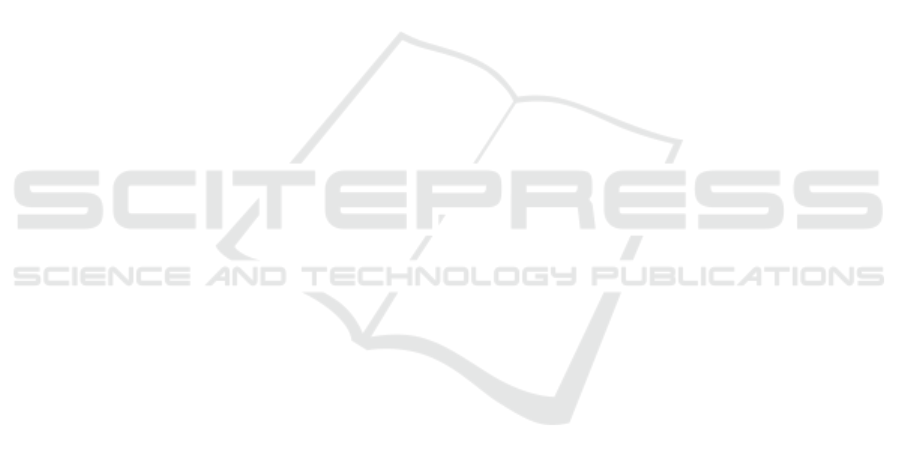
6 FINAL REMARKS AND
FUTURE WORK
In the work described in this paper it was possible to
explore how key phrases associated to different lev-
els of Work Domain Analysis are used in football
matches commentary published electronically by dif-
ferent sources. From this exploratory work the fol-
lowing conclusions could be obtained:
• The similarity score between commentary entries
and WDA key phrases shows a great dispersion
across all domains (sources, levels, and entries);
• The higher similarity values are obtained at the
WDA level L3. Values & priority measures. It is
worth of note that the key phrases identified at this
level have usually a closely related match annota-
tion item (e.g., Goals scored);
• Contrary to what may be expected, comments
from users in social media show, for all WDA
levels, higher semantic similarity values that com-
mentary entries in formal media.
Concerning future work, we foresee six main
ideas on how to increase the potential of this project:
• The informal and formal media have close simi-
larity scores, with higher similarity values being
achieved by fans comments - it is important to un-
derstand how this conclusion generalise to other
matches;
• Perform a more comprehensive study of the dif-
ferent key phrases, notably their relative ranking
and their potentially hierarchical structure (e.g.,
Goals and Goals scored/conceded or Runs and
Runs with/without the ball).
• Sentiment polarity of fans perspective can pro-
vide unanticipated insights concerning perfor-
mance analysis of football players. Sentiment
analysis captures the subjective part of perfor-
mance, and analysis based on metrics (stats about
passes, goals, and assists, for example) its objec-
tive part;
• Apply our method to other social media platforms
and sources of formal media commentary notably,
comparing how users behaviour in different plat-
forms;
• Try to adapt the used methods to other sports;
• The creation of a specific platform to connect
football fans and the Data Department of the Foot-
ball Teams could lead to an integrated (qualita-
tive and quantitative) perspective on performance
analysis.
ACKNOWLEDGEMENTS
Rui J. Lopes was partly supported by the Fundac¸
˜
ao
para a Ci
ˆ
encia e Tecnologia, under Grant Num-
ber UIDB/50008/2020 attributed to Instituto de
Telecomunicac¸
˜
oes. Ricardo Ribeiro was partly sup-
ported by national funds through Fundac¸
˜
ao para
a Ci
ˆ
encia e a Tecnologia (FCT) with reference
UIDB/50021/2020.
REFERENCES
Barros, B., Serr
˜
ao, C., and Lopes, R. J. (2018). Distributed
crowd-based annotation of soccer games using mo-
bile devices. In Proceedings of the 6th International
Congress on Sport Sciences Research and Technology
Support - Volume 1: icSPORTS,, pages 40–48.
Berber, E., McLean, S., Beanland, V., Read, G. J. M., and
Salmon, P. M. (2020). Defining the attributes for spe-
cific playing positions in football match-play: A com-
plex systems approach. Journal of Sports Sciences,
38(11–12):1248–1258.
C. Carling, A. M. W. and Reilly, T. (2005). Handbook of
soccer match analysis: A systematic approach to im-
proving performance. Routledge, 1st edition.
Devlin, J., Chang, M.-W., Lee, K., and Toutanova, K.
(2019). BERT: Pre-training of deep bidirectional
transformers for language understanding. In Proc. of
the 2019 Conference of the North American Chapter
of the Association for Computational Linguistics: Hu-
man Language Technologies, pages 4171–4186.
Mclean, S., Salmon, P., Gorman, A., Read, G., and
Solomon, C. (2017). What’s in a game? A systems
approach to enhancing performance analysis in foot-
ball. PLOS ONE, 12:e0172565.
Sanh, V., Debut, L., Chaumond, J., and Wolf, T. (2019).
Distilbert, a distilled version of BERT: smaller, faster,
cheaper and lighter. CoRR, abs/1910.01108.
Stein, M., Janetzko, H., Breitkreutz, T., Seebacher, D.,
Schreck, T., Grossniklaus, M., Couzin, I., and Keim,
D. A. (2016). Director’s cut: Analysis and annota-
tion of soccer matches. IEEE Computer Graphics and
Applications, 36(5):50–60.
Vaswani, A., Shazeer, N., Parmar, N., Uszkoreit, J., Jones,
L., Gomez, A. N., Kaiser, u., and Polosukhin, I.
(2017). Attention is all you need. In Proceedings of
the 31st International Conference on Neural Informa-
tion Processing Systems, page 6000–6010.
icSPORTS 2021 - 9th International Conference on Sport Sciences Research and Technology Support
150