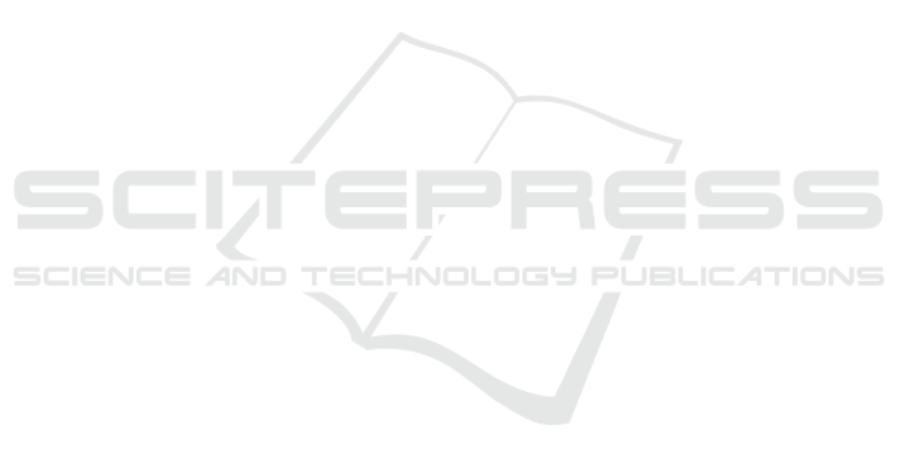
mation extraction to improve production control and
design decisions. In Industrial Conference on Data
Mining, pages 286–300. Springer.
Escobar, C. A. and Morales-Menendez, R. (2018). Machine
learning techniques for quality control in high confor-
mance manufacturing environment. Advances in Me-
chanical Engineering, 10(2):1687814018755519.
Farahani, S., Brown, N., Loftis, J., Krick, C., Pichl, F., Vac-
ulik, R., and Pilla, S. (2019). Evaluation of in-mold
sensors and machine data towards enhancing prod-
uct quality and process monitoring via industry 4.0.
The International Journal of Advanced Manufactur-
ing Technology, 105(1):1371–1389.
Herrero, S. G., Salda
˜
na, M. A. M., del Campo, M. A. M.,
and Ritzel, D. O. (2002). From the traditional concept
of safety management to safety integrated with qual-
ity.
Hollnagel, E., Woods, D. D., and Leveson, N. (2006). Re-
silience engineering: Concepts and precepts. Ashgate
Publishing, Ltd.
Jain, P., Mentzer, R., and Mannan, M. S. (2018). Re-
silience metrics for improved process-risk decision
making: Survey, analysis and application. Safety sci-
ence, 108:13–28.
Kaar, C., Frysak, J., Stary, C., Kannengiesser, U., and
M
¨
uller, H. (2018). Resilient ontology support facil-
itating multi-perspective process integration in indus-
try 4.0. In Proceedings of the 10th International Con-
ference on Subject-Oriented Business Process Man-
agement, pages 1–10.
Kusiak, A. (2019). Fundamentals of smart manufacturing:
A multi-thread perspective. Annual Reviews in Con-
trol, 47:214–220.
Lade, P., Ghosh, R., and Srinivasan, S. (2017a). Manufac-
turing analytics and industrial internet of things. IEEE
Intelligent Systems, 32(3):74–79.
Lade, P., Ghosh, R., and Srinivasan, S. (2017b). Manufac-
turing analytics and industrial internet of things. IEEE
Intelligent Systems, 32(3):74–79.
Lee, E. A. (2015). The past, present and future of cyber-
physical systems: A focus on models. Sensors,
15(3):4837–4869.
Marrella, A., Mecella, M., Pernici, B., and Plebani, P.
(2019). A design-time data-centric maturity model for
assessing resilience in multi-party business processes.
Information Systems, 86:62–78.
Mohanty, S., Gupta, K. K., and Raju, K. S. (2015). Vibra-
tion feature extraction and analysis of industrial ball
mill using mems accelerometer sensor and synchro-
nized data analysis technique. Procedia Computer
Science, 58:217–224.
M
¨
uller, G., Koslowski, T. G., and Accorsi, R. (2013).
Resilience-a new research field in business informa-
tion systems? In International Conference on Busi-
ness Information Systems, pages 3–14. Springer.
Pereira, E., Pinto, R., Reis, J., and Gonc¸alves, G. (2019).
Mqtt-rd: A mqtt based resource discovery for machine
to machine communication. In IoTBDS, pages 115–
124.
Reis, M. S. and Gins, G. (2017). Industrial process moni-
toring in the big data/industry 4.0 era: From detection,
to diagnosis, to prognosis. Processes, 5(3):35.
Rosemann, M. and Recker, J. (2006). Context-aware pro-
cess design: Exploring the extrinsic drivers for pro-
cess flexibility. In Proceedings of the Workshops and
Doctoral Consortium, pages 149–158. Namur Univer-
sity Press.
Schmitt, R., Permin, E., Kerkhoff, J., Plutz, M., and
B
¨
ockmann, M. G. (2017). Enhancing resiliency
in production facilities through cyber physical sys-
tems. In Industrial internet of things, pages 287–313.
Springer.
Shahbazi, Z. and Byun, Y.-C. (2021). Integration of
blockchain, iot and machine learning for multistage
quality control and enhancing security in smart manu-
facturing. Sensors, 21(4):1467.
Sheffi, Y. (2007). The resilient enterprise: overcoming vul-
nerability for competitive advantage. Zone Books.
Tellaeche, A. and Arana, R. (2013). Machine learning al-
gorithms for quality control in plastic molding indus-
try. In 2013 IEEE 18th Conference on Emerging Tech-
nologies & Factory Automation (ETFA), pages 1–4.
IEEE.
Tsang, Y. P., Choy, K. L., Wu, C.-H., Ho, G. T., Lam,
C. H., and Koo, P. (2018). An internet of things (iot)-
based risk monitoring system for managing cold sup-
ply chain risks. Industrial Management & Data Sys-
tems.
Wang, T., Chen, Y., Qiao, M., and Snoussi, H. (2018). A fast
and robust convolutional neural network-based defect
detection model in product quality control. The In-
ternational Journal of Advanced Manufacturing Tech-
nology, 94(9):3465–3471.
Zamiri, M., Marcelino-Jesus, E., Calado, J., Sarraipa, J.,
and Goncalves, R. J. (2019). Knowledge management
in research collaboration networks. In 2019 Interna-
tional Conference on Industrial Engineering and Sys-
tems Management (IESM), pages 1–6. IEEE.
IoT, Risk and Resilience based Framework for Quality Control: Application for Production in Plastic Machining
611