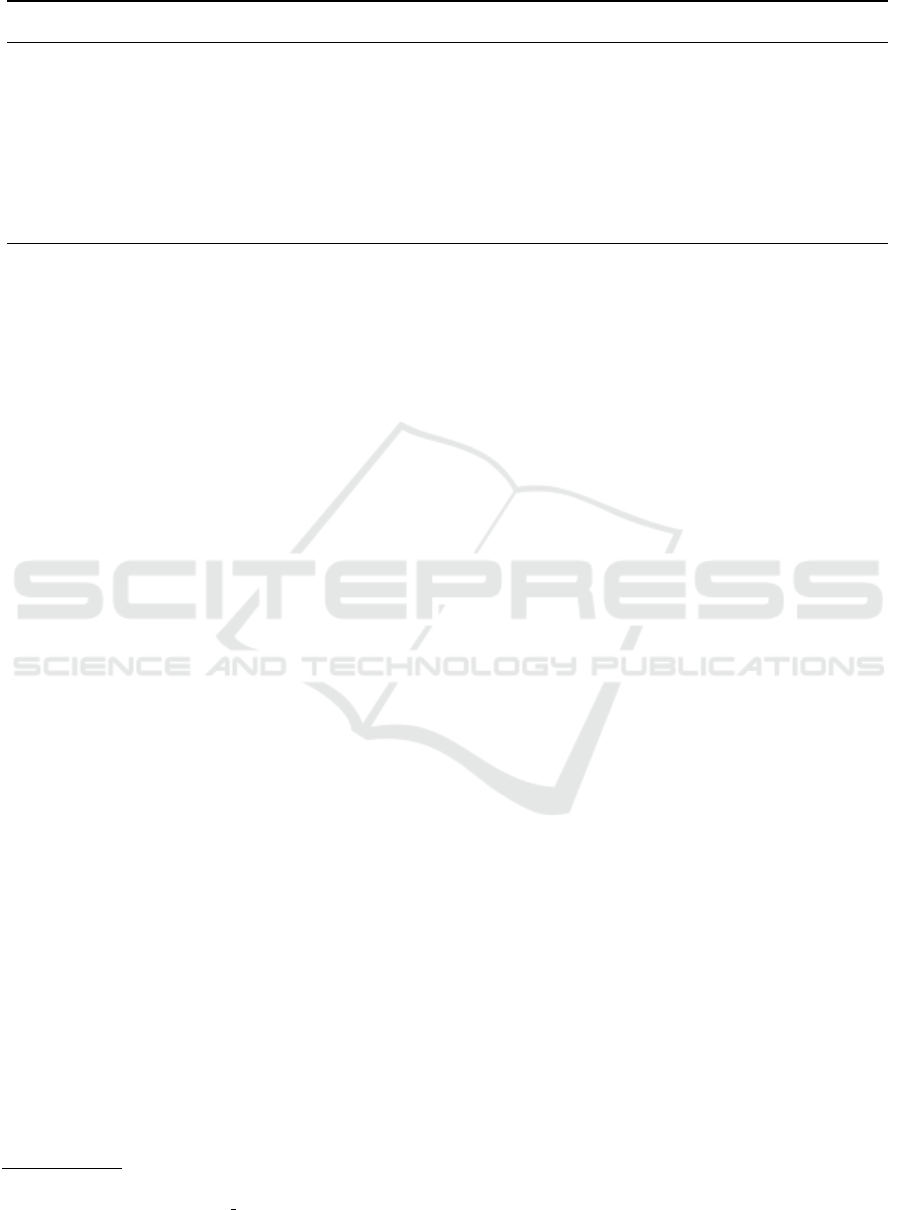
Table 1: Rank of the number of citations of the papers in the dataset (published in 2009) until 2012.
Ranking
@2012
Authors Title Journal
Citation
@2012
1 Park, S. H., et al.
Bulk heterojunction solar cells
with internal quantum efficiency approaching 100%.
Nature Photonics 1,126
2 Chen, H. Y., et al.
Polymer solar cells
with enhanced open-circuit voltage and efficiency.
Nature Photonics 930
3 Dennler, G., et al.
Polymer-fullerene bulk-heterojunction solar cells.
Advanced materials 747
4 Krebs, F. C., et al.
Fabrication and processing of polymer solar cells:
A review of printing and coating techniques.
Solar energy materials
and solar cells
495
5 Gr
¨
atzel, M., et al.
Recent advances in sensitized mesoscopic solar cells.
Accounts of
chemical research
465
have reported predicting these indices. Some studies
predict the h-index of future researchers(Ayaz et al.,
2018; Mir
´
o et al., 2017; Schreiber, 2013; Acuna et al.,
2012), studies that predict the number of citations af-
ter publication(Bai et al., 2019; Sasaki et al., 2016;
Stegehuis et al., 2015; Cao et al., 2016). Among
these, the difference is that Stegehuis et al.. and Cao
et al.. consider the number of citations one to three
years after publication and predict the number of cita-
tions in the reasonably distant future. In comparison,
Sasaki et al.. predict the number of citations three
years later without using citations after publication.
Recently, the application of deep learning tech-
niques to academic literature data has been promoted.
The SPECTER model(Cohan et al., 2020), trained on
the SciDocs dataset, is a representative example of ap-
plying text data in academic literature. However, the
SPECTER model uses the citation information of the
articles, and it does not simply obtain the distributed
representation of each article based on linguistic in-
formation alone. In this study, we used the learned
Sentence-BERT model(Reimers and Gurevych, 2019)
trained by the SNLI corpus(Bowman et al., 2015) as
a method to obtain the distributed representation for
each article.
On the other hand, there is an attempt to cap-
ture the citation information of academic literature
data as one huge graph and use it for task evalua-
tion such as link prediction. The SEAL model(Zhang
and Chen, 2018) is the top-ranked model on #ogbl-
citation2, for the citation prediction task in the aca-
demic literature dataset of the Open Graph Bench-
mark (OGB)(Weihua Hu, 2020), one of the bench-
mark datasets for graph data, as of February 2021
1
.
The SEAL model learns by sampling a pair of nodes
in a graph and using a subgraph containing the two
nodes to predict a link between the sampled nodes.
The SEAL model does not use the entire graph as
input but rather a large number of small subgraphs,
1
OGB:Leaderboards for Link Property Prediction:
https ogb
˙
stanford
˙
edudocsleader linkprop#ogbl-citation2
which has the advantage of being relatively easy to
apply to parallelization and large graphs.
3 METHODOLOGY
The purpose of this paper is to analyze the possibil-
ity of identifying papers with high impact by extract-
ing the number of citations after publication, which
is one of the representative impact indicators of sci-
entific research, and the corresponding information
on academic literature as a distributed representation.
In order to analyze the possibility of identifying pa-
pers with high impact, we use two methods to obtain
the distributed representation for each paper: one is
for linguistic information (title and abstract), and the
other is for citation information. We compare the dis-
tribution of the papers with the highest citations after
three years of the publication on the obtained variance
representation. The likelihood of identifying such pa-
pers is high if the papers with the highest citations are
skewed within a particular region and low otherwise.
This paper compares the likelihood of identifying the
papers with the highest citations by the method using
linguistic information and the method using citation
information for a relatively small dataset.
The method of comparison is as follows. Ob-
tain the distributed representation of each article by
two methods: one is the embedding method for lin-
guistic information, and the other is the embedding
method for citation information. After obtaining these
two distributed representations, we apply a clustering
method under the same number clusters k. Further-
more, we calculate the entropy of the entire dataset
with the percentage of papers in the same cluster that
will be the most cited papers in n years after publica-
tion. The following formula calculates the entropy.
H(P
t
) = −
∑
c∈C
P
t
(c)lnP
t
(c) (1)
However, the symbols in the equation are as follows:
Which Is More Helpful in Finding Scientific Papers to Be Top-cited in the Future: Content or Citations? Case Analysis in the Field of Solar
Cells 2009
361