 7th Annual Information Technol-
ogy, Electronics and Mobile Communication Confer-
ence (IEMCON), pages 1–4. IEEE.
Ly, H.-B., Nguyen, T.-A., and Pham, B. T. (2021). Estima-
tion of soil cohesion using machine learning method:
A random forest approach. Advances in Civil Engi-
neering, 2021.
Razafimandimby, C., Loscri, V., and Vegni, A. M. (2016).
A neural network and iot based scheme for perfor-
mance assessment in internet of robotic things. In
2016 IEEE first international conference on internet-
of-things design and implementation (IoTDI), pages
241–246. IEEE.
Saleem, T. J. and Chishti, M. A. (2019). Deep learning for
internet of things data analytics. Procedia computer
science, 163:381–390.
Sapna, S., Tamilarasi, A., Kumar, M. P., et al. (2012).
Backpropagation learning algorithm based on leven-
berg marquardt algorithm. Comp Sci Inform Technol
(CS and IT), 2:393–398.
Selvaraj, H., Niewiadomski, H., Buciak, P., Pleban, M., Sa-
piecha, P., Luba, T., and Muthukumar, V. (2002). Im-
plementation of large neural networks using decom-
position.
Teerapittayanon, S., McDanel, B., and Kung, H.-T. (2017a).
Distributed deep neural networks over the cloud, the
edge and end devices. In 2017 IEEE 37th Interna-
tional Conference on Distributed Computing Systems
(ICDCS), pages 328–339. IEEE.
Teerapittayanon, S., McDanel, B., and Kung, H.-T. (2017b).
Distributed deep neural networks over the cloud, the
edge and end devices. In 2017 IEEE 37th Interna-
tional Conference on Distributed Computing Systems
(ICDCS), pages 328–339. IEEE.
Yoon, J. (2019). Using a deep-learning approach for smart
iot network packet analysis. In 2019 IEEE European
Symposium on Security and Privacy Workshops (Eu-
roS PW), pages 291–299.
Yoon, J. (2019). Using a deep-learning approach for smart
iot network packet analysis. In 2019 IEEE European
Symposium on Security and Privacy Workshops (Eu-
roS&PW), pages 291–299. IEEE.
Mixed Software/Hardware based Neural Network Learning Acceleration
425