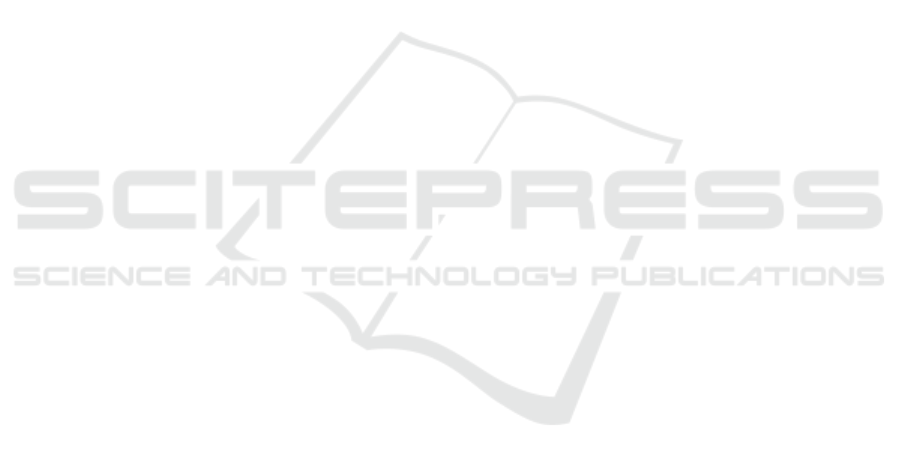
transform infrared spectroscopy (FTIR) tool as it is
a fast and cost-effective method, which allows early
detection of cancer-specific chemical changes in tis-
sues, cells and biofluids. In this research, it is pro-
posed to use this tool for diagnosis in pediatric acute
lymphoblastic leukemia using blood samples. As part
of the experiment, an evaluation has been performed
with 10 patients with this disease. Given this, the
authors developed a predictive model based on Ad-
aBoost with a percentage of 85% accuracy. In con-
trast to our approach, a technological model has been
developed focused on the diagnosis and treatment of
the four subtypes of pediatric leukemia through labo-
ratory results, symptoms, signs, and general medical
aspects of the patient. From which, an accuracy of
92.86% was obtained.
6 CONCLUSIONS
A machine learning model trained with a dataset in
a tabular manner with medical history, symptoms,
signs, and laboratory results has been developed to
be able to identify whether the patient has high prob-
ability in suffering from pediatric leukemia disease.
It has been shown that the multiclass boosted deci-
sion tree algorithm has a high percentage of accuracy
(92.86%) for obtaining a predictive result of sugges-
tion to support the diagnosis and treatment of pedi-
atric leukemia. In addition, there is evidence of an
opportunity to reduce the misdiagnosis results from
the solution, since a lower percentage of false posi-
tives (7.14%) was obtained.
An interesting future work can be the analysis
of information such as medical history (with ma-
chine learning), medical images (with computer vi-
sion) or adding new modules to complement and
increase knowledge and support the recovery rate
of pediatric cancer disease or its protection with
blockchain (Arroyo-Mari
˜
nos et al., 2021).
REFERENCES
Arroyo-Mari
˜
nos, J. C., Mejia-Valle, K. M., and Ugarte, W.
(2021). Technological model for the protection of ge-
netic information using blockchain technology in the
private health sector. In ICT4AWE.
Aslam, M., Xue, C., Chen, Y., Zhang, A., Liu, M., Wang,
K., and Cui, D. (2021). Breath analysis based early
gastric cancer classification from deep stacked sparse
autoencoder neural network. Scientific Reports, 11.
Bi, Q., Goodman, K., Kaminsky, J., and Lessler, J. (2019).
What is machine learning: a primer for the epidemiol-
ogist. American journal of epidemiology, 188.
Chaber, R., Kowal, A., Jakubczyk, P., Arthur, C., Łach,
K., Wojnarowska-Nowak, R., Kusz, K., Zawlik, I.,
Paszek, S., and Cebulski, J. (2021). A preliminary
study of ftir spectroscopy as a potential non-invasive
screening tool for pediatric precursor b lymphoblastic
leukemia. Molecules, 26.
Fathi, E., Jahangoshai Rezaee, M., Tavakkoli-Moghaddam,
R., Alizadeh, A., and Montazer, A. (2020). Design
of an integrated model for diagnosis and classification
of pediatric acute leukemia using machine learning.
Journal of Engineering in Medicine, 234.
Gonz
´
alez, E. E. M., Reyes-Ort
´
ız, J. A., and Gonz
´
alez-
Beltr
´
an, B. A. (2020). Machine learning models
for cancer type classification with unstructured data.
Computaci
´
on y Sistemas, 24(2).
Gonzalez, G., Lu, Z., Leaman, R., Weissenbacher, D.,
Boland, M. R., Chen, Y., Du, J., Fluck, J., Greene,
C. S., Holmes, J. H., Kashyap, A., Nielsen, R. L.,
Ouyang, Z., Schaaf, S., Taroni, J. N., Tao, C., Zhang,
Y., and Liu, H. (2019). Text mining and machine
learning for precision medicine. In PSB.
Hijiya, N. and Suttorp, M. (2019). How i treat chronic
myeloid leukemia in children and adolescents. Blood,
133.
Kaplan, J. (2019). Leukemia in children. Pediatrics in Re-
view, 40.
Marti-Bonmati, L., Alberich-Bayarri, A., Ladenstein, R.,
Blanquer, I., Segrelles, D., Cerd
´
a-Alberich, L., Gkon-
tra, P., Hero, B., Garcia Aznar, J. M., Keim,
D., Jentner, W., Seymour, K., Jim
´
enez-Pastor, A.,
Gonz
´
alez Valverde, I., Heras, B., Essiaf, S., Walker,
D., Rochette, M., Bubak, M., and Neri, E. (2020).
Primage project: predictive in silico multiscale ana-
lytics to support childhood cancer personalised evalu-
ation empowered by imaging biomarkers. European
Radiology Experimental, 4.
Mathew, S., Gulia, S., Singh, V., and Dev, V. (2017). A
review paper on cloud computing and its security con-
cerns. In RICE, volume 10.
Naymagon, L., Najfeld, V., Teruya-Feldstein, J., El Ja-
mal, S., and Mascarenhas, J. (2021). Acute Myeloid
Leukemia.
O’Brien, M., Seif, A., and Hunger, S. (2018). Acute lym-
phoblastic leukemia in children. Wintrobe’s Clinical
Hematology: Fourteenth Edition.
Rajagopal, S., S., H., and Kundapur, P. (2020). Performance
analysis of binary and multiclass models using azure
machine learning. International Journal of Electrical
and Computer Engineering (IJECE), 10.
Rodin, A., Gogoshin, G., Hilliard, S., Wang, L., Egelston,
C., Rockne, R., Chao, J., and Lee, P. (2021). Dis-
secting response to cancer immunotherapy by apply-
ing bayesian network analysis to flow cytometry data.
International Journal of Molecular Sciences, 22.
Verda, D., Parodi, S., Ferrari, E., and Muselli, M. (2019).
Analyzing gene expression data for pediatric and adult
cancer diagnosis using logic learning machine and
standard supervised methods. BMC Bioinform., 20(9).
Zhao, Y., Chang, C., Hannum, M., Lee, J., and Shen, R.
(2021). Bayesian network-driven clustering analy-
sis with feature selection for high-dimensional multi-
modal molecular data. Scientific Reports, 11.
Technological Model using Machine Learning Tools to Support Decision Making in the Diagnosis and Treatment of Pediatric Leukemia
353