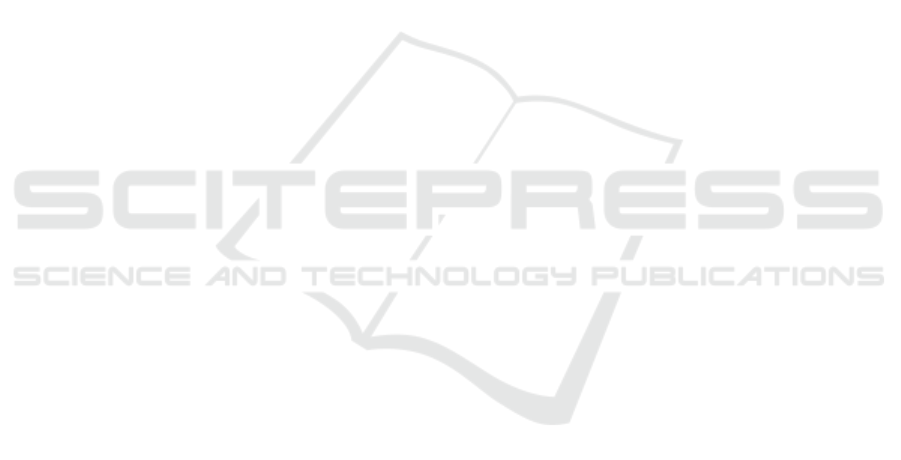
17R/FSE) and the Office of Naval Research Global
project ONRG-NICOP-N62909-19-1-2027.
REFERENCES
Alonso, I., Riazuelo, L., Montesano, L., and Murillo, A. C.
(2020). 3d-mininet: Learning a 2d representation
from point clouds for fast and efficient 3d lidar se-
mantic segmentation. IEEE Robotics and Automation
Society, RAL.
Behley, J., Garbade, M., Milioto, A., Quenzel, J., Behnke,
S., Stachniss, C., and Gall, J. (2019). SemanticKITTI:
A dataset for semantic scene understanding of lidar
sequences. In Proceedings of the IEEE International
Conference on Computer Vision.
Chen, M., Xue, H., and Cai, D. (2019). Domain adaptation
for semantic segmentation with maximum squares
loss. In Proceedings of the IEEE International Con-
ference on Computer Vision, pages 2090–2099.
Ganin, Y., Ustinova, E., Ajakan, H., Germain, P.,
Larochelle, H., Laviolette, F., Marchand, M., and
Lempitsky, V. (2016). Domain-adversarial training of
neural networks. The journal of machine learning re-
search, 17(1):2096–2030.
Hoffman, J., Tzeng, E., Park, T., Zhu, J.-Y., Isola, P.,
Saenko, K., Efros, A., and Darrell, T. (2018). Cycada:
Cycle-consistent adversarial domain adaptation. In
International conference on machine learning, pages
1989–1998.
Jaritz, M., Vu, T.-H., Charette, R. d., Wirbel, E., and P
´
erez,
P. (2020). xmuda: Cross-modal unsupervised domain
adaptation for 3d semantic segmentation. In Proceed-
ings of the IEEE/CVF Conference on Computer Vision
and Pattern Recognition, pages 12605–12614.
Li, Y., Yuan, L., and Vasconcelos, N. (2019). Bidirectional
learning for domain adaptation of semantic segmenta-
tion. In Proceedings of the IEEE Conference on Com-
puter Vision and Pattern Recognition, pages 6936–
6945.
Mei, J., Gao, B., Xu, D., Yao, W., Zhao, X., and Zhao,
H. (2019). Semantic segmentation of 3d lidar data in
dynamic scene using semi-supervised learning. IEEE
Transactions on Intelligent Transportation Systems,
21(6):2496–2509.
Milioto, A., Vizzo, I., Behley, J., and Stachniss, C. (2019).
Rangenet++: Fast and accurate lidar semantic seg-
mentation. In Proceedings of the IEEE/RSJ Interna-
tional Conference on Intelligent Robots and Systems
(IROS).
Pan, Y., Gao, B., Mei, J., Geng, S., Li, C., and Zhao, H.
(2020). Semanticposs: A point cloud dataset with
large quantity of dynamic instances. arXiv preprint
arXiv:2002.09147.
Qi, C. R., Su, H., Mo, K., and Guibas, L. J. (2017a). Point-
net: Deep learning on point sets for 3d classification
and segmentation. In Proceedings of the IEEE Con-
ference on Computer Vision and Pattern Recognition,
pages 652–660.
Qi, C. R., Yi, L., Su, H., and Guibas, L. J. (2017b). Point-
net++: Deep hierarchical feature learning on point sets
in a metric space. In Advances in neural information
processing systems, pages 5099–5108.
Rosu, R. A., Sch
¨
utt, P., Quenzel, J., and Behnke, S. (2019).
Latticenet: Fast point cloud segmentation using per-
mutohedral lattices. arXiv preprint arXiv:1912.05905.
Roynard, X., Deschaud, J.-E., and Goulette, F. (2018).
Paris-lille-3d: A large and high-quality ground-truth
urban point cloud dataset for automatic segmenta-
tion and classification. The International Journal of
Robotics Research, 37(6):545–557.
Sun, B., Feng, J., and Saenko, K. (2017). Correlation align-
ment for unsupervised domain adaptation. In Domain
Adaptation in Computer Vision Applications, pages
153–171. Springer.
Tzeng, E., Hoffman, J., Saenko, K., and Darrell, T. (2017).
Adversarial discriminative domain adaptation. In Pro-
ceedings of the IEEE conference on computer vision
and pattern recognition, pages 7167–7176.
Vu, T.-H., Jain, H., Bucher, M., Cord, M., and P
´
erez, P.
(2019). Advent: Adversarial entropy minimization for
domain adaptation in semantic segmentation. In Pro-
ceedings of the IEEE conference on computer vision
and pattern recognition, pages 2517–2526.
Wang, M. and Deng, W. (2018). Deep visual domain adap-
tation: A survey. Neurocomputing, 312:135–153.
Wu, B., Wan, A., Yue, X., and Keutzer, K. (2018). Squeeze-
seg: Convolutional neural nets with recurrent crf for
real-time road-object segmentation from 3d lidar point
cloud. In 2018 IEEE International Conference on
Robotics and Automation (ICRA), pages 1887–1893.
IEEE.
Wu, B., Zhou, X., Zhao, S., Yue, X., and Keutzer, K.
(2019). Squeezesegv2: Improved model structure
and unsupervised domain adaptation for road-object
segmentation from a lidar point cloud. In 2019 In-
ternational Conference on Robotics and Automation
(ICRA), pages 4376–4382. IEEE.
Xie, Y., Tian, J., and Zhu, X. X. (2019). A review of
point cloud semantic segmentation. arXiv preprint
arXiv:1908.08854.
Yang, Y. and Soatto, S. (2020). Fda: Fourier domain adap-
tation for semantic segmentation. In Proceedings of
the IEEE/CVF Conference on Computer Vision and
Pattern Recognition, pages 4085–4095.
Zhang, Y., Zhou, Z., David, P., Yue, X., Xi, Z., Gong, B.,
and Foroosh, H. (2020). Polarnet: An improved grid
representation for online lidar point clouds semantic
segmentation. In Proceedings of the IEEE/CVF Con-
ference on Computer Vision and Pattern Recognition,
pages 9601–9610.
Zhou, Y. and Tuzel, O. (2018). Voxelnet: End-to-end learn-
ing for point cloud based 3d object detection. In Pro-
ceedings of the IEEE Conference on Computer Vision
and Pattern Recognition, pages 4490–4499.
Zou, Y., Yu, Z., Liu, X., Kumar, B., and Wang, J. (2019).
Confidence regularized self-training. In Proceedings
of the IEEE International Conference on Computer
Vision, pages 5982–5991.
Domain Adaptation in LiDAR Semantic Segmentation by Aligning Class Distributions
337