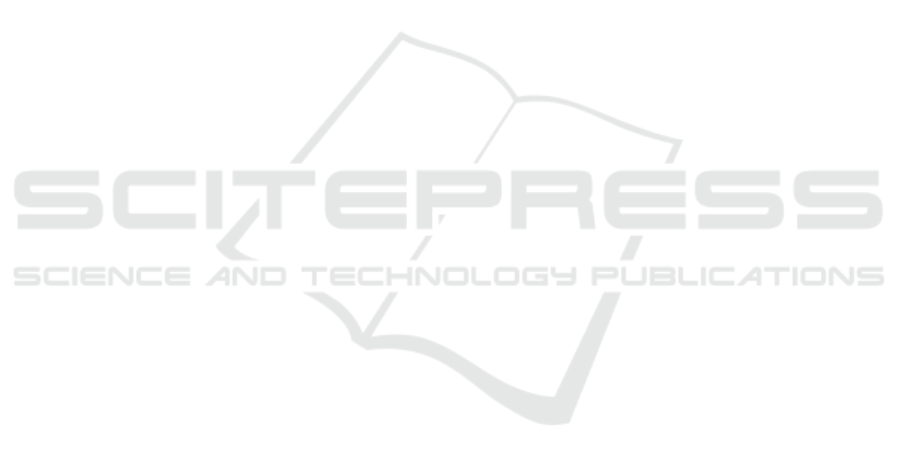
for bird species identification in comparison to the ex-
isting works. In conclusion, the proposed method can
be deemed suitable for practical bio-acoustic monitor-
ing of bird species in terrestrial environments and can
be considered for deployment on a wider scale.
Future extensions of the work will explore vali-
dation of the proposed RoBINN framework using an
external dataset and study of recent transformer-based
DL model for bird species identification.
REFERENCES
Chakraborty, D., Mukker, P., Rajan, P., and Dileep, A. D.
(2016). Bird call identification using dynamic kernel
based support vector machines and deep neural net-
works. In 2016 15th IEEE International Conference
on Machine Learning and Applications (ICMLA),
pages 280–285. IEEE.
Cornell Lab of Ornithology (2020). Cornell Bird-
call Identification. https://www.kaggle.com/c/
birdsong-recognition. Online; accessed 15 Oc-
tober 2020.
Gyires-T
´
oth, B. and Czeba, B. (2016). Convolutional neu-
ral networks for large-scale bird song classification in
noisy environment.
Incze, A., Jancs
´
o, H.-B., Szil
´
agyi, Z., Farkas, A., and Su-
lyok, C. (2018). Bird sound recognition using a con-
volutional neural network. In 2018 IEEE 16th Inter-
national Symposium on Intelligent Systems and Infor-
matics (SISY), pages 000295–000300. IEEE.
Ioffe, S. and Szegedy, C. (2015). Batch normalization: Ac-
celerating deep network training by reducing internal
covariate shift. arXiv preprint arXiv:1502.03167.
Kahl, S., St
¨
oter, F.-R., Go
¨
eau, H., Glotin, H., Planque, R.,
Vellinga, W.-P., and Joly, A. (2019). Overview of
birdclef 2019: large-scale bird recognition in sound-
scapes. In Working Notes of CLEF 2019-Conference
and Labs of the Evaluation Forum, number 2380,
pages 1–9. CEUR.
Knight, E. C., Poo Hernandez, S., Bayne, E. M., Bulitko,
V., and Tucker, B. V. (2020). Pre-processing spectro-
gram parameters improve the accuracy of bioacous-
tic classification using convolutional neural networks.
Bioacoustics, 29(3):337–355.
Koh, C.-Y., Chang, J.-Y., Tai, C.-L., Huang, D.-Y., Hsieh,
H.-H., and Liu, Y.-W. (2019). Bird sound classifica-
tion using convolutional neural networks. In CLEF
(Working Notes).
Kong, Q., Cao, Y., Iqbal, T., Wang, Y., Wang, W., and
Plumbley, M. D. (2020). Panns: Large-scale pre-
trained audio neural networks for audio pattern recog-
nition. IEEE/ACM Transactions on Audio, Speech,
and Language Processing, 28:2880–2894.
Lasseck, M. (2018). Audio-based Bird Species Identifica-
tion with Deep Convolutional Neural Networks. In
CLEF (Working Notes).
Misra, D. (2019). Mish: A self regularized non-
monotonic neural activation function. arXiv preprint
arXiv:1908.08681.
Park, D. S., Chan, W., Zhang, Y., Chiu, C.-C., Zoph, B.,
Cubuk, E. D., and Le, Q. V. (2019). Specaugment:
A simple data augmentation method for automatic
speech recognition. arXiv preprint arXiv:1904.08779.
Planqu
´
e, B., Vellinga, W., Pieterse, S., and Jongsma, J.
(2005). Xeno-canto: sharing bird sounds from around
the world.
Priyadarshani, N., Marsland, S., and Castro, I. (2018). Au-
tomated birdsong recognition in complex acoustic en-
vironments: a review. Journal of Avian Biology,
49(5):jav–01447.
Sang, J., Park, S., and Lee, J. (2018). Convolutional re-
current neural networks for urban sound classification
using raw waveforms. pages 2444–2448.
Sankupellay, M. and Konovalov, D. (2018). Bird call recog-
nition using deep convolutional neural network resnet-
50. In Proc. ACOUSTICS, volume 7, pages 1–8.
Schl
¨
uter, J. and Grill, T. (2015). Exploring data augmenta-
tion for improved singing voice detection with neural
networks. In ISMIR, pages 121–126.
Woo, S., Park, J., Lee, J.-Y., and So Kweon, I. (2018).
Cbam: Convolutional block attention module. In Pro-
ceedings of the European conference on computer vi-
sion (ECCV), pages 3–19.
Xie, J., Hu, K., Zhu, M., Yu, J., and Zhu, Q. (2019). Inves-
tigation of different cnn-based models for improved
bird sound classification. IEEE Access, 7:175353–
175361.
Yan, G., Wang, M., Liu, X., and Song, X. (2019).
Sound event recognition based in feature combina-
tion with low snr. In 2019 International Conference
on Artificial Intelligence and Advanced Manufactur-
ing (AIAM), pages 109–114.
Yi-de, M., Qing, L., and Zhi-Bai, Q. (2004). Automated im-
age segmentation using improved PCNN model based
on cross-entropy. In Proceedings of 2004 Interna-
tional Symposium on Intelligent Multimedia, Video
and Speech Processing, 2004., pages 743–746. IEEE.
Zhang, H., Cisse, M., Dauphin, Y. N., and Lopez-Paz, D.
(2017). mixup: Beyond empirical risk minimization.
arXiv preprint arXiv:1710.09412.
Zhao, Z., Zhang, S.-h., Xu, Z.-y., Bellisario, K., Dai, N.-h.,
Omrani, H., and Pijanowski, B. C. (2017). Automated
bird acoustic event detection and robust species clas-
sification. Ecological Informatics, 39:99–108.
SIGMAP 2021 - 18th International Conference on Signal Processing and Multimedia Applications
38