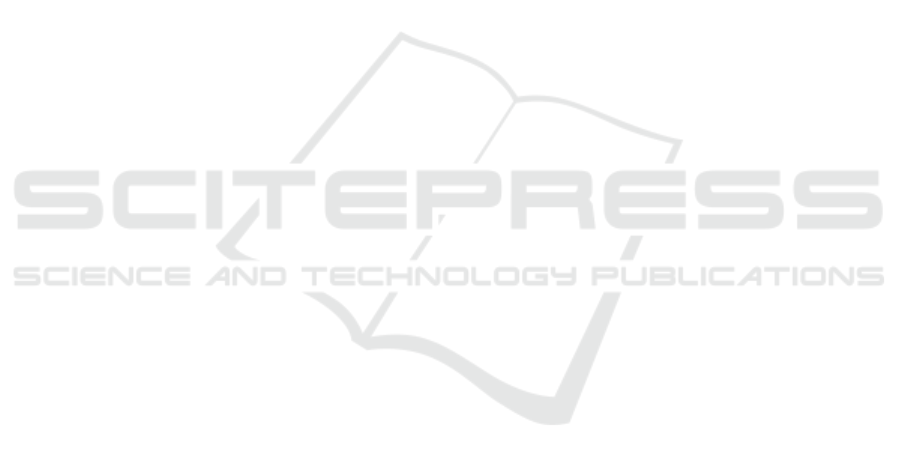
nine datasets were summarized, in different groups of
features. Each dataset is accompanied by a data lo-
cation link. All the mentioned datasets have ground
truth data, except for one dataset, which provides data
from a new colour version of the DVS camera. An-
other, currently unique, dataset is aimed at agricul-
tural environments, where data are recorded in such
settings as a forest, a meadow, a cattle farm, etc.
Choosing an appropriate dataset is an essential
task for successful evaluation and development of
new methods as well as for their quantitative and qual-
itative analysis. The type of environment and the
type of camera motion used (fast, slow, rotational,
and translational) within n-DOF are two major fac-
tors. While there is a sparse availability of event-
based visual navigation datasets, there are no datasets
that provide data for event-based feature detection and
tracking. This direction of event-based visual naviga-
tion is based on the classical approach to how motion
is estimated from frame-based data. Based on all of
the above, the design of new datasets is highly neces-
sary since it will lead to the development and better
availability of new methods.
ACKNOWLEDGEMENTS
A.Zujevs is supported by the European Regional
Development Fund within the Activity 1.1.1.2
“Post-doctoral Research Aid” of the Specific Aid
Objective 1.1.1 (No.1.1.1.2/VIAA/2/18/334), while
A.Nikitenko is supported by the Latvian Council of
Science (lzp-2018/1-0482).
REFERENCES
Alzugaray, I. and Chli, M. (2018). Asynchronous Cor-
ner Detection and Tracking for Event Cameras in
Real Time. IEEE Robotics and Automation Letters,
3(4):3177–3184.
Barranco, F., Fermuller, C., Aloimonos, Y., and Delbruck,
T. (2016). A Dataset for Visual Navigation with
Neuromorphic Methods. Frontiers in Neuroscience,
10(FEB):1–9.
Benosman, R., Clercq, C., Lagorce, X., Sio-Hoi Ieng, and
Bartolozzi, C. (2014). Event-Based Visual Flow.
IEEE Transactions on Neural Networks and Learning
Systems, 25(2):407–417.
Binas, J., Neil, D., Liu, S.-C., and Delbruck, T. (2017).
DDD17: End-To-End DAVIS Driving Dataset. pages
1–9.
Brandli, C., Berner, R., Minhao Yang, Shih-Chii Liu, and
Delbruck, T. (2014). A 240x180 130 dB 3 us Latency
Global Shutter Spatiotemporal Vision Sensor. IEEE
Journal of Solid-State Circuits, 49(10):2333–2341.
Brandli, C., Strubel, J., Keller, S., Scaramuzza, D., and
Delbruck, T. (2016). ELiSeD — An event-based line
segment detector. In 2016 Second International Con-
ference on Event-based Control, Communication, and
Signal Processing (EBCCSP), pages 1–7. IEEE.
Bryner, S., Gallego, G., Rebecq, H., and Scaramuzza, D.
(2019). Event-based, Direct Camera Tracking from
a Photometric 3D Map using Nonlinear Optimization.
In 2019 International Conference on Robotics and Au-
tomation (ICRA), volume 2019-May, pages 325–331.
IEEE.
Conradt, J., Berner, R., Cook, M., and Delbruck, T. (2009).
An embedded AER dynamic vision sensor for low-
latency pole balancing. In 2009 IEEE 12th Inter-
national Conference on Computer Vision Workshops,
ICCV Workshops, pages 780–785. IEEE.
Gallego, G., Delbruck, T., Orchard, G. M., Bartolozzi,
C., Taba, B., Censi, A., Leutenegger, S., Davison,
A., Conradt, J., Daniilidis, K., and Scaramuzza, D.
(2020). Event-based Vision: A Survey. IEEE Trans-
actions on Pattern Analysis and Machine Intelligence,
pages 1–1.
Gallego, G., Lund, J. E. A., Mueggler, E., Rebecq, H.,
Delbr
¨
uck, T., and Scaramuzza, D. (2016). Event-
based, 6-dof camera tracking for high-speed applica-
tions. ArXiv, abs/1607.03468.
Gehrig, M., Aarents, W., Gehrig, D., and Scaramuzza, D.
(2021). Dsec: A stereo event camera dataset for driv-
ing scenarios. IEEE Robotics and Automation Letters,
6(3):4947–4954.
Hess, W., Kohler, D., Rapp, H., and Andor, D. (2016). Real-
time loop closure in 2D LIDAR SLAM. In 2016 IEEE
International Conference on Robotics and Automation
(ICRA), volume 2016-June, pages 1271–1278. IEEE.
Hu, Y., Binas, J., Neil, D., Liu, S.-C., and Delbruck, T.
(2020). DDD20 End-to-End Event Camera Driving
Dataset: Fusing Frames and Events with Deep Learn-
ing for Improved Steering Prediction. arXiv.
Katz, M. L., Nikolic, K., and Delbruck, T. (2012). Live
demonstration: Behavioural emulation of event-based
vision sensors. In 2012 IEEE International Sympo-
sium on Circuits and Systems, pages 736–740. IEEE.
Kim, H., Leutenegger, S., and Davison, A. J. (2016). Real-
Time 3D Reconstruction and 6-DoF Tracking with an
Event Camera. In Proceedings of the European Con-
ference on Computer Vision (ECCV), pages 349–364.
Springer, Cham, eccv 2016. edition.
Liu, M. and Delbruck, T. (2018). Adaptive Time-Slice
Block-Matching Optical Flow Algorithm for Dynamic
Vision Sensors. In British Machine Vision Conference
2018.
Mahowald, M. (1992). VLSI Analogs of Neuronal Visual
Processing: A Synthesis of Form and Function. PhD
thesis, California Institute of Technology Pasadena,
California.
Mueggler, E. (2017). Event-based Vision for High-Speed
Robotics. PhD thesis, University of Zurich.
Mueggler, E., Bartolozzi, C., and Scaramuzza, D. (2017a).
Fast Event-based Corner Detection. In Procedings
ICINCO 2021 - 18th International Conference on Informatics in Control, Automation and Robotics
512