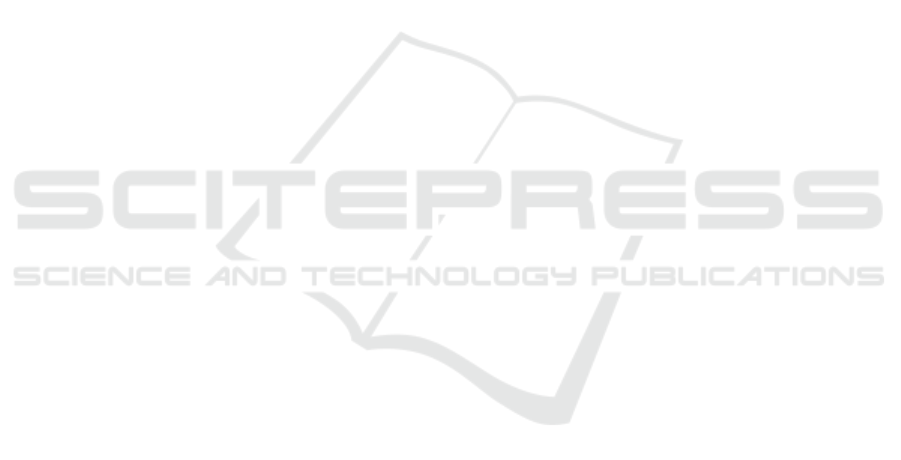
ing and Artificial Intelligence, Advances in Intelligent
and Soft Computing, Berlin, Heidelberg. Springer.
De Pessemier, T., Dooms, S., Deryckere, T., and Martens,
L. (2010). Time dependency of data quality for col-
laborative filtering algorithms. In Proceedings of
the fourth ACM conference on Recommender systems,
RecSys ’10, pages 281–284, New York, NY, USA. As-
sociation for Computing Machinery.
Fang, H., Zhang, D., Shu, Y., and Guo, G. (2020).
Deep Learning for Sequential Recommendation: Al-
gorithms, Influential Factors, and Evaluations. arXiv:
1905.01997.
Han, D., Li, J., Li, W., Liu, R., and Chen, H. (2019). An app
usage recommender system: improving prediction ac-
curacy for both warm and cold start users. Multimedia
Systems, 25(6):603–616.
Ishwarya, M. V., Swetha, G., Saptha Maaleekaa, S., and
Anu Grahaa, R. (2019). Efficient Recommender Sys-
tem by Implicit Emotion Prediction. In Advances in
Big Data and Cloud Computing, Advances in Intelli-
gent Systems and Computing, pages 173–178.
Jannach, D., Manzoor, A., Cai, W., and Chen, L. (2020).
A Survey on Conversational Recommender Systems.
arXiv:2004.00646 [cs]. arXiv: 2004.00646.
Jannach, D. and Zanker, M. (2020). Interactive and
Context-Aware Systems in Tourism. In Handbook
of e-Tourism, pages 1–22. Springer International Pub-
lishing, Cham.
Jin, Y., Tintarev, N., Htun, N. N., and Verbert, K. (2020).
Effects of personal characteristics in control-oriented
user interfaces for music recommender systems. User
Modeling and User-Adapted Interaction, 30(2):199–
249.
Kang, J., Condiff, K., Chang, S., Konstan, J. A., Terveen,
L., and Harper, F. M. (2017). Understanding How
People Use Natural Language to Ask for Recommen-
dations. In Proceedings of the Eleventh ACM Confer-
ence on Recommender Systems, pages 229–237, New
York, USA. ACM.
Lavanya, R., Khokle, T., and Maity, A. (2021). Review on
Hybrid Recommender System for Mobile Devices. In
Artificial Intelligence Techniques for Advanced Com-
puting Applications, pages 477–486, Singapore.
Lee, S. (2017). Improving Jaccard Index for Measuring
Similarity in Collaborative Filtering. In Information
Science and Applications 2017.
Li, B.-H., Zhang, A.-M., Zheng, W., Wan, S., Qin,
X.-L., Li, X., and Yin, H.-L. (2018). GRIP: A
Group Recommender Based on Interactive Preference
Model. Journal of Computer Science and Technology,
33(5):1039–1055.
Mahmood, T., Ricci, F., Venturini, A., and H
¨
opken, W.
(2008). Adaptive Recommender Systems for Travel
Planning. In Information and Communication Tech-
nologies in Tourism, pages 1–11, Vienna, Austria.
Mendoza, M. and Torres, N. (2020). Evaluating content
novelty in recommender systems. Journal of Intelli-
gent Information Systems, 54(2):297–316.
Mimoun, M. S. B., Poncin, I., and Garnier, M. (2017). Vir-
tual Sales Agents: The Reasons of Failure. In The
Customer is NOT Always Right? Marketing Orienta-
tionsin a Dynamic Business World.
Naiseh, M., Jiang, N., Ma, J., and Ali, R. (2020). Explain-
able Recommendations in Intelligent Systems: Deliv-
ery Methods, Modalities and Risks. In Research Chal-
lenges in Information Science, Lecture Notes in Busi-
ness Information Processing, Cham. Springer Interna-
tional Publishing.
Nalmpantis, O. and Tjortjis, C. (2017). The 50/50 Rec-
ommender: A Method Incorporating Personality into
Movie Recommender Systems. In Engineering Appli-
cations of Neural Networks, pages 498–507. Springer
International Publishing.
Narang, K., Song, Y., Schwing, A., and Sundaram, H.
(2021). FuseRec: fusing user and item homophily
modeling with temporal recommender systems. Data
Mining and Knowledge Discovery, 35(3):837–862.
Narducci, F., de Gemmis, M., Lops, P., and Semeraro, G.
(2018). Improving the User Experience with a Con-
versational Recommender System. In AI*IA 2018 –
Advances in Artificial Intelligence, pages 528–538.
Springer International Publishing.
Prasad, B. (2005). HYREC: A Hybrid Recommendation
System for E-Commerce. In Mu
˜
noz-
´
Avila, H. and
Ricci, F., editors, Case-Based Reasoning Research
and Development, Lecture Notes in Computer Sci-
ence, pages 408–420, Berlin, Heidelberg. Springer.
Ramnani, R. R., Sengupta, S., Ravilla, T. R., and Patil, S. G.
(2018). Smart entertainment - A critiquing based di-
alog system for eliciting user preferences and making
recommendations. In 23rd International Conference
on Applications of Natural Language to Information
Systems, Paris, France, volume 10859, pages 456–
463.
Sanchez, O. R., Torre, I., He, Y., and Knijnenburg, B. P.
(2020). A recommendation approach for user privacy
preferences in the fitness domain. User Modeling and
User-Adapted Interaction, 30(3):513–565.
Sidana, S., Trofimov, M., Horodnytskyi, O., Laclau, C.,
Maximov, Y., and Amini, M.-R. (2021). User prefer-
ence and embedding learning with implicit feedback
for recommender systems. Data Mining and Knowl-
edge Discovery, 35(2):568–592.
Tian, X., Hao, Y., Zhao, P., Wang, D., Liu, Y., and
Sheng, V. S. (2021). Considering Interaction Se-
quence of Historical Items for Conversational Recom-
mender System. In Database Systems for Advanced
Applications, pages 115–131. Springer International
Publishing.
U. S. Food & Drug Administration (2021). Drugs@FDA:
FDA-Approved Drugs.
Vajjhala, N. R., Rakshit, S., Oshogbunu, M., and Salisu, S.
(2021). Novel User Preference Recommender System
Based on Twitter Profile Analysis. In Soft Computing
Techniques and Applications, Advances in Intelligent
Systems and Computing, pages 85–93. Springer.
Ziegler, C.-N. (2005). Semantic Web Recommender Sys-
tems. In Current Trends in Database Technology -
EDBT 2004 Workshops, Lecture Notes in Computer
Science, pages 78–89, Berlin, Heidelberg. Springer.
WEBIST 2021 - 17th International Conference on Web Information Systems and Technologies
290