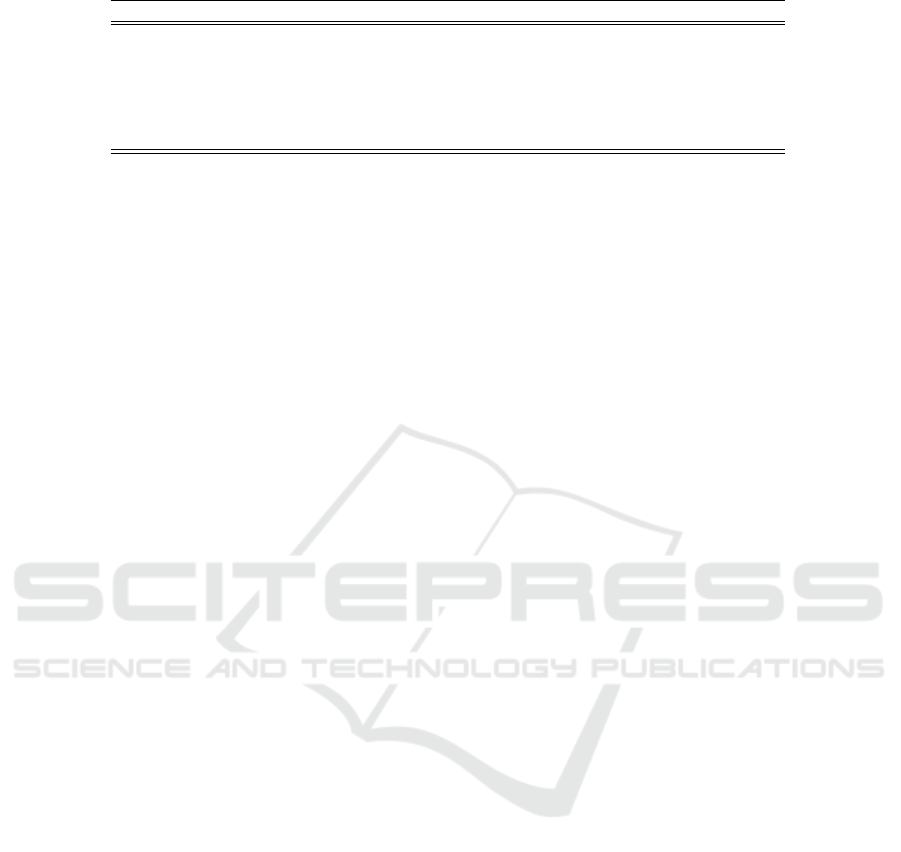
Table 2: Ablation Study.
Training Nums Method Accuracy % Improvement % GCN-Channels
All-Training Samples
Base 89.02 - -
Base+GCN 91.03 2.01 128-64-32
Base+AGN 92.04 3.02 128-64-32
Base+BN 90.20 1.18 -
Ours-concat 92.12 3.10 128-64-32
Ours-residual 93.59 4.57 128-64-32
L. A., and Jemal, A. (2018). Global cancer statistics
2018: Globocan estimates of incidence and mortality
worldwide for 36 cancers in 185 countries. CA: a can-
cer journal for clinicians, 68(6):394–424.
Buty, M., Xu, Z., Gao, M., Bagci, U., Wu, A., and Mollura,
D. J. (2016). Characterization of lung nodule malig-
nancy using hybrid shape and appearance features. In
International Conference on Medical Image Comput-
ing and Computer-Assisted Intervention, pages 662–
670. Springer.
Dey, R., Lu, Z., and Hong, Y. (2018). Diagnostic classifi-
cation of lung nodules using 3d neural networks. In
2018 IEEE 15th International Symposium on Biomed-
ical Imaging (ISBI 2018), pages 774–778. IEEE.
Eaton, D. and Murphy, K. (2012). Bayesian structure learn-
ing using dynamic programming and mcmc. arXiv
preprint arXiv:1206.5247.
Elidan, G. (2010). Copula bayesian networks. In Advances
in neural information processing systems, pages 559–
567.
Ferrari, V. and Zisserman, A. (2008). Learning visual at-
tributes. In Advances in neural information process-
ing systems, pages 433–440.
Hussein, S., Gillies, R., Cao, K., Song, Q., and Bagci,
U. (2017). Tumornet: Lung nodule characteriza-
tion using multi-view convolutional neural network
with gaussian process. In 2017 IEEE 14th Inter-
national Symposium on Biomedical Imaging (ISBI
2017), pages 1007–1010. IEEE.
Jiang, H., Wang, R., Shan, S., Yang, Y., and Chen, X.
(2017). Learning discriminative latent attributes for
zero-shot classification. In Proceedings of the IEEE
International Conference on Computer Vision, pages
4223–4232.
Kingma, D. P. and Ba, J. (2014). Adam: A
method for stochastic optimization. arXiv preprint
arXiv:1412.6980.
Kipf, T. N. and Welling, M. (2016). Semi-supervised clas-
sification with graph convolutional networks. arXiv
preprint arXiv:1609.02907.
Kumar, N., Berg, A. C., Belhumeur, P. N., and Nayar, S. K.
(2009). Attribute and simile classifiers for face veri-
fication. In 2009 IEEE 12th international conference
on computer vision, pages 365–372. IEEE.
Lampert, C. H., Nickisch, H., and Harmeling, S. (2013).
Attribute-based classification for zero-shot visual ob-
ject categorization. IEEE transactions on pattern
analysis and machine intelligence, 36(3):453–465.
Liang, K., Chang, H., Ma, B., Shan, S., and Chen, X.
(2018). Unifying visual attribute learning with ob-
ject recognition in a multiplicative framework. IEEE
transactions on pattern analysis and machine intelli-
gence, 41(7):1747–1760.
Liang, K., Guo, Y., Chang, H., and Chen, X. (2017). In-
complete attribute learning with auxiliary labels. In
IJCAI, pages 2252–2258.
Liu, L., Dou, Q., Chen, H., Olatunji, I. E., Qin, J., and Heng,
P.-A. (2018). Mtmr-net: Multi-task deep learning with
margin ranking loss for lung nodule analysis. In Deep
Learning in Medical Image Analysis and Multimodal
Learning for Clinical Decision Support, pages 74–82.
Springer.
Liu, Z., Luo, P., Wang, X., and Tang, X. (2015). Deep
learning face attributes in the wild. In Proceedings
of the IEEE international conference on computer vi-
sion, pages 3730–3738.
Meng, Z., Adluru, N., Kim, H. J., Fung, G., and Singh,
V. (2018). Efficient relative attribute learning using
graph neural networks. In Proceedings of the Euro-
pean conference on computer vision (ECCV), pages
552–567.
Min, W., Mei, S., Liu, L., Wang, Y., and Jiang, S. (2019).
Multi-task deep relative attribute learning for visual
urban perception. IEEE Transactions on Image Pro-
cessing, 29:657–669.
Paszke, A., Gross, S., Massa, F., Lerer, A., Bradbury, J.,
Chanan, G., Killeen, T., Lin, Z., Gimelshein, N.,
Antiga, L., et al. (2019). Pytorch: An imperative
style, high-performance deep learning library. In
Advances in Neural Information Processing Systems,
pages 8024–8035.
Pearl, J. (1982). Reverend bayes on inference engines:
a distributed hierarchical approach. In Proceedings
of the Second AAAI Conference on Artificial Intelli-
gence, pages 133–136.
Pearl, J. (1998). Bayesian networks. In The handbook
of brain theory and neural networks, pages 149–153.
MIT Press.
Pearl, J. (2014). Probabilistic reasoning in intelligent sys-
tems: networks of plausible inference. Elsevier.
Rohekar, R. Y., Nisimov, S., Gurwicz, Y., Koren, G., and
Novik, G. (2018). Constructing deep neural net-
works by bayesian network structure learning. In
Advances in Neural Information Processing Systems,
pages 3047–3058.
Shen, S., Han, S. X., Aberle, D. R., Bui, A. A., and Hsu, W.
(2019). An interpretable deep hierarchical semantic
convolutional neural network for lung nodule malig-
nancy classification. Expert systems with applications,
128:84–95.
Attribute Relation Modeling for Pulmonary Nodule Malignancy Reasoning
65