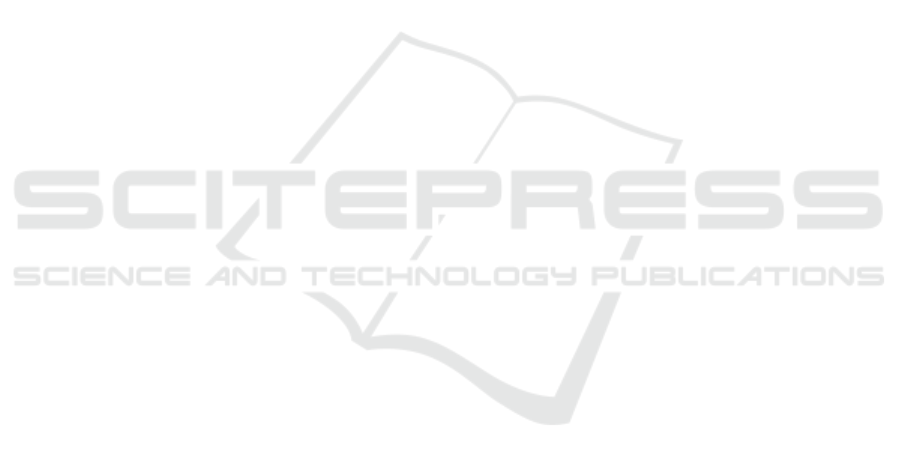
Girdhar, R. and Ramanan, D. (2017). Attentional pooling
for action recognition. In NeurIPS 2017, 31st Confer-
ence on Neural Information Processing Systems.
Jhuang, H., Gall, J., Zuffi, S., Schmid, C., and Black, M. J.
(2013). Towards understanding action recognition. In
Proceedings of the IEEE international conference on
computer vision, pages 3192–3199.
Kim, D., Cho, D., and Kweon, I. S. (2018). Self-supervised
video representation learning with space-time cubic
puzzles. In AAAI 2019, 33rd AAAI Conference on Ar-
tificial Intelligence.
Kolesnikov, A., Zhai, X., and Beyer, L. (2019). Revisiting
self-supervised visual representation learning. In Pro-
ceedings of the IEEE/CVF Conference on Computer
Vision and Pattern Recognition (CVPR).
Kuehne, H., Jhuang, H., Garrote, E., Poggio, T., and Serre,
T. (2011). Hmdb: A large video database for human
motion recognition. In 2011 International Conference
on Computer Vision, pages 2556–2563.
Lee, H.-Y., Huang, J.-B., Singh, M., and Yang, M.-H.
(2017). Unsupervised representation learning by sort-
ing sequences. In Proceedings of the IEEE Interna-
tional Conference on Computer Vision (ICCV).
Li, B., Dai, Y., Cheng, X., Chen, H., Lin, Y., and He,
M. (2017a). Skeleton based action recognition using
translation-scale invariant image mapping and multi-
scale deep cnn. In 2017 IEEE International Con-
ference on Multimedia & Expo Workshops (ICMEW),
pages 601–604. IEEE.
Li, C., Zhong, Q., Xie, D., and Pu, S. (2017b). Skeleton-
based action recognition with convolutional neural
networks. In 2017 IEEE International Conference on
Multimedia Expo Workshops (ICMEW), pages 597–
600.
Li, X., Liu, S., Mello, S. D., Wang, X., Kautz, J., and
Yang, M.-H. (2019). Joint-task self-supervised learn-
ing for temporal correspondence. In NeurIPS 2019,
33rd Conference on Neural Information Processing
Systems.
Lin, L., Song, S., Yang, W., and Liu, J. (2020). Ms2l :
Multi-task self-supervised learning for skeleton based
action recognition. Proceedings of the 28th ACM In-
ternational Conference on Multimedia.
Misra, I., Zitnick, C. L., and Hebert, M. (2016). Shuffle
and learn: Unsupervised learning using temporal or-
der verification. In ECCV 2016, 12th European Con-
ference on Computer Vision.
Noroozi, M. and Favaro, P. (2016). Unsupervised learning
of visual representations by solving jigsaw puzzles. In
ECCV 2016, 12th European Conference on Computer
Vision.
Pirk, S., Khansari, M., Bai, Y., Lynch, C., and Sermanet, P.
(2019). Online object representations with contrastive
learning.
Rohrbach, M., Rohrbach, A., Regneri, M., Amin, S., An-
driluka, M., Pinkal, M., and Schiele, B. (2015). Rec-
ognizing fine-grained and composite activities using
hand-centric features and script data. International
Journal of Computer Vision, pages 1–28.
Shi, L., Zhang, Y., Cheng, J., and Lu, H. (2019). Skeleton-
based action recognition with directed graph neural
networks. In Proceedings of the IEEE/CVF Confer-
ence on Computer Vision and Pattern Recognition,
pages 7912–7921.
Su, J.-C., Maji, S., and Hariharan, B. (2020). When does
self-supervision improve few-shot learning? In ECCV
2020, 16th European Conference on Computer Vision.
Sumer, O., Dencker, T., and Ommer, B. (2017). Self-
supervised learning of pose embeddings from spa-
tiotemporal relations in videos. In Proceedings of the
IEEE International Conference on Computer Vision
(ICCV).
Tran, D., Wang, H., Torresani, L., Ray, J., LeCun, Y., and
Paluri, M. (2018). A closer look at spatiotemporal
convolutions for action recognition. In Proceedings of
the IEEE Conference on Computer Vision and Pattern
Recognition (CVPR).
Vondrick, C., Shrivastava, A., Fathi, A., Guadarrama, S.,
and Murphy, K. (2018). Tracking emerges by coloriz-
ing videos. In ECCV 2018, 14th European Conference
on Computer Vision.
Wang, J., Jiao, J., Bao, L., He, S., Liu, Y., and Liu, W.
(2019). Self-supervised spatio-temporal representa-
tion learning for videos by predicting motion and ap-
pearance statistics. In Proceedings of the IEEE/CVF
Conference on Computer Vision and Pattern Recogni-
tion (CVPR).
Wu, J., Li, Y., Wang, L., Wang, K., Li, R., and Zhou, T.
(2019a). Skeleton based temporal action detection
with yolo. In Journal of Physics: Conference Series,
volume 1237, page 022087. IOP Publishing.
Wu, Y., Kirillov, A., Massa, F., Lo, W.-Y., and Girshick, R.
(2019b). Detectron2.
Yamato, J., Ohya, J., and Ishii, K. (1992). Recognizing
human action in time-sequential images using hidden
markov model. In CVPR, volume 92, pages 379–385.
Yan, S., Xiong, Y., and Lin, D. (2018). Spatial Tempo-
ral Graph Convolutional Networks for Skeleton-Based
Action Recognition. Proceedings of the AAAI Confer-
ence on Artificial Intelligence, 32(1).
Zhang, R., Isola, P., and Efros, A. A. (2016). Colorful image
colorization. In ECCV 2016, 12th European Confer-
ence on Computer Vision.
Implicitly using Human Skeleton in Self-supervised Learning: Influence on Spatio-temporal Puzzle Solving and on Video Action
Recognition
135