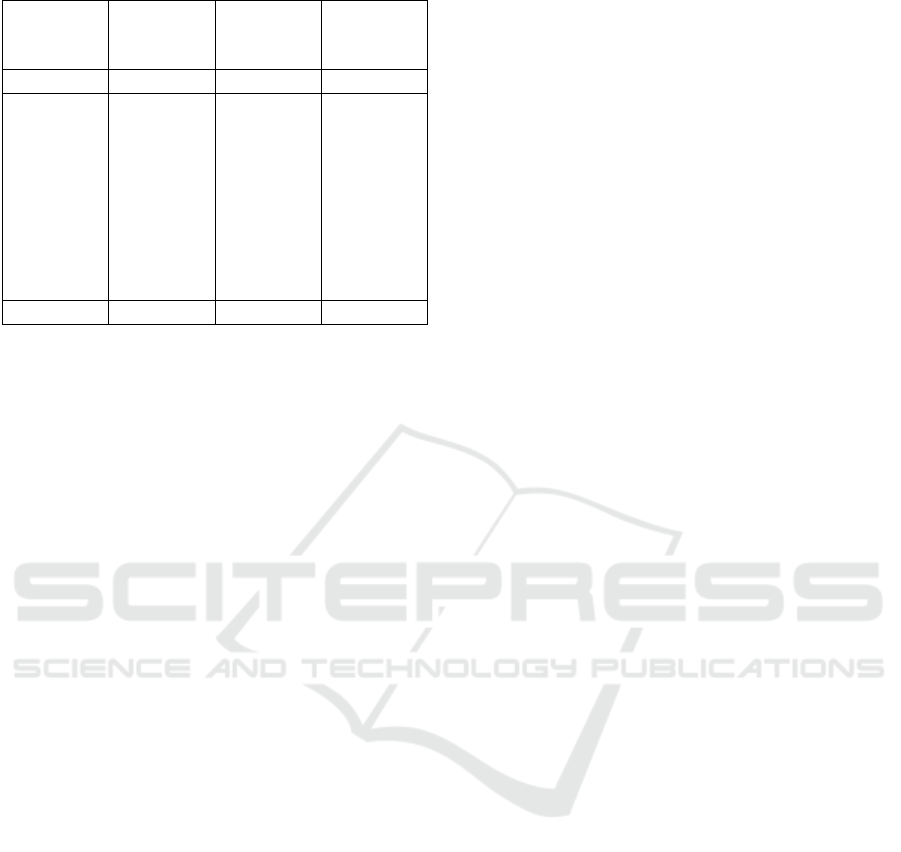
Table 3: Experiment results of Model1.
Classifier Accuracy F1 Score
(macro-
avg)
PCC
RF 0.790 0.47 061
KNN
(neighbor
= 5)
0.50 0.15 0.35
KNN
(neighbor
= 10)
0.612 0.20 0.35
KNN
(neighbor
= 15)
0.612 0.23 0.35
DT 0.779 0.56 0.653
sifier to predict QoE in the context of adaptive video
streaming services. For future work we aim to de-
velop QoE management approach of video services in
SDN/MEC environment where we can implement our
QoE prediction model as in-network solution. our on-
going work will focus on QoE management and con-
trol approach for video streaming services delivered
over the emergent network technologies.
REFERENCES
Le Callet, P., M
¨
oller, S., & Perkis, A. (2012). Qualinet
white paper on definitions of quality of experience.
European network on quality of experience in multi-
media systems and services (COST Action IC 1003),
3(2012).
Reiter, U., Brunnstr
¨
om, K., De Moor, K., Larabi, M. C.,
Pereira, M., Pinheiro, A., ... & Zgank, A. (2014). Fac-
tors influencing quality of experience. In Quality of
experience (pp. 55-72). Springer, Cham.
Barakovi
´
c Husi
´
c, J., Barakovi
´
c, S., Cero, E., Slamnik, N.,
O
´
cuz, M., Dedovi
´
c, A., & Zup
ˇ
ci
´
c, O. (2020). Quality
of experience for unified communications: A survey.
International Journal of Network Management, 30(3),
e2083.
Juluri, P., Tamarapalli, V., & Medhi, D. (2015). Measure-
ment of quality of experience of video-on-demand ser-
vices: A survey. IEEE Communications Surveys &
Tutorials, 18(1), 401-418.
Wamser, F., Seufert, M., Casas, P., Irmer, R., Tran-Gia, P.,
& Schatz, R. (2015, June). YoMoApp: A tool for an-
alyzing QoE of YouTube HTTP adaptive streaming in
mobile networks. In 2015 European Conference on
Networks and Communications (EuCNC) (pp. 239-
243). IEEE.
Robitza, W., Dethof, A. M., G
¨
oring, S., Raake, A., Beyer,
A., & Polzehl, T. (2020, May). Are You Still Watch-
ing? Streaming Video Quality and Engagement As-
sessment in the Crowd. In 2020 Twelfth Interna-
tional Conference on Quality of Multimedia Experi-
ence (QoMEX) (pp. 1-6). IEEE.
Casas, P., Seufert, M., & Schatz, R. (2013). YOUQMON: A
system for on-line monitoring of YouTube QoE in op-
erational 3G networks. ACM SIGMETRICS Perfor-
mance Evaluation Review, 41(2), 44-46.
Dimopoulos, G., Leontiadis, I., Barlet-Ros, P., & Papagian-
naki, K. (2016, November). Measuring video QoE
from encrypted traffic. In Proceedings of the 2016 In-
ternet Measurement Conference (pp. 513-526).
Moldovan, C., & Metzger, F. (2016, September). Bridg-
ing the gap between qoe and user engagement in http
video streaming. In 2016 28th International Teletraffic
Congress (ITC 28) (Vol. 1, pp. 103-111). IEEE.
Shafiq, M. Z., Erman, J., Ji, L., Liu, A. X., Pang, J., &
Wang, J. (2014). Understanding the impact of net-
work dynamics on mobile video user engagement.
ACM SIGMETRICS Performance Evaluation Re-
view, 42(1), 367-379.
Orsolic, I., Pevec, D., Suznjevic, M., & Skorin-Kapov,
L. (2017). A machine learning approach to classify-
ing YouTube QoE based on encrypted network traf-
fic. Multimedia tools and applications, 76(21), 22267-
22301.
ark, M., Naaman, M., & Berger, J. (2016, March). A data-
driven study of view duration on youtube. In Proceed-
ings of the International AAAI Conference on Web
and Social Media (Vol. 10, No. 1).
Wu, S., Rizoiu, M. A., & Xie, L. (2018, June). Beyond
views: Measuring and predicting engagement in on-
line videos. In Proceedings of the International AAAI
Conference on Web and Social Media (Vol. 12, No.
1).
Bartolec, I., Orsolic, I., & Skorin-Kapov, L. (2019, June).
In-network YouTube performance estimation in light
of end user playback-related interactions. In 2019
Eleventh International Conference on Quality of Mul-
timedia Experience (QoMEX) (pp. 1-3). IEEE.
Seufert, M., Casas, P., Wehner, N., Gang, L., & Li, K.
(2019, February). Stream-based machine learning for
real-time QoE analysis of encrypted video stream-
ing traffic. In 2019 22nd Conference on innovation in
clouds, internet and networks and workshops (ICIN)
(pp. 76-81). IEEE.
Letaifa, A. B. (2017, June). Adaptive QoE monitoring ar-
chitecture in SDN networks: Video streaming services
case. In 2017 13th International Wireless Communi-
cations and Mobile Computing Conference (IWCMC)
(pp. 1383-1388). IEEE.
Abar, T., Letaifa, A. B., & El Asmi, S. (2017, June).
Machine learning based QoE prediction in SDN net-
works. In 2017 13th International Wireless Communi-
cations and Mobile Computing Conference (IWCMC)
(pp. 1395-1400). IEEE.
Laiche, F., Letaifa, A. B., & Aguili, T. (2020, Septem-
ber). QoE Influence Factors (IFs) classification Sur-
vey focusing on User Behavior/Engagement metrics.
In 2020 IEEE 29th International Conference on En-
abling Technologies: Infrastructure for Collaborative
Enterprises (WETICE) (pp. 143-146). IEEE.
Inclusion of User Behavior and Social Context Information in ML-based QoE Prediction
603