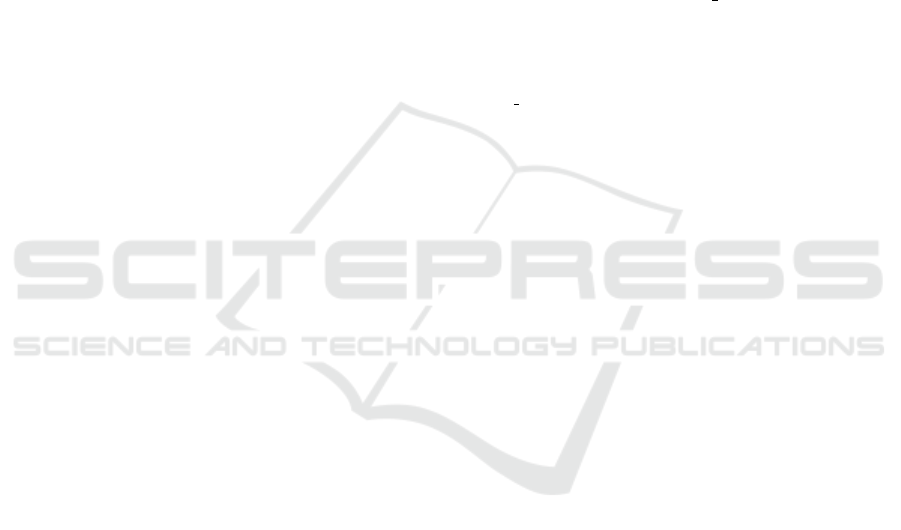
havior from china. (august 2019). https://about.fb.
com/news/2019/08/removing-cib-china/.
Grace, L. and Hone, B. (2019). Factitious: Large scale com-
puter game to fight fake news and improve news liter-
acy. In Extended Abstracts of the 2019 CHI Confer-
ence on Human Factors in Computing Systems, pages
1–8.
Hughes, T., Smith, J., and Leavitt, A. (2018). Helping peo-
ple better assess the stories they see in news feed with
the context button. (2018). https://about.fb.com/news/
2018/04/news-feed-fyi-more-context/.
Khan, M. L. (2017). Social media engagement: What moti-
vates user participation and consumption on youtube?
Computers in Human Behavior, 66(4):236–247.
Kumar, S. and Shah, N. (2018). False information on
web and social media: A survey. arXiv preprint
arXiv:1804.08559.
Lewandowsky, S., Ecker, U. K., and Cook, J. (2017). Be-
yond misinformation: Understanding and coping with
the “post-truth” era. Journal of applied research in
memory and cognition, 6(4):353–369.
Lewandowsky, S., Ecker, U. K., Seifert, C. M., Schwarz,
N., and Cook, J. (2012). Misinformation and its
correction: Continued influence and successful debi-
asing. Psychological science in the public interest,
13(3):106–131.
Marwick, A. E. (2018). Why do people share fake news?
a sociotechnical model of media effects. Georgetown
Law Technology Review, 2(2):474–512.
Mele, N., Lazer, D., Baum, M., Grinberg, N., Friedland,
L., Joseph, K., Hobbs, W., and Mattsson, C. (2017).
Combating fake news: An agenda for research and ac-
tion. Retrieved on October, 17:2018.
Morris, M. R., Counts, S., Roseway, A., Hoff, A., and
Schwarz, J. (2012). Tweeting is believing? under-
standing microblog credibility perceptions. In Pro-
ceedings of the ACM 2012 conference on computer
supported cooperative work, pages 441–450.
Nekmat, E. (2020). Nudge effect of fact-check alerts:
Source influence and media skepticism on sharing of
news misinformation in social media. Social Media+
Society, 6(1):2056305119897322.
Nonnecke, B. and Preece, J. (1999). Shedding light on lurk-
ers in online communities. Ethnographic Studies in
Real and Virtual Environments: Inhabited Informa-
tion Spaces and Connected Communities, Edinburgh,
123128.
Pennycook, G., McPhetres, J., Zhang, Y., Lu, J. G., and
Rand, D. G. (2020). Fighting covid-19 misinforma-
tion on social media: Experimental evidence for a
scalable accuracy-nudge intervention. Psychological
science, 31(7):770–780.
Perez, E. B., King, J., Watanabe, Y. H., and Chen, X.
(2020). Counterweight: Diversifying news consump-
tion. In Adjunct Publication of the 33rd Annual ACM
Symposium on User Interface Software and Technol-
ogy, pages 132–134.
Preece, J. and Shneiderman, B. (2009). The reader-to-leader
framework: Motivating technology-mediated social
participation. AIS transactions on human-computer
interaction, 1(1):13–32.
Roozenbeek, J. and van der Linden, S. (2019). Fake news
game confers psychological resistance against online
misinformation. Palgrave Communications, 5(1):1–
10.
Rosen, G. (2021). How we’re tackling misin-
formation across our apps (2021). https:
//about.fb.com/news/2021/03/how-were-tackling-
misinformation-across-our-apps/.
Rosen, G. and Lyons, T. (2019). Remove, reduce, inform:
New steps to manage problematic content. (april
2019). https://about.fb.com/news/2019/04/remove-
reduce-inform-new-steps/.
Roth, Y. and Harvey, D. (2018). How twitter is fight-
ing spam and malicious automation. (june 2018).
https://blog.twitter.com/en\ us/topics/company/
2018/how-twitter-is-fighting-spam-and-malicious-
automation.html.
Roth, Y. and Pickles, N. (2020). Updating our approach to
misleading information. https://blog.twitter.com/en\
us/topics/product/2020/updating-our-approach-to-
misleading-information.html.
Shao, G. (2009). Understanding the appeal of user-
generated media: a uses and gratification perspective.
Internet research, 19(1):7–25.
Smith, J. (2017). Designing against misinformation. (de-
cember 2017). https://medium.com/facebook-design/
designing-against-misinformation-e5846b3aa1e2.
Starbird, K. (2017). Examining the alternative media
ecosystem through the production of alternative nar-
ratives of mass shooting events on twitter. In Eleventh
International AAAI Conference on Web and Social
Media.
Starbird, K., Arif, A., Wilson, T., Van Koevering, K., Yefi-
mova, K., and Scarnecchia, D. (2018). Ecosystem or
echo-system? exploring content sharing across alter-
native media domains. In Proceedings of the Inter-
national AAAI Conference on Web and Social Media,
volume 12.
Su, S. (2017). New test with related articles (2017).
https://about.fb.com/news/2017/04/news-feed-fyi-
new-test-with-related-articles/.
Thaler, R. H. and Sunstein, C. R. (2009). Nudge: Improving
decisions about health, wealth, and happiness. Pen-
guin.
Torres, R., Gerhart, N., and Negahban, A. (2018). Combat-
ing fake news: An investigation of information veri-
fication behaviors on social networking sites. In Pro-
ceedings of the 51st Hawaii international conference
on system sciences.
Trifiro, B. M. and Gerson, J. (2019). Social media usage
patterns: Research note regarding the lack of universal
validated measures for active and passive use. Social
Media+ Society, 5(2):2056305119848743.
Reframing the Fake News Problem: Social Media Interaction Design to Make the Truth Louder
165