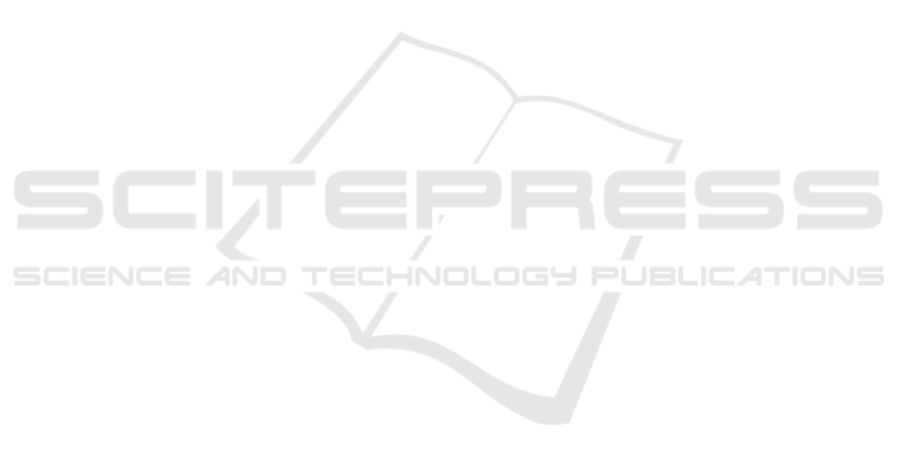
glish Premier League (EPL), Bundesliga, or LaLiga
should be cautiously considered. As a future work, we
plan to evaluate our proposed model to these leagues.
Second, we only considered eleven players in the
starting lineup, which has the rooms for improvement.
Despite the limitations, we believe our experimental
design and results can provide important insights for
both football industry and academic researchers who
want to lighten important characteristics of winning
teams.
ACKNOWLEDGEMENTS
This research was supported by the framework
of international cooperation program managed by
the National Research Foundation of Korea (NRF-
2020K2A9A2A11103842), and the MSIT(Ministry
of Science and ICT), Korea, under the ICT Creative
Consilience program (IITP-2021-2020-0-01821) su-
pervised by the IITP (Institute for Information &
communications Technology Planning & Evaluation).
REFERENCES
Bastida Castillo, A., G
´
omez Carmona, C. D., De la
Cruz S
´
anchez, E., and Pino Ortega, J. (2018). Ac-
curacy, intra-and inter-unit reliability, and comparison
between gps and uwb-based position-tracking systems
used for time–motion analyses in soccer. European
journal of sport science, 18(4):450–457.
Bavelas, A. (1950). Communication patterns in task-
oriented groups. The journal of the acoustical society
of America, 22(6):725–730.
Breiman, L. (2001). Random forests. Machine learning,
45(1):5–32.
Chen, T. and Guestrin, C. (2016). Xgboost: A scalable
tree boosting system. In Proceedings of the 22nd acm
sigkdd international conference on knowledge discov-
ery and data mining, pages 785–794.
Clemente, F. M., Martins, F. M. L., Kalamaras, D., Wong,
P. D., and Mendes, R. S. (2015). General network
analysis of national soccer teams in fifa world cup
2014. International Journal of Performance Analysis
in Sport, 15(1):80–96.
Freeman, L. C. (1977). A set of measures of centrality based
on betweenness. Sociometry, pages 35–41.
Georgievski, B., Labadze, L., and Aboelsoud, M. E. (2019).
Comparative advantage as a success factor in football
clubs: Evidence from the english premier league (epl).
Journal of Human Sport and Exercise.
Gunn, S. R. et al. (1998). Support vector machines for
classification and regression. ISIS technical report,
14(1):5–16.
Harrop, K. and Nevill, A. (2014). Performance indicators
that predict success in an english professional league
one soccer team. International Journal of Perfor-
mance Analysis in Sport, 14(3):907–920.
Hassan, A., Akl, A.-R., Hassan, I., and Sunderland, C.
(2020). Predicting wins, losses and attributes’ sen-
sitivities in the soccer world cup 2018 using neural
network analysis. Sensors, 20(11):3213.
Johansen, H. D., Pettersen, S. A., Halvorsen, P., and Jo-
hansen, D. (2013). Combining video and player
telemetry for evidence-based decisions in soccer. In
icSPORTS, pages 197–205.
Linke, D., Link, D., and Lames, M. (2020). Football-
specific validity of tracab’s optical video tracking sys-
tems. PloS one, 15(3):e0230179.
Pettersson, D. and Nyquist, R. (2017). Football match pre-
diction using deep learning. Psychology of Sport and
Exercise, 15(5):538–547.
Pons, E., Garc
´
ıa-Calvo, T., Resta, R., Blanco, H., L
´
opez del
Campo, R., D
´
ıaz Garc
´
ıa, J., and Pulido, J. J. (2019). A
comparison of a gps device and a multi-camera video
technology during official soccer matches: Agreement
between systems. PloS one, 14(8):e0220729.
Razali, N., Mustapha, A., Yatim, F. A., and Ab Aziz,
R. (2017). Predicting football matches results using
bayesian networks for english premier league (epl).
In Iop conference series: Materials science and en-
gineering, volume 226, page 012099. IOP Publishing.
Tang, X., Wang, J., Zhong, J., and Pan, Y. (2013). Pre-
dicting essential proteins based on weighted degree
centrality. IEEE/ACM Transactions on Computational
Biology and Bioinformatics, 11(2):407–418.
Passing to Win: Using Characteristics of Passing Information for Match Winner Prediction
59