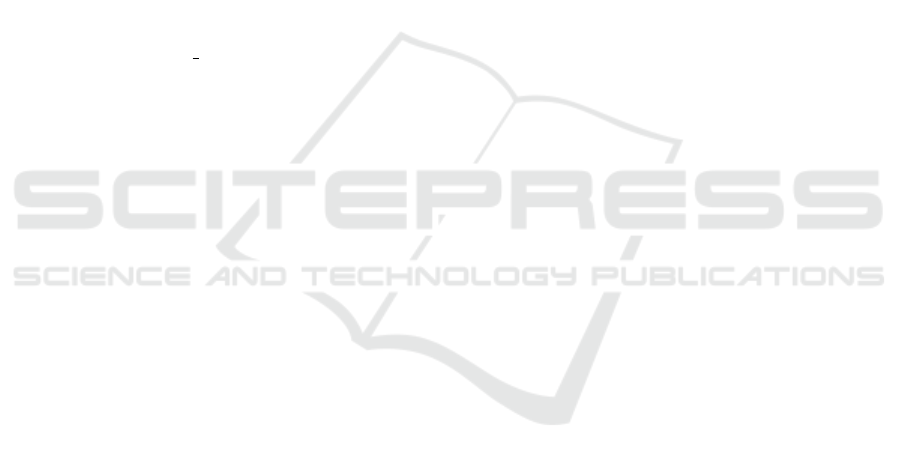
5 CONCLUSION
This paper presented a completely new approach to
synthesize retinal fundus photographs and using the
synthetic images for CNN training. In comparison to
other state-of-the-art approaches like the method pre-
sented in (Costa et al., 2017b), the proposed synthe-
sizing method generates a very realistic vessels tree
without unconnected vessels. The final synthesized
image is a realistic image that achieves state-of-the-art
performance in segmentation networks without com-
plex preprocessing and can, therefore, be used to en-
large training sets and solve the problem of lacking
training data. The proposed approach improved the
performance of vessel segmentation as shown quanti-
tatively and qualitatively from the conducted experi-
ments and the comparison against state-of-the-art reti-
nal vessel segmentation.
As future work, we will consider different
databases that are used in retinal vessel segmentation
such as HRF or CHASE DB1 to be synthesized which
includes various disease patterns. The synthesizing
process will be adjustable to generate more realistic
images with different resolutions and generalize the
statistical shapes of different real databases.
Finally, it is stressed that due to the highly ad-
justable pipeline, the generated images are easily use-
able for optic disc segmentation and fovea localiza-
tion tasks.
REFERENCES
Abr
`
amoff, M. D., Garvin, M. K., and Sonka, M. (2010).
Retinal imaging and image analysis. IEEE reviews in
biomedical engineering, 3:169–208.
Al-Diri, B., Hunter, A., and Steel, D. (2009). An ac-
tive contour model for segmenting and measuring reti-
nal vessels. IEEE Transactions on Medical imaging,
28(9):1488–1497.
Bonaldi, L., Menti, E., Ballerini, L., Ruggeri, A., and
Trucco, E. (2016). Automatic generation of synthetic
retinal fundus images: Vascular network. In MIUA,
pages 54–60.
Budai, A., Bock, R., Maier, A., Hornegger, J., and Michel-
son, G. (2013). Robust vessel segmentation in fundus
images. International journal of biomedical imaging,
2013.
Castro, D. L., Valenti, C., and Tegolo, D. (2020). Retinal
image synthesis through the least action principle. In
2020 5th International Conf. on Intelligent Informat-
ics and Biomedical Sciences (ICIIBMS), pages 111–
116. IEEE.
Chaudhuri, S., Chatterjee, S., Katz, N., Nelson, M., and
Goldbaum, M. (1989). Detection of blood vessels in
retinal images using two-dimensional matched filters.
IEEE Transactions on medical imaging, 8(3):263–
269.
Costa, P., Galdran, A., Meyer, M. I., Mendonc¸a, A. M., and
Campilho, A. (2017a). Adversarial synthesis of reti-
nal images from vessel trees. In International Confer-
ence Image Analysis and Recognition, pages 516–523.
Springer.
Costa, P., Galdran, A., Meyer, M. I., Niemeijer, M.,
Abr
`
amoff, M., Mendonc¸a, A. M., and Campilho,
A. (2017b). End-to-end adversarial retinal image
synthesis. IEEE transactions on medical imaging,
37(3):781–791.
Feng, S., Zhuo, Z., Pan, D., and Tian, Q. (2020). Ccnet: A
cross-connected convolutional network for segment-
ing retinal vessels using multi-scale features. Neuro-
computing, 392:268–276.
Fiorini, S., Ballerini, L., Trucco, E., and Ruggeri, A. (2014).
Automatic generation of synthetic retinal fundus im-
ages. In Eurographics Italian Chapter Conference,
pages 41–44.
Guibas, J. T., Virdi, T. S., and Li, P. S. (2017). Synthetic
medical images from dual generative adversarial net-
works. arXiv preprint arXiv:1709.01872.
Hoover, A., Kouznetsova, V., and Goldbaum, M. (2000).
Locating blood vessels in retinal images by piecewise
threshold probing of a matched filter response. IEEE
Transactions on Medical imaging, 19(3):203–210.
Hu, K., Zhang, Z., Niu, X., Zhang, Y., Cao, C., Xiao, F.,
and Gao, X. (2018). Retinal vessel segmentation of
color fundus images using multiscale convolutional
neural network with an improved cross-entropy loss
function. Neurocomputing, 309:179–191.
Ibtehaz, N. and Rahman, M. S. (2020). Multiresunet:
Rethinking the u-net architecture for multimodal
biomedical image segmentation. Neural Networks,
121:74 – 87.
Joshua, A. O., Nelwamondo, F. V., and Mabuza-Hocquet,
G. (2020). Blood vessel segmentation from fundus
images using modified u-net convolutional neural net-
work. Journal of Image and Graphics, 8(1).
Liskowski, P. and Krawiec, K. (2016a). Segmenting reti-
nal blood vessels with deep neural networks. IEEE
transactions on medical imaging, 35(11):2369–2380.
Liskowski, P. and Krawiec, K. (2016b). Segmenting reti-
nal blood vessels with deep neural networks. IEEE
transactions on medical imaging, 35(11):2369–2380.
Mart
´
ınez-P
´
erez, M. E., Hughes, A. D., Stanton, A. V.,
Thom, S. A., Bharath, A. A., and Parker, K. H.
(1999). Retinal blood vessel segmentation by means
of scale-space analysis and region growing. In In-
ternational Conference on Medical Image Comput-
ing and Computer-Assisted Intervention, pages 90–
97. Springer.
Melin
ˇ
s
ˇ
cak, M., Prenta
ˇ
si
´
c, P., and Lon
ˇ
cari
´
c, S. (2015). Reti-
nal vessel segmentation using deep neural networks.
In 10th International Conference on Computer Vision
Theory and Applications (VISAPP 2015).
Ngo, L. and Han, J.-H. (2017). Multi-level deep neural
network for efficient segmentation of blood vessels
Synthesizing Fundus Photographies for Training Segmentation Networks
77