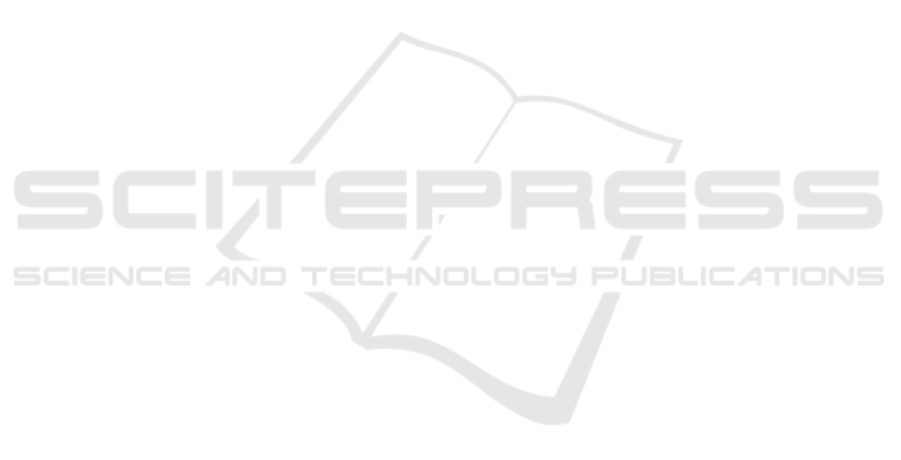
REFERENCES
Agarwal., A. and Bansal., V. (2020). Exploring sentiments
of voters through social media content: A case study
of 2017 assembly elections of three states in india. In
Proceedings of the 22nd International Conference on
Enterprise Information Systems - Volume 1: ICEIS,,
pages 596–602. INSTICC, SciTePress.
Banthia, M., Goel, S., Deepanshi, and Sinha, A. (2020).
Geo-spatial analysis of information outreach in twitter
social network. In Singh, P. K., Sood, S., Kumar, Y.,
Paprzycki, M., Pljonkin, A., and Hong, W.-C., editors,
Futuristic Trends in Networks and Computing Tech-
nologies, pages 444–455, Singapore. Springer Singa-
pore.
Barnaghi, P., Ghaffari, P., and Breslin, J. G. (2016). Opinion
Mining and Sentiment Polarity on Twitter and Corre-
lation between Events and Sentiment. Proceedings -
2016 IEEE 2nd International Conference on Big Data
Computing Service and Applications, BigDataService
2016, pages 52–57.
Del-Alc
´
azar, J. (2021). Ecuador Estado Digital Ene/21.
Mentinno - Formaci
´
on Gerencial - New Media.
Feinerer, I., Hornik, K., and Meyer, D. (2008). Text min-
ing infrastructure in r. Journal of Statistical Software,
25(5):1–54.
Gaurav, M., Kumar, A., Srivastava, A., and Miller, S.
(2013). Leveraging candidate popularity on Twitter
to predict election outcome. Proceedings of the 7th
Workshop on Social Network Mining and Analysis,
SNA-KDD 2013.
Gustisa Wisnu, G. R., Ahmadi, Muttaqi, A. R., Santoso,
A. B., Putra, P. K., and Budi, I. (2020). Sentiment
analysis and topic modelling of 2018 central java gu-
bernatorial election using twitter data. 2020 Interna-
tional Workshop on Big Data and Information Secu-
rity, IWBIS 2020, pages 35–40.
Jhawar, A., Munjal, V., Ranjan, S., and Karmakar, P.
(2020). Social Network based Sentiment and Net-
work Analysis to Predict Elections. Proceedings of
CONECCT 2020 - 6th IEEE International Conference
on Electronics, Computing and Communication Tech-
nologies, pages 0–5.
Kearney, M. W. (2019). rtweet: Collecting and analyzing
twitter data. Journal of Open Source Software, 4(42).
R package version 0.7.0.
Kristiyanti, D. A., Normah, and Umam, A. H. (2019). Pre-
diction of Indonesia presidential election results for
the 2019-2024 period using twitter sentiment analysis.
Proceedings of 2019 5th International Conference on
New Media Studies, CONMEDIA 2019, pages 36–42.
Krouska, A., Troussas, C., and Virvou, M. (2017). Compar-
ative evaluation of algorithms for sentiment analysis
over social networking services. Journal of Universal
Computer Science, 23(8):755–768.
Li, N. and Wu, D. D. (2010). Using text mining and senti-
ment analysis for online forums hotspot detection and
forecast. Decision Support Systems, 48(2):354–368.
Liu, R., Yao, X., Guo, C., and Wei, X. (2021). Can We
Forecast Presidential Election Using Twitter Data?
An Integrative Modelling Approach. Annals of GIS,
27(1):43–56.
Parmelee, J. H. (2014). The agenda-building function of
political tweets. New Media & Society, 16(3):434–
450.
Parraga-Alava, J., Caicedo, R. A., G
´
omez, J. M., and
Inostroza-Ponta, M. (2019). An unsupervised learn-
ing approach for automatically to categorize potential
suicide messages in social media. In 2019 38th Inter-
national Conference of the Chilean Computer Science
Society (SCCC), pages 1–8.
Rofr
´
ıo, D., Ruiz, A., Sosebee, E., Raza, Q., Bashir, A.,
Crandall, J., and Sandoval, R. (2019). Presidential
elections in ecuador: Bot presence in twitter. In 2019
Sixth International Conference on eDemocracy eGov-
ernment (ICEDEG), pages 218–223.
Sharma, A. and Ghose, U. (2020). Sentimental Analysis
of Twitter Data with respect to General Elections in
India. Procedia Computer Science, 173(2019):325–
334.
Sharma, P. and Moh, T. S. (2016). Prediction of Indian elec-
tion using sentiment analysis on Hindi Twitter. Pro-
ceedings - 2016 IEEE International Conference on
Big Data, Big Data 2016, pages 1966–1971.
Singh, P., Dwivedi, Y. K., Kahlon, K. S., Pathania, A., and
Sawhney, R. S. (2020). Can twitter analytics predict
election outcome? An insight from 2017 Punjab as-
sembly elections. Government Information Quarterly,
37(2):101444.
Troussas, C., Krouska, A., and Virvou, M. (2016). Evalua-
tion of ensemble-based sentiment classifiers for twit-
ter data. In 2016 7th International Conference on In-
formation, Intelligence, Systems Applications (IISA),
pages 1–6.
Wang, L. and Gan, J. Q. (2019). Prediction of the 2017
French Election Based on Twitter Data Analysis Us-
ing Term Weighting. 2018 10th Computer Science
and Electronic Engineering Conference, CEEC 2018
- Proceedings, pages 231–235.
Wickham, H., Averick, M., Bryan, J., Chang, W., Mc-
Gowan, L. D., Franc¸ois, R., Grolemund, G., Hayes,
A., Henry, L., Hester, J., Kuhn, M., Pedersen, T. L.,
Miller, E., Bache, S. M., M
¨
uller, K., Ooms, J., Robin-
son, D., Seidel, D. P., Spinu, V., Takahashi, K.,
Vaughan, D., Wilke, C., Woo, K., and Yutani, H.
(2019). Welcome to the tidyverse. Journal of Open
Source Software, 4(43):1686.
Yaqub, U., Sharma, N., Pabreja, R., Chun, S. A., Atluri, V.,
and Vaidya, J. (2020). Location-based sentiment anal-
yses and visualization of twitter election data. Digit.
Gov.: Res. Pract., 1(2).
WEBIST 2021 - 17th International Conference on Web Information Systems and Technologies
266