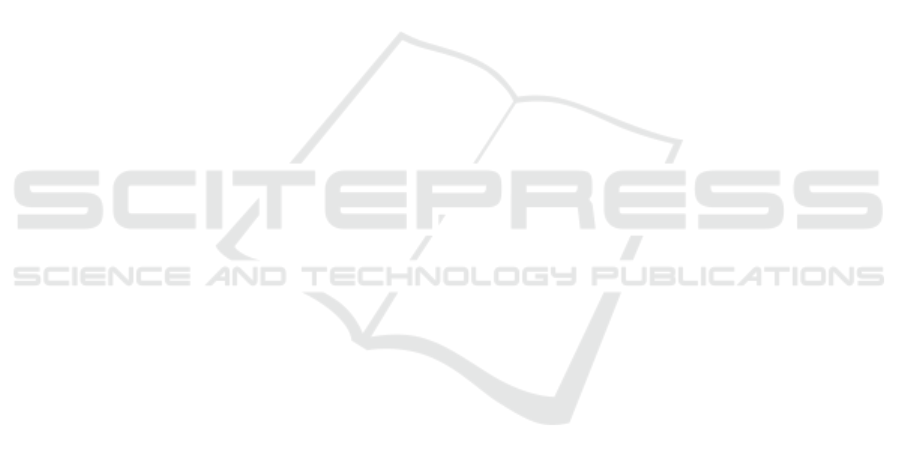
ble 2). Using a hockey goalie dataset, we show that
POOF can improve performance with a very small
amount of labels. We also show POOF can achieve
significantly improved results over using pretrained
weights across various accuracy thresholds. Further-
more, we showed this performance improvement is
achieved across most individual joints and also sug-
gested multiple directions for future research. Over-
all, this research should significantly reduce the time
required for annotating pose data across different do-
mains without compromising model accuracy and al-
low pose estimation to be more easily applied to a
wide variety of domains.
ACKNOWLEDGEMENTS
This research is supported in part by grants from MI-
TACs and Stathletes, Inc.
REFERENCES
Bertasius, G., Feichtenhofer, C., Tran, D., Shi, J., and Tor-
resani, L. (2019). Learning temporal pose estimation
from sparsely-labeled videos. arXiv, (NeurIPS):1–12.
Butler, D. J., Wulff, J., Stanley, G. B., and Black, M. J.
(2012). A naturalistic open source movie for optical
flow evaluation. In European Conference on Com-
puter Vision, pages 611–625. Springer.
Charles, J., Pfister, T., Magee, D., Hogg, D., and Zisserman,
A. (2016). Personalizing human video pose estima-
tion. In Proceedings of the IEEE Conference on Com-
puter Vision and Pattern Recognition, pages 3063–
3072.
Doersch, C. and Zisserman, A. (2019). Sim2real transfer
learning for 3d human pose estimation: motion to the
rescue. arXiv preprint arXiv:1907.02499.
Hinterstoisser, S., Pauly, O., Heibel, H., Marek, M., and
Bokeloh, M. (2019). An annotation saved is an anno-
tation earned: Using fully synthetic training for object
instance detection. arXiv preprint arXiv:1902.09967.
Li, W., Wang, Z., Yin, B., Peng, Q., Du, Y., Xiao, T., Yu,
G., Lu, H., Wei, Y., and Sun, J. (2019). Rethinking
on multi-stage networks for human pose estimation.
arXiv preprint arXiv:1901.00148.
Lin, T.-Y., Maire, M., Belongie, S., Hays, J., Perona, P.,
Ramanan, D., Doll
´
ar, P., and Zitnick, C. L. (2014).
Microsoft coco: Common objects in context. In Euro-
pean Conference on Computer Vision, pages 740–755.
Springer.
Neverova, N., Thewlis, J., Guler, R. A., Kokkinos, I., and
Vedaldi, A. (2019). Slim densepose: Thrifty learning
from sparse annotations and motion cues. In Proceed-
ings of the IEEE/CVF Conference on Computer Vision
and Pattern Recognition, pages 10915–10923.
Newell, A., Yang, K., and Deng, J. (2016). Stacked hour-
glass networks for human pose estimation. In Euro-
pean Conference on Computer Vision, pages 483–499.
Springer.
Pfister, T., Charles, J., and Zisserman, A. (2015). Flow-
ing convnets for human pose estimation in videos. In
Proceedings of the IEEE international Conference on
Computer Vision, pages 1913–1921.
Romero, J., Loper, M., and Black, M. J. (2015). Flow-
cap: 2d human pose from optical flow. In German
Conference on Pattern Recognition, pages 412–423.
Springer.
Teed, Z. and Deng, J. (2020). Raft: Recurrent all-pairs field
transforms for optical flow. In European Conference
on Computer Vision, pages 402–419. Springer.
Zhang, D., Guo, G., Huang, D., and Han, J. (2018). Pose-
flow: A deep motion representation for understand-
ing human behaviors in videos. In Proceedings of
the IEEE Conference on Computer Vision and Pattern
Recognition, pages 6762–6770.
icSPORTS 2021 - 9th International Conference on Sport Sciences Research and Technology Support
122