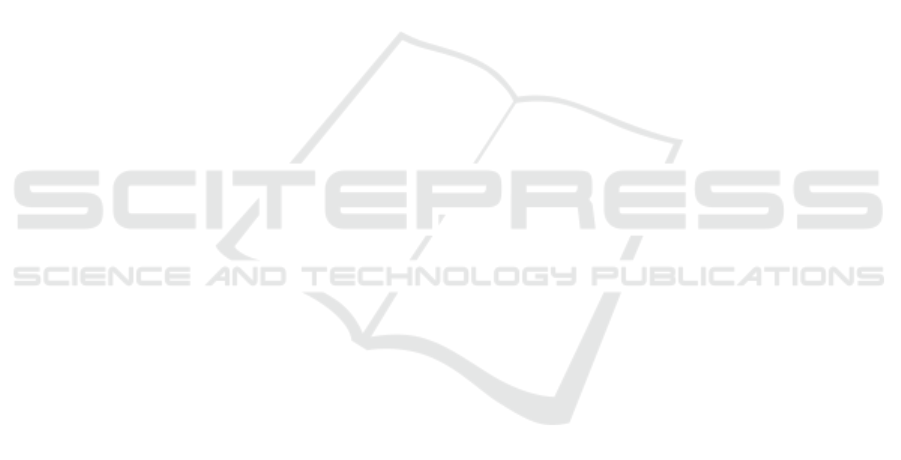
dition, these results have been further improved by
using a tailored splitting of the network. Indeed, the
latter allows a better follow-up of community struc-
tures.
REFERENCES
Br
´
odka, P., Saganowski, S., and Kazienko, P. (2013).
Ged: the method for group evolution discovery in so-
cial networks. Social Network Analysis and Mining,
3(1):1–14.
Clauset, A., Newman, M. E., and Moore, C. (2004). Finding
community structure in very large networks. Physical
review E, 70(6):066111.
Dakiche, N., Benbouzid-Si Tayeb, F., Benatchba, K., and
Slimani, Y. (2021). Tailored network splitting for
community evolution prediction in dynamic social
networks. New Generation Computing, pages 1–38.
Dakiche, N., Benbouzid-Si Tayeb, F., Slimani, Y., and Be-
natchba, K. (2018). Sensitive analysis of timeframe
type and size impact on community evolution predic-
tion. In 2018 IEEE International Conference on Fuzzy
Systems (FUZZ-IEEE). IEEE.
Dakiche, N., Benbouzid-Si Tayeb, F., Slimani, Y., and
Benatchba, K. (2019). Community evolution pre-
diction in dynamic social networks using commu-
nity features’ change rates. In Proceedings of the
34th ACM/SIGAPP Symposium on Applied Comput-
ing, pages 2078–2085.
Diakidis, G., Karna, D., Fasarakis-Hilliard, D., Vogiatzis,
D., and Paliouras, G. (2015). Predicting the evolution
of communities in social networks. In Proceedings of
the 5th International Conference on Web Intelligence,
Mining and Semantics, pages 1–6. ACM.
El Moussaoui, M., Agouti, T., Tikniouine, A., and El Ad-
nani, M. (2019). A comprehensive literature review on
community detection: Approaches and applications.
Procedia Computer Science, 151:295–302.
Hall, M., Frank, E., Holmes, G., Pfahringer, B., Reutemann,
P., and Witten, I. H. (2009). The weka data min-
ing software: An update. SIGKDD Explor. Newsl.,
11(1):10–18.
He, Z., Tajeuna, E. G., Wang, S., and Bouguessa, M. (2017).
A comparative study of different approaches for track-
ing communities in evolving social networks. In 2017
IEEE International Conference on Data Science and
Advanced Analytics (DSAA), pages 89–98. IEEE.
Holme, P. and Saram
¨
aki, J. (2012). Temporal networks.
Physics reports, 519(3):97–125.
˙
Ilhan, N. and
¨
O
˘
g
¨
ud
¨
uc
¨
u, S¸. G. (2016). Feature identification
for predicting community evolution in dynamic social
networks. Engineering Applications of Artificial In-
telligence, 55:202–218.
Omodei, E., De Domenico, M., and Arenas, A. (2015).
Characterizing interactions in online social networks
during exceptional events. Frontiers in Physics, 3:59.
Palla, G., Der
´
enyi, I., Farkas, I., and Vicsek, T. (2005).
Uncovering the overlapping community structure of
complex networks in nature and society. Nature,
435(7043):814–818.
Panzarasa, P., Opsahl, T., and Carley, K. M. (2009). Pat-
terns and dynamics of users’ behavior and interaction:
Network analysis of an online community. Journal
of the American Society for Information Science and
Technology, 60(5):911–932.
Pavlopoulou, M. E. G., Tzortzis, G., Vogiatzis, D., and
Paliouras, G. (2017). Predicting the evolution of com-
munities in social networks using structural and tem-
poral features. In 2017 12th International Workshop
on Semantic and Social Media Adaptation and Per-
sonalization (SMAP), pages 40–45. IEEE.
Rajita, B., Ranjan, Y., Umesh, C. T., and Panda, S. (2020).
Spark-based parallel method for prediction of events.
Arabian Journal for Science and Engineering, pages
1–17.
Saganowski, S., Br
´
odka, P., Koziarski, M., and Kazienko,
P. (2019). Analysis of group evolution prediction in
complex networks. PloS one, 14(10):e0224194.
Sasaki, Y. (2007). The truth of the f-measure. Teach Tutor
Mater, 1(5):1–5.
Shahriari, M., Gunashekar, S., Domarus, M. v., and
Klamma, R. (2016). Predictive analysis of temporal
and overlapping community structures in social me-
dia. In Proceedings of the 25th International Confer-
ence Companion on World Wide Web, pages 855–860.
International World Wide Web Conferences Steering
Committee.
Tabassum, S., Pereira, F. S., Fernandes, S., and Gama, J.
(2018). Social network analysis: An overview. Wiley
Interdisciplinary Reviews: Data Mining and Knowl-
edge Discovery, 8(5):e1256.
Takaffoli, M., Rabbany, R., and Za
¨
ıane, O. R. (2014). Com-
munity evolution prediction in dynamic social net-
works. In 2014 IEEE/ACM International Conference
on Advances in Social Networks Analysis and Mining
(ASONAM), pages 9–16. IEEE.
Viswanath, B., Mislove, A., Cha, M., and Gummadi, K. P.
(2009). On the evolution of user interaction in face-
book. In Proceedings of the 2nd ACM SIGCOMM
Workshop on Social Networks (WOSN’09).
WEBIST 2021 - 17th International Conference on Web Information Systems and Technologies
102