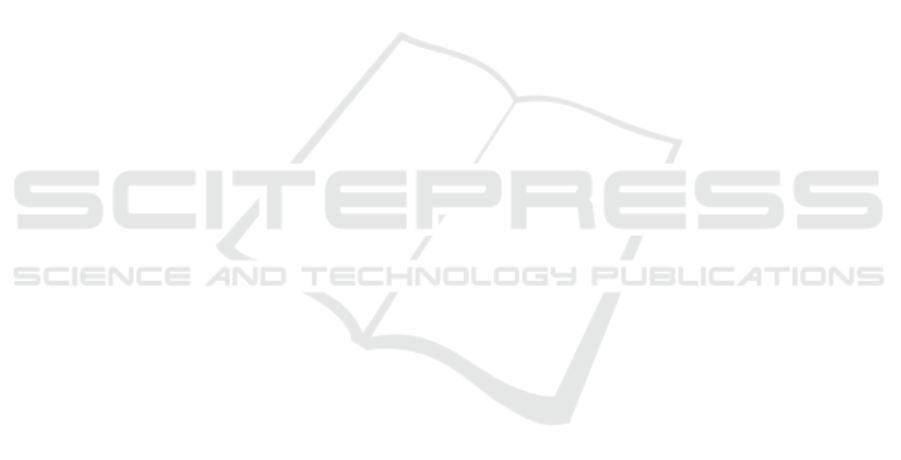
aspects that can be understood from texts in the spe-
cific case of the mining industry (Pons et al., 2021),
and finally the study on discourse analysis over time
in the extractive industry, where a text analytics per-
spective is developed on the specific field under the
same scope of this paper, but use a text analysis tool
that does not take into account corpora (WordStat).
8 CONCLUSIONS
This study has shown that a methodology for text
indexing and classification, based in turn on a solid
technique of clustering, adapted to guarantee some
relevant properties, that are very desirable in a con-
text of usage of document archives as tools for better
understanding the social reality, can be used to pre-
dict fundamental properties of fairness, transparency
and applicability of the contracts themselves. In par-
ticular, for this context it would be very interesting to
develop techniques that are naturally evolving from
basic clustering methods, as we proposed in the study.
From a comparison viewpoint it will be interesting
to understand whether convolutional networks and
other machine learning methods could be fruitfully
employed, without quitting to critical sense (Vincent
and Ogier, 2019). We are now adapting the method
for other types of repositories. In particular we are
making use of the above mentioned approach onto so-
cial media, similarly to what has been proposed in (Li
et al., 2019).
Further we shall disclose other aspects to be veri-
fied by gold standard analysis, that could be contained
in documents, and in particular in contract texts, in-
cluding analysis of textual connections among named
entities as, in particular, persons and organisations.
Economists have long discussed the risk that a large
endowment in natural resources may turn into a curse
(Van Der Ploeg, 2011). This analysis has shown that
the balance of power between companies and states
may tilt into one direction, with detrimental effects
on fairness.
Similarly on how we did investigate here we also
aim at developing novel methods to identify text ana-
lytics methods that can be employed with the purpose
of compliance analysis, within the declarative frame-
work defined in (Olivieri et al., 2015).
REFERENCES
Aizawa, A. (2003). An information-theoretic perspective of
tf-idf measures. Information Processing and Manage-
ment, 39(1):45–65.
Ambrosino, A., Cedrini, M., Davis, J., Fiori, S., Guerzoni,
M., and Nuccio, M. (2018). What topic modeling
could reveal about the evolution of economics*. Jour-
nal of Economic Methodology, 25(4):329–348.
Basole, R., Park, H., and Chao, R. (2019). Visual anal-
ysis of venture similarity in entrepreneurial ecosys-
tems. IEEE Transactions on Engineering Manage-
ment, 66(4):568–582.
Eckhaus, E. and Sheaffer, Z. (2018). Managerial hubris
detection: the case of enron. Risk Management,
20(4):304–325.
Gupta, A., Dengre, V., Kheruwala, H., and Shah, M. (2020).
Comprehensive review of text-mining applications in
finance. Financial Innovation, 6(1).
Laxmi Lydia, E., Govindaswamy, P., Lakshmanaprabu,
S., and Ramya, D. (2018). Document clustering
based on text mining k-means algorithm using eu-
clidean distance similarity. Journal of Advanced Re-
search in Dynamical and Control Systems, 10(2 Spe-
cial Issue):208–214.
Lee, P., Kleinman, G., and Kuei, C.-H. (2020). Using text
analytics to apprehend urban sustainability develop-
ment. Sustainable Development, 28(4):897–921.
Li, D., Zhang, Y., and Li, C. (2019). Mining public opinion
on transportation systems based on social media data.
Sustainability (Switzerland), 11(15).
Olivieri, F., Cristani, M., and Governatori, G. (2015). Com-
pliant business processes with exclusive choices from
agent specification. Lecture Notes in Computer Sci-
ence (including subseries Lecture Notes in Artificial
Intelligence and Lecture Notes in Bioinformatics),
9387:603–612.
Pons, A., Vintr
`
o, C., Rius, J., and Vilaplana, J. (2021). Im-
pact of corporate social responsibility in mining in-
dustries. Resources Policy, 72.
Van Der Ploeg, F. (2011). Natural resources: Curse or bless-
ing? Journal of Economic Literature, 49(2):366–420.
Vincent, N. and Ogier, J.-M. (2019). Shall deep learning be
the mandatory future of document analysis problems?
Pattern Recognition, 86:281–289.
Wang, W., Chen, W., Zhu, K., and Wang, H. (2020). Em-
phasizing the entrepreneur or the idea? the impact
of text content emphasis on investment decisions in
crowdfunding. Decision Support Systems, 136.
Wei, L., Li, G., Zhu, X., Sun, X., and Li, J. (2019). Devel-
oping a hierarchical system for energy corporate risk
factors based on textual risk disclosures. Energy Eco-
nomics, 80:452–460.
Yuan, H., Lau, R., and Xu, W. (2016). The determinants
of crowdfunding success: A semantic text analytics
approach. Decision Support Systems, 91:67–76.
Text Analytics Can Predict Contract Fairness, Transparency and Applicability
323