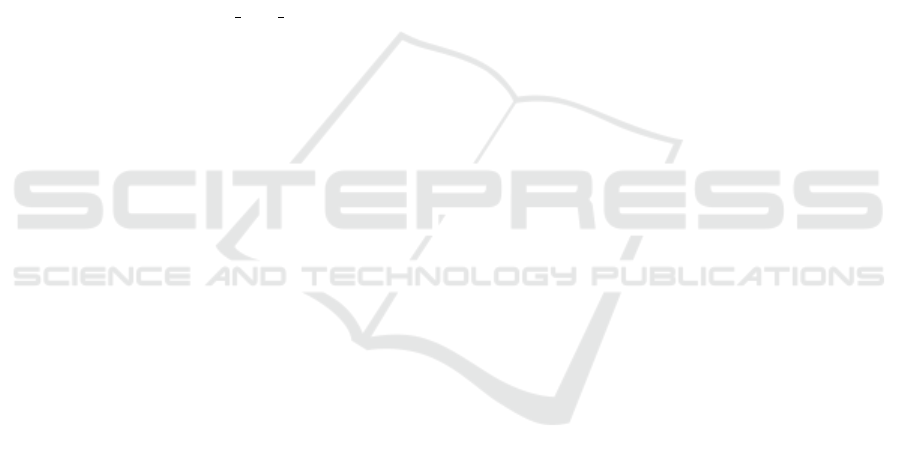
son nano, the accuracy increases up to 86% due to
the dedicated GPU that process Convolutional Neural
Networks(CNN).
The use of better detection datasets would in-
crease the obtained mAP, mainly in the detection of
powdery mildew disease, where the average precision
was of 75% in the EfficientDet-D0 best train iteration.
Moreover, an optimization step regarding the Nvidia
Jetson nano with TensorRT would decrease the infer-
ence time. We will address this in our future work for
the implementation of the algorithm in the CERES
agro-robot (Santiago. et al., 2020).
ACKNOWLEDGEMENTS
This work is funded by Universidad Militar Nueva
Granada- Vicerrectoria de Investigaciones, under re-
search grant for project INV ING 3185 “Sistema de
toma de decisiones para la aplicaci
´
on de medidas cor-
rectivas que ayuden a mantener la salud de un cultivo
de hortalizas utilizando un robot (CERES) dedicado a
labores de agricultura”.
REFERENCES
Carisse, O. and Fall, M. (2021). Decision trees to
forecast risks of strawberry powdery mildew caused
by podosphaera aphanis. Agriculture (Switzerland),
11(1):1–16. cited By 0.
Chen, J., Yin, H., and Zhang, D. (2020). A self-adaptive
classification method for plant disease detection using
gmdh-logistic model. Sustainable Computing: Infor-
matics and Systems, 28:100415.
Chouhan, S., Singh, D. U., Sharma, U., and Jain, S. (2020).
Leaf disease segmentation and classification of jat-
ropha curcas l. and pongamia pinnata l. biofuel plants
using computer vision based approaches. Measure-
ment, 171.
Ciaparrone, G., S
´
anchez, F. L., Tabik, S., Troiano, L., Tagli-
aferri, R., and Herrera, F. (2020). Deep learning in
video multi-object tracking: A survey. Neurocomput-
ing, 381:61 – 88.
Girshick, R. (2015). Fast r-cnn. In 2015 IEEE International
Conference on Computer Vision (ICCV), pages 1440–
1448.
Girshick, R. B., Donahue, J., Darrell, T., and Malik, J.
(2013). Rich feature hierarchies for accurate ob-
ject detection and semantic segmentation. CoRR,
abs/1311.2524.
Gomez Selvaraj, M., Vergara, A., Montenegro, F., Alonso
Ruiz, H., Safari, N., Raymaekers, D., Ocimati, W.,
Ntamwira, J., Tits, L., Omondi, A. B., and Blomme,
G. (2020). Detection of banana plants and their major
diseases through aerial images and machine learning
methods: A case study in dr congo and republic of
benin. ISPRS Journal of Photogrammetry and Remote
Sensing, 169:110 – 124.
Hancock, J., Sjulin, T., and Lobos, G. (2008). Strawberries,
volume 9781402069079. cited By 36.
He, K., Zhang, X., Ren, S., and Sun, J. (2015). Deep
residual learning for image recognition. CoRR,
abs/1512.03385.
Huang, J.-D., Wang, C.-F., Lian, C.-L., Huang, M.-Y.,
Zhang, C., and Liu, J.-Q. (2020). Isolation and identi-
fication of five new diterpenoids from jatropha curcas.
Phytochemistry Letters, 40:37–41.
Klerkx, L., Jakku, E., and Labarthe, P. (2019). A review
of social science on digital agriculture, smart farming
and agriculture 4.0: New contributions and a future
research agenda. NJAS - Wageningen Journal of Life
Sciences, 90-91:100315.
Lezoche, M., Hernandez, J. E., del Mar Eva Alemany D
´
ıaz,
M., Panetto, H., and Kacprzyk, J. (2020). Agri-food
4.0: A survey of the supply chains and technologies
for the future agriculture. Computers in Industry,
117:103187.
Liu, W., Anguelov, D., Erhan, D., Szegedy, C., Reed, S. E.,
Fu, C., and Berg, A. C. (2015). SSD: single shot multi-
box detector. CoRR, abs/1512.02325.
Mathew, D., Sathish Kumar, C., and Anita Cherian, K.
(2020). Foliar fungal disease classification in banana
plants using elliptical local binary pattern on multires-
olution dual tree complex wavelet transform domain.
Information Processing in Agriculture.
¨
Umit Atila, Uc¸ar, M., Akyol, K., and Uc¸ar, E. (2021).
Plant leaf disease classification using efficientnet deep
learning model. Ecological Informatics, 61:101182.
Mojjada, R. K., Kiran Kumar, K., Yadav, A., and Satya Vara
Prasad, B. (2020). Detection of plant leaf disease us-
ing digital image processing. Materials Today: Pro-
ceedings.
Park, H., Eun, J., and Kim, S. (2017). Image-based dis-
ease diagnosing and predicting of the crops through
the deep learning mechanism. In 2017 International
Conference on Information and Communication Tech-
nology Convergence (ICTC), pages 129–131.
Petrasch, S., Knapp, S. J., van Kan, J. A. L., and Blanco-
Ulate, B. (2019). Grey mould of strawberry, a devas-
tating disease caused by the ubiquitous necrotrophic
fungal pathogenBotrytis cinerea. Molecular Plant
Pathology, 20(6):877–892.
Prakash, R. M., Saraswathy, G. P., Ramalakshmi, G., Man-
galeswari, K. H., and Kaviya, T. (2017). Detection
of leaf diseases and classification using digital image
processing. In 2017 International Conference on In-
novations in Information, Embedded and Communi-
cation Systems (ICIIECS), pages 1–4.
P
´
erez-Borrero, I., Mar
´
ın-Santos, D., Geg
´
undez-Arias,
M. E., and Cort
´
es-Ancos, E. (2020). A fast and ac-
curate deep learning method for strawberry instance
segmentation. Computers and Electronics in Agricul-
ture, 178:105736.
Priya, R. and Ramesh, D. (2020). Ml based sustainable
precision agriculture: A future generation perspec-
Strawberry Disease Detection in Precision Agriculture
543