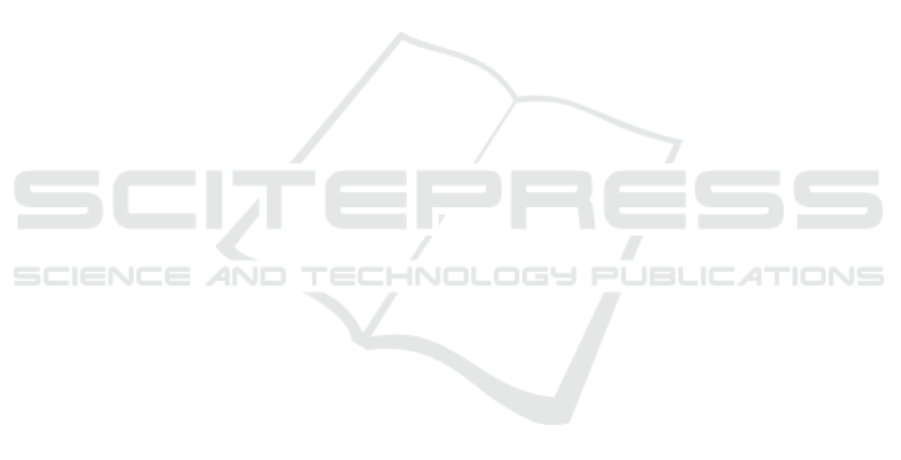
4.3.3, it is possible that shot type labels can go ig-
nored and/or be assimilated by other labels if there
are insufficient instances, or the model is unable to
learn features to define it from a similar class.
REFERENCES
Er
ˇ
culj, F. and
ˇ
Strumbelj, E. (2015). Basketball shot types
and shot success in different levels of competitive bas-
ketball. Plos One, 10(6).
ESPN (2020).
Foster, B. T. and Binns, M. D. (2019). Analytics for the
front office: Valuing protections on nba draft picks.
MIT SLOAN Sports Analytics Conference, 13.
Goyal, R., Kahou, S. E., Michalski, V., Materzynska, J.,
Westphal, S., Kim, H., Haenel, V., Fr
¨
und, I., Yianilos,
P., Mueller-Freitag, M., Hoppe, F., Thurau, C., Bax,
I., and Memisevic, R. (2017). The ”something some-
thing” video database for learning and evaluating vi-
sual common sense. CoRR, abs/1706.04261.
Gu, X., Xue, X., and Wang, F. (2020). Fine-grained action
recognition on a novel basketball dataset. In ICASSP
2020 - 2020 IEEE International Conference on Acous-
tics, Speech and Signal Processing (ICASSP), pages
2563–2567.
Johnson, N. (2020). Extracting player tracking data from
video using non-stationary cameras and a combination
of computer vision techniques. MIT SLOAN Sports
Analytics Conference, 14.
Kaplan, S., Ramamoorthy, V., Gupte, C., Sagar, A.,
Premkumar, D., Wilbur, J., and Zilberman, D. (2019).
The economic impact of nba superstars: Evidence
from missed games using ticket microdata from a sec-
ondary marketplace. MIT SLOAN Sports Analytics
Conference, 13.
Karpathy, A., Toderici, G., Shetty, S., Leung, T., Suk-
thankar, R., and Fei-Fei, L. (2014). Large-scale video
classification with convolutional neural networks. In
CVPR.
Materzynska, J., Berger, G., Bax, I., and Memisevic, R.
(2019). The jester dataset: A large-scale video dataset
of human gestures. 2019 IEEE/CVF International
Conference on Computer Vision Workshop (ICCVW).
Monfort, M., Andonian, A., Zhou, B., Ramakrishnan, K.,
Bargal, S. A., Yan, T., Brown, L., Fan, Q., Gutfru-
end, D., Vondrick, C., et al. (2019). Moments in time
dataset: one million videos for event understanding.
IEEE Transactions on Pattern Analysis and Machine
Intelligence, pages 1–8.
Mortensen, J. and Bornn, L. (2019). From markov mod-
els to poisson point processes: Modeling movement
in the nba. MIT SLOAN Sports Analytics Conference,
13.
Nistala Akhil, G. J. (2019). Using deep learning to under-
stand patterns of player movement in the nba. MIT
SLOAN Sports Analytics Conference, 13.
Sigurdsson, G. A., Varol, G., Wang, X., Farhadi, A.,
Laptev, I., and Gupta, A. (2016). Hollywood in
homes: Crowdsourcing data collection for activity un-
derstanding. CoRR, abs/1604.01753.
Tien, M.-C., Huang, C., Chen, Y.-W., Hsiao, M.-H., and
Lee, S.-Y. (2007). Shot classification of basketball
videos and its application in shooting position extrac-
tion. volume 1, pages I–1085.
Tran, L., Yin, X., and Liu, X. (2019). Representa-
tion learning by rotating your faces. IEEE Transac-
tions on Pattern Analysis and Machine Intelligence,
41(12):3007–3021.
Wang, L., Xiong, Y., Wang, Z., Qiao, Y., Lin, D., Tang, X.,
and Gool, L. V. (2016). Temporal segment networks:
Towards good practices for deep action recognition.
CoRR, abs/1608.00859.
Williams, C. Q., Webster, L., Spaniol, F., and Bonnette, R.
(2020). The effect of foot placement on the jump shot
accuracy of ncaa division i basketball players. The
Sport Journal, 21.
Zhen, L., Wang, L., and Hao, Z. (2016). A biomechanical
analysis of basketball shooting.
Zhou, B., Andonian, A., Oliva, A., and Torralba, A. (2018).
Temporal relational reasoning in videos. Computer
Vision – ECCV 2018 Lecture Notes in Computer Sci-
ence, page 831–846.
Analysis of Deep Learning Action Recognition for Basketball Shot Type Identification
27