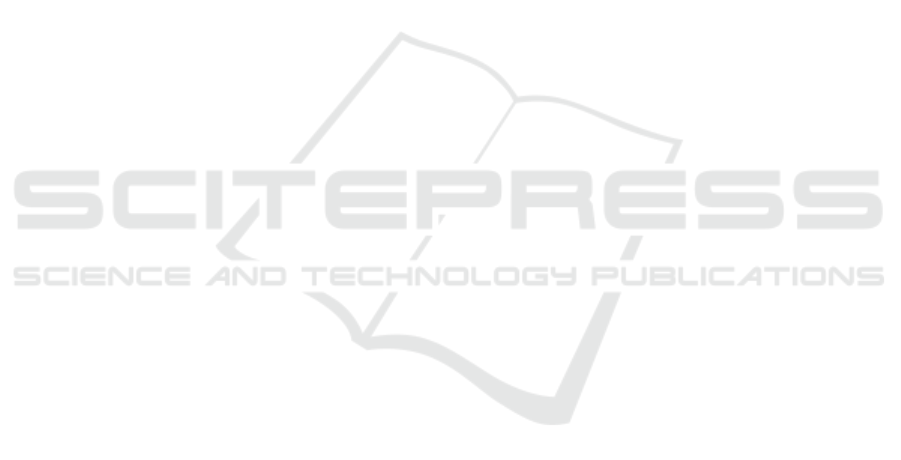
the OBS metric for different penetration rates of
CAVs. The model’s performance is only reported for
the long-term prediction since such samples have the
highest performance drop in the egocentric percep-
tion model based on the aforementioned experiment.
To prepare the data, we model the cooperative per-
ception mode by assuming 1%, 5%, 10%, 20%, and
50% percentages of CAVs. Figure 6 and Figure 5
show the relation between percentage of CAVs and
OBS metric, and percentage of CAVs and accuracy,
respectively. Both figures demonstrate a logarithmic
increase in performance and observability with in-
creasing the penetration rate. With 20% penetration
of CAVs on average, 85% of TV’s surrounding be-
come observable and the drop in performance com-
pared to full observability mode is decreased to 1%.
7 CONCLUSION
In this paper, we proposed two perception models, ap-
plicable to any vehicle trajectory dataset recorded by
a top-down view sensor, to model the egocentric and
cooperative perception. Then, a comparative study
has been performed to quantify the impact of each
perception mode on the problem of lane change pre-
diction. The results showed a 4% performance drop in
our long-term LC prediction model (i.e., T
delay
= 4s)
when using ego-centric perception instead of the full-
observable original dataset. Also, the results indi-
cated that cooperative perception with 20% penetra-
tion of CAVs can almost compensate for the perfor-
mance drop of our prediction model related to ego-
centric perception limitation.
Future work should consider extending the data
generation method by considering the errors in object
detection and tracking modules. The binary represen-
tation used in this work can be extended to a proba-
bilistic representation which enables encoding the er-
ror and uncertainty in vehicles states estimation. Fur-
thermore, the 2D occlusion model used in this study
can be extended to a 3D occlusion model to decrease
the modelling error.
ACKNOWLEDGEMENTS
This work was supported by Jaguar Land Rover and
the U.K.-EPSRC as part of the jointly funded Towards
Autonomy: Smart and Connected Control (TASCC)
Programme under Grant EP/N01300X/1. We would
like to thank Omar Al-Jarrah for reviewing previous
version of this paper.
REFERENCES
Chen, Q., Tang, S., Yang, Q., and Fu, S. (2019). Cooper:
Cooperative perception for connected autonomous ve-
hicles based on 3d point clouds. arXiv preprint
arXiv:1905.05265.
Colyar, J. and Halkias, J. (2007). Us highway 101 dataset.
Technical report, U.S. Department of Transportation,
Federal Highway Administration (FHWA).
Deo, N. and Trivedi, M. M. (2018). Convolutional social
pooling for vehicle trajectory prediction. In Proceed-
ings of the IEEE Conference on Computer Vision and
Pattern Recognition (CVPR) Workshops.
Ding, W., Chen, J., and Shen, S. (2019). Predicting vehi-
cle behaviors over an extended horizon using behav-
ior interaction network. In 2019 International Con-
ference on Robotics and Automation (ICRA), pages
8634–8640.
Earnshaw, R. A. (1985). Fundamental Algorithms for Com-
puter Graphics: NATO Advanced Study Institute Di-
rected by JE Bresenham, RA Earnshaw, MLV Pitte-
way. Springer.
Fern
´
andez-Llorca, D., Biparva, M., Izquierdo-Gonzalo, R.,
and Tsotsos, J. K. (2020). Two-stream networks for
lane-change prediction of surrounding vehicles. In
2020 IEEE 23rd International Conference on Intelli-
gent Transportation Systems (ITSC), pages 1–6.
Gallitz, O., De Candido, O., Botsch, M., Melz, R., and
Utschick, W. (2020). Interpretable machine learning
structure for an early prediction of lane changes. In
Farka
ˇ
s, I., Masulli, P., and Wermter, S., editors, Artifi-
cial Neural Networks and Machine Learning – ICANN
2020, pages 337–349, Cham. Springer International
Publishing.
Halkias, J. and Colyar, J. (2007). Us highway 80 dataset.
Technical report, U.S. Department of Transportation,
Federal Highway Administration (FHWA).
Hu, Y., Zhan, W., and Tomizuka, M. (2018). Probabilis-
tic prediction of vehicle semantic intention and mo-
tion. In 2018 IEEE Intelligent Vehicles Symposium
(IV), pages 307–313.
Izquierdo, R., Quintanar, A., Parra, I., Fern
´
andez-Llorca,
D., and Sotelo, M. A. (2019a). Experimental val-
idation of lane-change intention prediction method-
ologies based on cnn and lstm. In 2019 IEEE In-
telligent Transportation Systems Conference (ITSC),
pages 3657–3662.
Izquierdo, R., Quintanar, A., Parra, I., Fern
´
andez-Llorca,
D., and Sotelo, M. A. (2019b). The prevention dataset:
a novel benchmark for prediction of vehicles inten-
tions. In 2019 IEEE Intelligent Transportation Sys-
tems Conference (ITSC), pages 3114–3121.
Kim, S., Liu, W., Ang, M. H., Frazzoli, E., and Rus, D.
(2015). The impact of cooperative perception on de-
cision making and planning of autonomous vehicles.
IEEE Intelligent Transportation Systems Magazine,
7(3):39–50.
Kingma, D. P. and Ba, J. (2017). Adam: A method for
stochastic optimization.
ROBOVIS 2021 - 2nd International Conference on Robotics, Computer Vision and Intelligent Systems
120