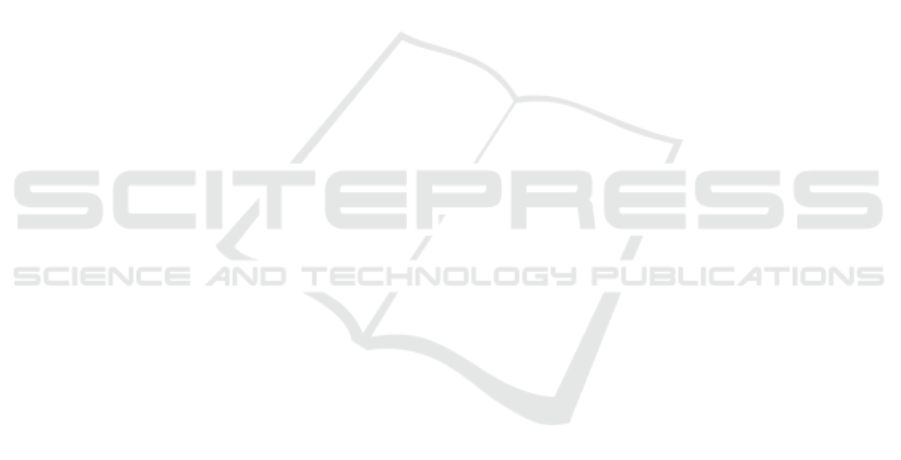
translation without a PnP process. What’s more, we
propose a novel pose refinement algorithm Contour-
Align by aligning the mask contour and the 2D pro-
jection contour for the single RGB image. This refine-
ment technique can be applied to most of the post-
processing of RBG based 6D estimation. Further-
more, the evaluation shows our work surpasses cur-
rent state-of-the-art methods. Therefore, our work is
encouraging because it indicates that it is feasible to
accurately predict the 6D pose object pose in a clut-
tered environment using RGB data only. An interest-
ing future work is to improve the estimation accuracy
when the CAD model is unavailable.
ACKNOWLEDGEMENTS
This work was supported by EPSRC Grant
No.EP/R026084/1, Robotics and Artificial Intel-
ligence for Nuclear (RAIN), UK.
REFERENCES
Abadi, M., Barham, P., Chen, J., Chen, Z., Davis, A.,
Dean, J., Devin, M., Ghemawat, S., Irving, G., Isard,
M., et al. (2016). Tensorflow: A system for large-
scale machine learning. In 12th {USENIX} sympo-
sium on operating systems design and implementation
({OSDI} 16), pages 265–283.
Brachmann, E., Krull, A., Michel, F., Gumhold, S., Shot-
ton, J., and Rother, C. (2014). Learning 6d object
pose estimation using 3d object coordinates. In Euro-
pean conference on computer vision, pages 536–551.
Springer.
Brachmann, E., Michel, F., Krull, A., Ying Yang, M.,
Gumhold, S., et al. (2016). Uncertainty-driven 6d
pose estimation of objects and scenes from a single
rgb image. In Proceedings of the IEEE conference on
computer vision and pattern recognition, pages 3364–
3372.
Do, T.-T., Cai, M., Pham, T., and Reid, I. (2018). Deep-
6dpose: Recovering 6d object pose from a single rgb
image. arXiv preprint arXiv:1802.10367.
He, K., Gkioxari, G., Doll
´
ar, P., and Girshick, R. (2017).
Mask r-cnn. In Proceedings of the IEEE international
conference on computer vision, pages 2961–2969.
He, K., Zhang, X., Ren, S., and Sun, J. (2016). Deep resid-
ual learning for image recognition. In Proceedings of
the IEEE conference on computer vision and pattern
recognition, pages 770–778.
Hinterstoisser, S., Cagniart, C., Ilic, S., Sturm, P., Navab,
N., Fua, P., and Lepetit, V. (2011). Gradient response
maps for real-time detection of textureless objects.
IEEE transactions on pattern analysis and machine
intelligence, 34(5):876–888.
Hinterstoisser, S., Lepetit, V., Ilic, S., Holzer, S., Bradski,
G., Konolige, K., and Navab, N. (2012). Model based
training, detection and pose estimation of texture-less
3d objects in heavily cluttered scenes. In Asian con-
ference on computer vision, pages 548–562. Springer.
Hodan, T., Barath, D., and Matas, J. (2020). Epos: estimat-
ing 6d pose of objects with symmetries. In Proceed-
ings of the IEEE/CVF conference on computer vision
and pattern recognition, pages 11703–11712.
Hu, Y., Fua, P., Wang, W., and Salzmann, M. (2020).
Single-stage 6d object pose estimation. In Proceed-
ings of the IEEE/CVF Conference on Computer Vision
and Pattern Recognition, pages 2930–2939.
Hu, Y., Hugonot, J., Fua, P., and Salzmann, M. (2019).
Segmentation-driven 6d object pose estimation. In
Proceedings of the IEEE Conference on Computer Vi-
sion and Pattern Recognition, pages 3385–3394.
Jafari, O. H., Mustikovela, S. K., Pertsch, K., Brachmann,
E., and Rother, C. (2018). ipose: instance-aware
6d pose estimation of partly occluded objects. In
Asian Conference on Computer Vision, pages 477–
492. Springer.
Kehl, W., Manhardt, F., Tombari, F., Ilic, S., and Navab, N.
(2017). Ssd-6d: Making rgb-based 3d detection and
6d pose estimation great again. In Proceedings of the
IEEE International Conference on Computer Vision,
pages 1521–1529.
Kendall, A. and Cipolla, R. (2017). Geometric loss func-
tions for camera pose regression with deep learning.
In Proceedings of the IEEE Conference on Computer
Vision and Pattern Recognition, pages 5974–5983.
Krull, A., Brachmann, E., Nowozin, S., Michel, F., Shot-
ton, J., and Rother, C. (2017). Poseagent: Budget-
constrained 6d object pose estimation via reinforce-
ment learning. In Proceedings of the IEEE Conference
on Computer Vision and Pattern Recognition, pages
6702–6710.
Lepetit, V., Moreno-Noguer, F., and Fua, P. (2009). Epnp:
An accurate o (n) solution to the pnp problem. Inter-
national journal of computer vision, 81(2):155.
Li, Y., Wang, G., Ji, X., Xiang, Y., and Fox, D. (2018).
Deepim: Deep iterative matching for 6d pose estima-
tion. In Proceedings of the European Conference on
Computer Vision (ECCV), pages 683–698.
Lowe, D. G. (2004). Distinctive image features from scale-
invariant keypoints. International journal of computer
vision, 60(2):91–110.
Manhardt, F., Kehl, W., Navab, N., and Tombari, F. (2018).
Deep model-based 6d pose refinement in rgb. In Pro-
ceedings of the European Conference on Computer Vi-
sion (ECCV), pages 800–815.
Michel, F., Kirillov, A., Brachmann, E., Krull, A.,
Gumhold, S., Savchynskyy, B., and Rother, C. (2017).
Global hypothesis generation for 6d object pose esti-
mation. In Proceedings of the IEEE Conference on
Computer Vision and Pattern Recognition, pages 462–
471.
Oberweger, M., Rad, M., and Lepetit, V. (2018). Making
deep heatmaps robust to partial occlusions for 3d ob-
ject pose estimation. In Proceedings of the European
ROBOVIS 2021 - 2nd International Conference on Robotics, Computer Vision and Intelligent Systems
38