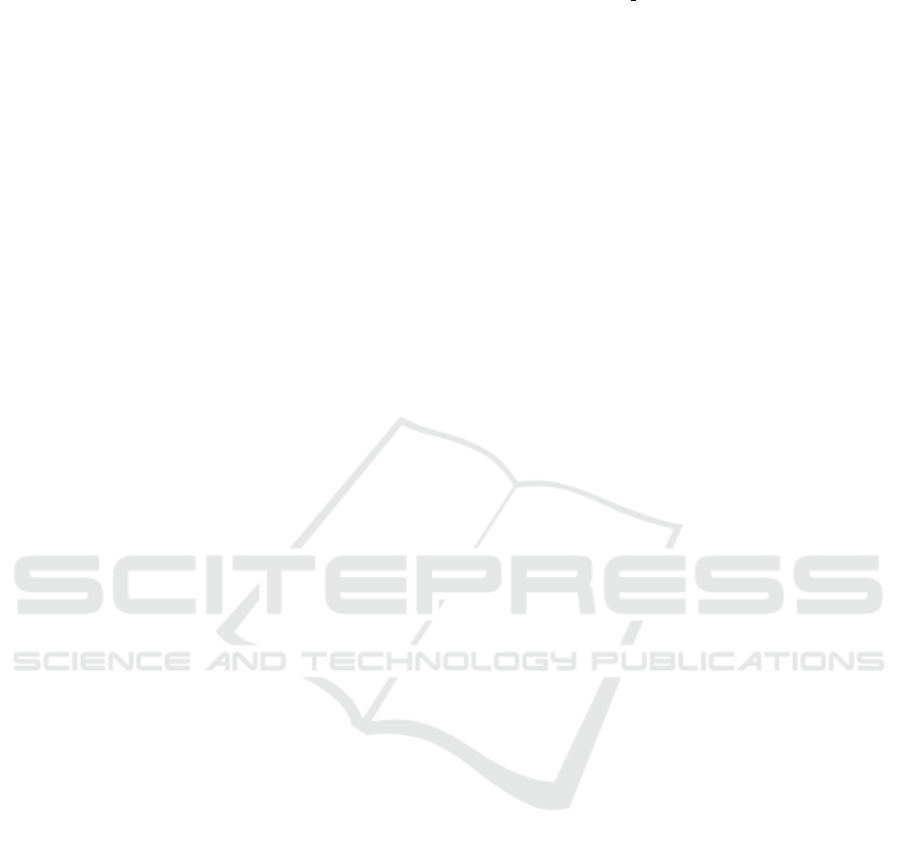
Darktrace (2017). Darktrace industrial uses
machine learning to identify cyber cam-
paigns targeting critical infrastructure.
https://www.darktrace.com/en/press/2017/204/.
Das, R. and Morris, T. H. (2017). Machine learning and cy-
ber security. 2017 International Conference on Com-
puter, Electrical & Communication Engineering (IC-
CECE), Computer, Electrical & Communication En-
gineering (ICCECE), 2017 International Conference
on, pages 1 – 7.
DCMS (2021). Cyber security breaches survey
2021. Technical report, UK Government.
https://www.gov.uk/government/statistics/cyber-
security-breaches-survey-2021/cyber-security-
breaches-survey-2021.
Elmrabit, N., Zhou, F., Li, F., and Zhou, H. (2020). Evalu-
ation of machine learning algorithms for anomaly de-
tection. 2020 International Conference on Cyber Se-
curity and Protection of Digital Services (Cyber Se-
curity), Cyber Security and Protection of Digital Ser-
vices (Cyber Security), 2020 International Conference
on, pages 1 – 8.
Fransen, F. . . ., Kerkdijk, R. . . ., and Smulders, A. . . .
(2015). Cyber security information exchange to gain
insight into the effects of cyber threats and incidents.
Elektrotechnik und Informationstechnik, 132(2):106–
112.
Gupta, B., S. Q. (2019). Machine learning for computer and
cyber security : principles, algorithms, and practices.
CRC Press.
Johnson, C., B. L. W. D. S. J. S. C. (2019). NIST Special
Publication 800-150: Guide to Cyber Threat Infor-
mation Sharing. National Institute for Standards and
Technology.
Jungsoo, P., Long Nguyen, V., Bencivengo, G., and Souh-
wan, J. (2020). Automatic generation of maec and
stix standards for android malware threat intelligence.
KSII Transactions on Internet & Information Systems,
14(8):3420 – 3436.
KALOUDI, N. and JINGYUE, L. (2020). The ai-based cy-
ber threat landscape: A survey. ACM Computing Sur-
veys, 53(1):1 – 34.
Kumar, S.R, Y. S. S. S. S. A. (2016). Recommendations
for effective cyber security execution. In 2016 Inter-
national Conference on Innovation and Challenges in
Cyber Security (ICICCS-INBUSH), pages 342–346.
MITRE (2021). Mitre att&ck. https://cti-
taxii.mitre.org/stix/collections/95ecc380-afe9-11e4-
9b6c-751b66dd541e/objects/.
Mittal, S., J. A. F. T. (2017). Thinking, fast and slow: Com-
bining vector spaces and knowledge graphs. CoRR,
abs/1708.03310. http://arxiv.org/abs/1708.03310.
Mugan, J. (2013). A developmental approach to learning
causal models for cyber security. volume 8751, page
87510A.
NCSC (2021). Cyber information sharing partner-
ship. https://www.ncsc.gov.uk/section/keep-up-to-
date/cisp.
OASIS (2021a). Stix 2.1 bundle specification.
https://docs.oasis-open.org/cti/stix/v2.1/cs02/stix-
v2.1-cs02.html#
gms872kuzdmg.
OASIS (2021b). STIX Version 2.1. https://docs.oasis-
open.org/cti/stix/v2.1/cs02/stix-v2.1-cs02.html.
PaloAlto (2021). Expanse - attack surface reduction.
https://expanse.co/attack-surface-reduction/.
Riesco, R., Larriva-Novo, X., and Villagra, V. A. (2020).
Cybersecurity threat intelligence knowledge exchange
based on blockchain: Proposal of a new incentive
model based on blockchain and smart contracts to fos-
ter the cyber threat and risk intelligence exchange of
information. Telecommunication Systems, 73(2):259
– 288.
Riesco, R. and Villagr
´
a, V. A. (2019). Leveraging cyber
threat intelligence for a dynamic risk framework: Au-
tomation by using a semantic reasoner and a new com-
bination of standards (stix
TM
, swrl and owl). Inter-
national Journal of Information Security, 18(6):715 –
739.
Scheau, M., Arsene, A.-L., and Popescu, G. (2018). Ar-
tificial intelligence / machine learning challenges and
evolution. 7:11–22.
Shaukat, K., Luo, S., Varadharajan, V., Hameed, I., and Xu,
M. (2020). A survey on machine learning techniques
for cyber security in the last decade. IEEE Access,
8:222310–222354.
Smart, W. (2018). Lessons learned review of the
wannacry ransomware cyber attack. Tech-
nical report, Department for Health and So-
cial Care. https://www.england.nhs.uk/wp-
content/uploads/2018/02/lessons-learned-review-
wannacry-ransomware-cyber-attack-cio-review.pdf.
Vectra.ai (2021). Vectra.ai - how we do it.
https://www.vectra.ai/products/how-we-do-it.
Wali, A., Soon Ae, C., and Geller, J. (2013). A bootstrap-
ping approach for developing a cyber-security ontol-
ogy using textbook index terms. 2013 International
Conference on Availability, Reliability and Security,
Availability, Reliability and Security (ARES), 2013
Eighth International Conference on, Availability, Re-
liability and Security (ARES), 2012 Seventh Interna-
tional Conference on, pages 569 – 576.
Xu, J., Wen, Y., Yang, C., and Meng, D. (2020). An ap-
proach for poisoning attacks against rnn-based cyber
anomaly detection. 2020 IEEE 19th International
Conference on Trust, Security and Privacy in Com-
puting and Communications (TrustCom), Trust, Secu-
rity and Privacy in Computing and Communications
(TrustCom), 2020 IEEE 19th International Confer-
ence on, TRUSTCOM, pages 1680 – 1687.
Zheng, H., Wang, Y., Han, C., Le, F., He, R., and Lu,
J. (2018). Learning and applying ontology for ma-
chine learning in cyber attack detection. 2018 17th
IEEE International Conference On Trust, Security
And Privacy In Computing And Communications/
12th IEEE International Conference On Big Data
Science And Engineering (TrustCom/BigDataSE),
Trust, Security And Privacy In Computing And
Communications/ 12th IEEE International Confer-
ence On Big Data Science And Engineering (Trust-
Com/BigDataSE), 2018 17th IEEE International Con-
The LeWiS Method: Target Variable Estimation using Cyber Security Intelligence
25