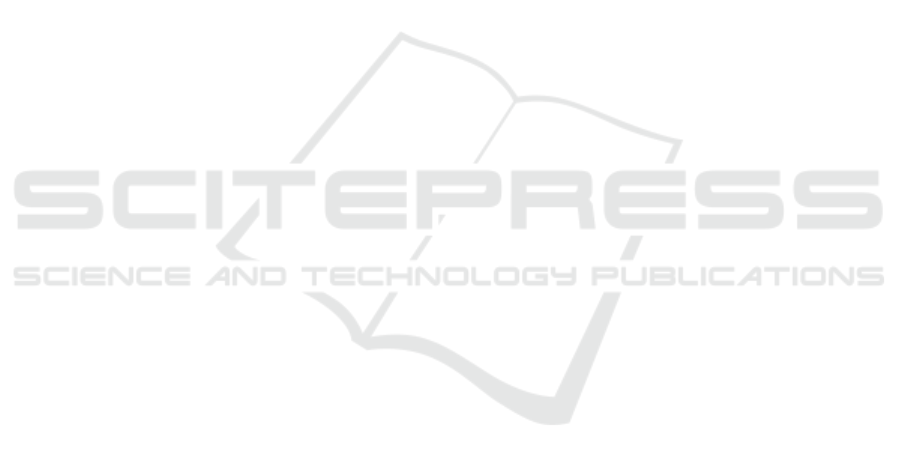
comes precise enough. The challenge will be in par-
ticular to model properly the complex influences driv-
ing the internal forces.
When the model has reached an acceptable level
of accuracy, it can be further implemented as a soft-
ware agent integrated in a DT structure, to simulate a
human worker, learning and adapting from the worker
behaviour and synchronising with the field, to reach a
state where emulation is possible. Of course the HDT
by nature carries all the concerns linked to data pro-
tection, acceptance and ethics, which we did not ad-
dress here. This is another story...
REFERENCES
Alam, K. M. and El Saddik, A. (2017). C2ps: A digital
twin architecture reference model for the cloud-based
cyber-physical systems. IEEE Access, 5:2050–2062.
Baskaran, S., Niaki, F. A., Tomaszewski, M., Gill, J. S.,
Chen, Y., Jia, Y., Mears, L., and Krovi, V. (2019).
Digital human and robot simulation in automotive as-
sembly using siemens process simulate: A feasibility
study. Procedia Manufacturing, 34:986–994. 47th
SME North American Manufacturing Research Con-
ference, NAMRC 47, Pennsylvania, USA.
Blake, T. (2016). The human’s digital twin. Professional
Engineering, 29(12):30 – 33.
Burden, D. and Savin-Baden, M. (2019). Virtual Humans:
Today and Tomorrow. Chapman & Hall/CRC Artifi-
cial Intelligence and Robotics Series. CRC Press, Tay-
lor & Francis Group.
Case, K., Marshall, R., and Summerskill, S. (2016). Digi-
tal human modelling over four decades. International
Journal of the Digital Human, 1(2):pp. 112–131.
Costa, P. T. and McCrae, R. R. (1988). Personality in adult-
hood: a six-year longitudinal study of self-reports and
spouse ratings on the neo personality inventory. Jour-
nal of personality and social psychology, 54(5):853.
Hafez, W. (2020). Human digital twin: Enabling human-
multi smart machines collaboration. In Bi Y., Bha-
tia R., K. S. e., editor, Intelligent Systems and Applica-
tions. IntelliSys 2019. Advances in Intelligent Systems
and Computing, volume 1038. Springer, Cham.
Kamara-Esteban, O., Azkune, G., Pijoan, A., Borges,
C. E., Alonso-Vicario, A., and de Ipi
˜
na, D. L. (2017).
Massha: An agent-based approach for human activity
simulation in intelligent environments. Pervasive and
Mobile Computing, 40:279–300.
Kennedy, W. G. (2012). Modelling Human Behaviour
in Agent-Based Models, pages 167–179. Springer
Netherlands, Dordrecht.
Kotseruba, I. and Tsotsos, J. K. (2020). 40 years of
cognitive architectures: core cognitive abilities and
practical applications. Artificial Intelligence Review,
53(1):17–94.
Lieto, A., Bhatt, M., Oltramari, A., and Vernon, D. (2018).
The role of cognitive architectures in general artifi-
cial intelligence. Cognitive Systems Research, 48:1–3.
Cognitive Architectures for Artificial Minds.
Longo, F., Nicoletti, L., and Padovano, A. (2019). Modeling
workers’ behavior: A human factors taxonomy and a
fuzzy analysis in the case of industrial accidents. Int.
Journal of Industrial Ergonomics, 69:29–47.
Lu, Y., Liu, C., Wang, K. I.-K., Huang, H., and Xu,
X. (2020). Digital twin-driven smart manufactur-
ing: Connotation, reference model, applications and
research issues. Robotics and Computer-Integrated
Manufacturing, 61:101837.
Negri, E., Fumagalli, L., and Macchi, M. (2017). A re-
view of the roles of digital twin in cps-based produc-
tion systems. Procedia Manufacturing, 11:939 – 948.
27th International Conference on Flexible Automa-
tion and Intelligent Manufacturing, FAIM2017, 27-30
June 2017, Modena, Italy.
Nikolakis, N., Alexopoulos, K., Xanthakis, E., and Chrys-
solouris, G. (2019). The digital twin implementation
for linking the virtual representation of human-based
production tasks to their physical counterpart in the
factory-floor. International Journal of Computer Inte-
grated Manufacturing, 32(1):1–12.
Ortony, A., Clore, G. L., and Collins, A. (1990). The cog-
nitive structure of emotions. Cambridge university
press.
Peruzzini, M., Grandi, F., and Pellicciari, M. (2018). Ex-
ploring the potential of operator 4.0 interface and
monitoring. Computers & Industrial Engineering.
Russell, J. A. (1980). A circumplex model of af-
fect. Journal of Personality and Social Psychology,
6(39):1161–1178.
Saracco, R., Autiosalo, J., Kerckhove, D., Flammini, F.,
and Nisiotis, L. (2020). Personal digital twins and
their role in epidemics control: An IEEE digital re-
ality white paper.
Schmidt, F. L. and Hunter, J. E. (1998). The validity and
utility of selection methods in personnel psychology:
Practical and theoretical implications of 85 years of
research findings. Psychological bulletin, 124(2):262.
Shafto, M., Conroy, M., Doyle, R., Glaessgen, E., Kemp,
C., LeMoigne, J., and Wang, L. (2010). Draft model-
ing, simulation, information technology & processing
roadmap, technology area 11. Technical report, Na-
tional Aeronautics and Space Administration, NASA
Headquarters, Washington, DC 20546.
Shvo, M., Buhmann, J., and Kapadia, M. (2019). An inter-
dependent model of personality, motivation, emotion,
and mood for intelligent virtual agents. In Proceed-
ings of the 19th ACM International Conference on In-
telligent Virtual Agents, pages 65–72.
S
´
anchez-L
´
opez, Y. and Cerezo, E. (2019). Designing emo-
tional bdi agents: good practices and open questions.
The Knowledge Engineering Review, 34:e26.
Tao, F., Zhang, H., Liu, A., and Nee, A. Y. C. (2019). Dig-
ital twin in industry: State-of-the-art. IEEE Transac-
tions on Industrial Informatics, 15(4):2405–2415.
Zibuschka, J., Ruff, C., Horch, A., and Roßnagel, H.
(2020). A human digital twin as building block of
open identity management for the internet of things.
In Roßnagel, H., Schunck, C. H., M
¨
odersheim, S.,
and H
¨
uhnlein, D., editors, Open Identity Summit 2020,
pages 133–142, Bonn. Gesellschaft f
¨
ur Informatik
e.V.
IN4PL 2021 - 2nd International Conference on Innovative Intelligent Industrial Production and Logistics
144