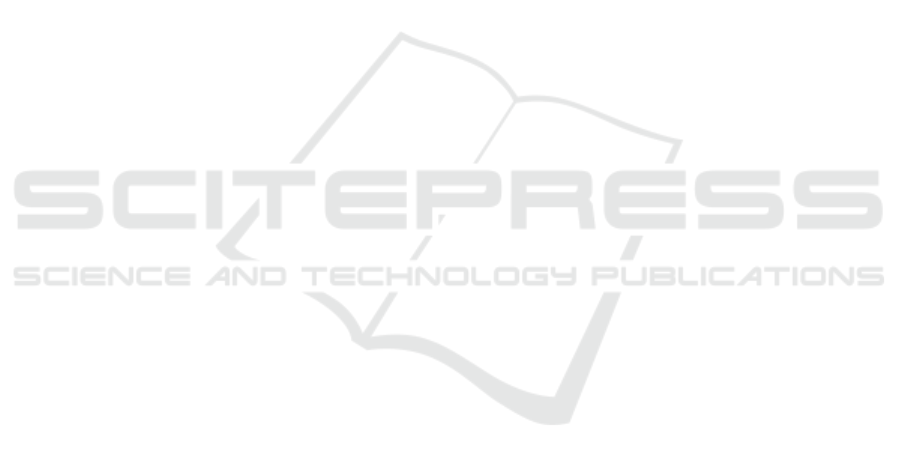
be part of an artificial intelligence system for tax fraud
detection.
For future studies new variables should be in-
cluded, such as the issuance of invoices, billing with
sales on credit cards, or changes in the number of em-
ployees. Or yet, ANN assembles models associated
with other Machine Learning algorithms.
REFERENCES
Abdou, H., P. J. (2011). Credit scoring, statistical tech-
niques and evaluation criteria: a review of the liter-
ature. Intelligent Systems in Accounting, Finance &
Management, 18(2/3):59–88.
Aggarwal, C. C. (2015). Data mining: the textbook.
Springer.
An, J., Zheng, Q., Wei, R., Dong, B., and Li, X. (2020).
Neud-tri: Network embedding based on upstream and
downstream for transaction risk identification. In Pro-
ceedings - 2020 IEEE 44th Annual Computers, Soft-
ware, and Applications Conference, COMPSAC 2020,
pages 277–286.
Bahrami, M., Bozkaya, B., and Balcisoy, S. (2020). Using
behavioral analytics to predict customer invoice pay-
ment. Big Data, 8(1):25–37.
Bardelli, C., Rondinelli, A., Vecchio, R., and Figini, S.
(2020). Automatic electronic invoice classification us-
ing machine learning models. Machine Learning and
Knowledge Extraction, 2(4):617–629.
Bramer, M. (2016). Principles of Data Mining, volume 1.
Springer, London.
Bromiley, P., M. M. N. A. R. E. (2015). Enterprise risk man-
agement: Review, critique, and research directions.
Long Range Planning, 48(4):265–276.
Caffo, B. (2015). Regression Models for Data Science in R,
volume 1. Leanpub.
Cha, S. ., Wang, H., Tan, Z., Joung, Y. ., Tseng, Y. ., and
Yeh, K. . (2020). On privacy aware carriers for value-
possessed e-invoices considering intelligence mining.
IEEE Transactions on Emerging Topics in Computa-
tional Intelligence, 4(5):641–652.
Fayyad, U., P. G. S. P. (1996). From data mining to knowl-
edge discovery in databases. AI Magazine, 17(3):18.
Golbayani, P., Florescu, I., and Chatterjee, R. (2020). A
comparative study of forecasting corporate credit rat-
ings using neural networks, support vector machines,
and decision trees. The North American Journal of
Economics and Finance, 54:101251.
Gouv
ˆ
ea M., Gonc¸alves E., M. D. (2013). An
´
alise de risco
de cr
´
edito com aplicac¸
˜
ao de regress
˜
ao log
´
ıstica e re-
des neurais. Revista Contabilidade Vista & Revista,
24(4):96–123.
He, Y., Wang, C., Li, N., and Zeng, Z. (2020). Attention and
memory-augmented networks for dual-view sequen-
tial learning. In Proceedings of the ACM SIGKDD In-
ternational Conference on Knowledge Discovery and
Data Mining, pages 125–134.
Hosmer Jr., D., L. S. (2004). Applied logistic regression,
volume 1. John Wiley & Sons.
Jabeur, S. B., Serret, V., et al. (2021). Bankruptcy prediction
using fuzzy convolutional neural networks. In World
Finance Conference.
Kim, S., Tsai, Y. ., Singh, K., Choi, Y., Ibok, E., Li, C. ., and
Cha, M. (2020). Date: Dual attentive tree-aware em-
bedding for customs fraud detection. In Proceedings
of the ACM SIGKDD International Conference on
Knowledge Discovery and Data Mining, pages 2880–
2890.
Lessmann, S., B. B. S. H. e. a. (2015). Benchmarking state-
of-the-art classification algorithms for credit scoring:
An update of research. European Journal of Opera-
tional Research, 247(1):124–136.
Maher A., M. F. (2016). Classifiers consensus system ap-
proach for credit scoring. Knowledge-Based Systems,
104(1):89–105.
Mathews, J., Mehta, P., Suryamukhi, and Babu, S. (2020).
Link prediction techniques to handle tax evasion.
In ACM International Conference Proceeding Series,
pages 307–315.
Oliveira, P., G. S. (2018). Ci
ˆ
encia de Dados com R –
Introduc¸
˜
ao. Ed. IBPAD, Bras
´
ılia, 1 edition.
Pandey, T., J. A. D. S. and Cho, S. (2018). A novel commit-
tee machine and reviews of neural network and sta-
tistical models for currency exchange rate prediction:
An experimental analysis. Journal of King Saud Uni-
versity – Computer and Information Sciences.
Pochiraju, B. and Seshadri, S. (2019). Essentials of Busi-
ness Analytics: An Introduction to the Methodology
and Its Applications, volume 264. Springer.
Silva, L. A., P. S. M. B. C. (2016). Introduc¸
˜
ao a Minerac¸
˜
ao
de Dados: com aplicac¸
˜
oes em R. Ed. Elsevier, Rio de
Janeiro, 1 edition.
Teles, G., Rodrigues, J. J., Rab
ˆ
e, R. A., and Kozlov, S. A.
(2020). Artificial neural network and bayesian net-
work models for credit risk prediction. Journal of Ar-
tificial Intelligence and Systems, 2:118–132.
Thomas, L., C. J. and Edelman, D. (2017). Credit scoring
and its applications, volume 2. Siam.
Wu, D., O. D. L. D. A. (2015). Decision making in enter-
prise risk management: A review and introduction to
special issue. Omega, 57(Part A):1–4.
Yu, J., Qiao, Y., Sun, K., Zhang, H., and Yang, J. (2018).
Poster: Classification of transaction behavior in tax
invoices using compositional cnn-rnn model. In Ubi-
Comp/ISWC 2018 - Adjunct Proceedings of the 2018
ACM International Joint Conference on Pervasive and
Ubiquitous Computing and Proceedings of the 2018
ACM International Symposium on Wearable Comput-
ers, pages 315–318.
Towards a Smart Identification of Tax Default Risk with Machine Learning
429