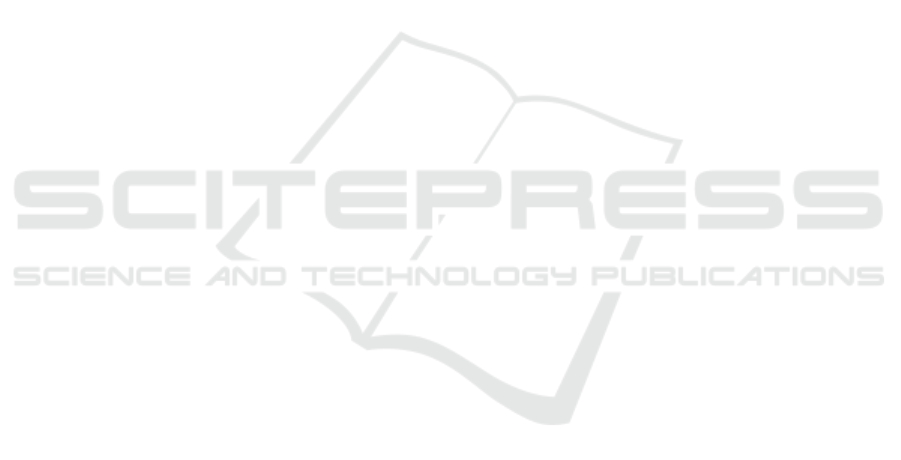
Isensee, F., Petersen, J., Klein, A., Zimmerer, D., Jaeger,
P. F., Kohl, S., Wasserthal, J., Koehler, G., Norajitra,
T., Wirkert, S. J., and Maier-Hein, K. H. (2018). nnu-
net: Self-adapting framework for u-net-based medical
image segmentation. CoRR, abs/1809.10486.
Jia, J. (2020). A package to compute segmentation metrics:
seg-metrics.
Lou, A., Guan, S., and Loew, M. H. (2021). DC-UNet: re-
thinking the u-net architecture with dual channel effi-
cient CNN for medical image segmentation. In Land-
man, B. A. and I
ˇ
sgum, I., editors, Medical Imaging
2021: Image Processing. SPIE.
Menze, B. H., Jakab, A., Bauer, S., Kalpathy-Cramer, J.,
Farahani, K., Kirby, J., Burren, Y., Porz, N., Slot-
boom, J., Wiest, R., Lanczi, L., Gerstner, E., We-
ber, M.-A., Arbel, T., Avants, B. B., Ayache, N.,
Buendia, P., Collins, D. L., Cordier, N., Corso, J. J.,
Criminisi, A., Das, T., Delingette, H., Demiralp, C.,
Durst, C. R., Dojat, M., Doyle, S., Festa, J., Forbes,
F., Geremia, E., Glocker, B., Golland, P., Guo, X.,
Hamamci, A., Iftekharuddin, K. M., Jena, R., John,
N. M., Konukoglu, E., Lashkari, D., Mariz, J. A.,
Meier, R., Pereira, S., Precup, D., Price, S. J., Ra-
viv, T. R., Reza, S. M. S., Ryan, M., Sarikaya, D.,
Schwartz, L., Shin, H.-C., Shotton, J., Silva, C. A.,
Sousa, N., Subbanna, N. K., Szekely, G., Taylor, T. J.,
Thomas, O. M., Tustison, N. J., Unal, G., Vasseur, F.,
Wintermark, M., Ye, D. H., Zhao, L., Zhao, B., Zi-
kic, D., Prastawa, M., Reyes, M., and Leemput, K. V.
(2015). The multimodal brain tumor image segmen-
tation benchmark (BRATS). IEEE Transactions on
Medical Imaging, 34(10):1993–2024.
Milletari, F., Navab, N., and Ahmadi, S. (2016). V-net:
Fully convolutional neural networks for volumetric
medical image segmentation. CoRR, abs/1606.04797.
Myronenko, A. (2018). 3d MRI brain tumor segmen-
tation using autoencoder regularization. CoRR,
abs/1810.11654.
Oktay, O., Schlemper, J., Folgoc, L. L., Lee, M. C. H., Hein-
rich, M. P., Misawa, K., Mori, K., McDonagh, S. G.,
Hammerla, N. Y., Kainz, B., Glocker, B., and Rueck-
ert, D. (2018). Attention u-net: Learning where to
look for the pancreas. CoRR, abs/1804.03999.
Paszke, A., Gross, S., Massa, F., Lerer, A., Bradbury, J.,
Chanan, G., Killeen, T., Lin, Z., Gimelshein, N.,
Antiga, L., Desmaison, A., K
¨
opf, A., Yang, E., De-
Vito, Z., Raison, M., Tejani, A., Chilamkurthy, S.,
Steiner, B., Fang, L., Bai, J., and Chintala, S. (2019).
Pytorch: An imperative style, high-performance deep
learning library. CoRR, abs/1912.01703.
Ronneberger, O., Fischer, P., and Brox, T. (2015). U-net:
Convolutional networks for biomedical image seg-
mentation. CoRR, abs/1505.04597.
Sinha, A. and Dolz, J. (2019). Multi-scale guided at-
tention for medical image segmentation. CoRR,
abs/1906.02849.
Tamimi, A. F. and Juweid, M. (2017). Epidemiology and
outcome of glioblastoma. In Glioblastoma, pages
143–153. Codon Publications.
Tustison, N. J., Avants, B. B., Cook, P. A., Zheng, Y., Egan,
A., Yushkevich, P. A., and Gee, J. C. (2010). N4itk:
Improved n3 bias correction. IEEE Transactions on
Medical Imaging, 29(6):1310–1320.
Zhou, C., Ding, C., Wang, X., Lu, Z., and Tao, D.
(2019). One-pass multi-task networks with cross-task
guided attention for brain tumor segmentation. CoRR,
abs/1906.01796.
Zhou, Z., Siddiquee, M. M. R., Tajbakhsh, N., and Liang,
J. (2018). Unet++: A nested u-net architecture for
medical image segmentation. CoRR, abs/1807.10165.
Coordinate Attention UNet
127