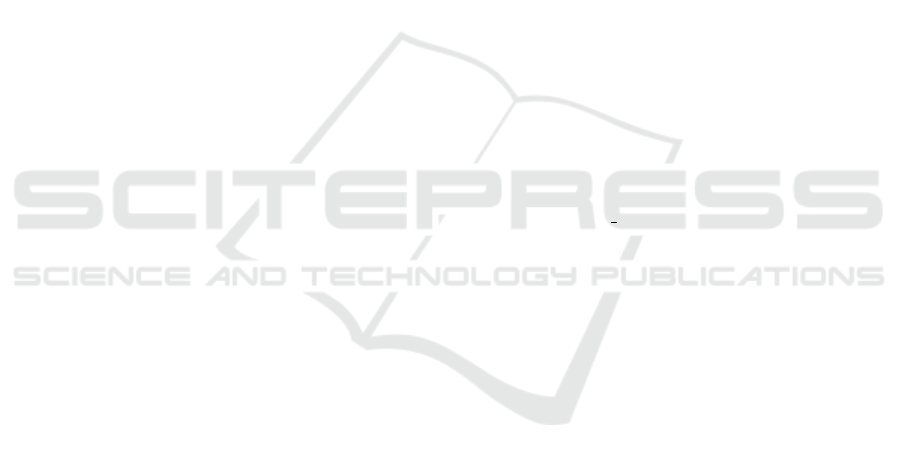
we could expand our methodology to other financial
instruments to explore the possibility that sentiment
data can act as features on government and corporate
bonds, or even on derivatives. Lastly, as we observed
in some models, there were cases where the mean
squared error was low, but the fit between the actual
and the predicted price was not good. Thus, it would
be very helpful if we could define a new measure that
can improve the fit capturing.
REFERENCES
Alshahrani Hasan, A. and Fong, A. C. (2018). Sentiment
Analysis Based Fuzzy Decision Platform for the Saudi
Stock Market. In 2018 IEEE International Confer-
ence on Electro/Information Technology (EIT), pages
0023–0029, Rochester, MI. IEEE.
Antweiler, W. and Frank, M. Z. (2004). Is all that talk
just noise? the information content of internet stock
message boards. The Journal of finance, 59(3):1259–
1294.
Beleveslis, D., Tjortjis, C., Psaradelis, D., and Nikoglou,
D. (2019). A hybrid method for sentiment analy-
sis of election related tweets. In 2019 4th South-
East Europe Design Automation, Computer Engineer-
ing, Computer Networks and Social Media Confer-
ence (SEEDA-CECNSM), pages 1–6. IEEE.
Blystone, D. (2019). Overbought or oversold? use the rela-
tive strength index to find out.
Bollen, J., Mao, H., and Zeng, X.-J. (2011). Twitter mood
predicts the stock market. Journal of Computational
Science, 2(1):1–8. arXiv: 1010.3003.
Chakraborty, P., Pria, U. S., Rony, M. R. A. H., and Majum-
dar, M. A. (2017). Predicting stock movement using
sentiment analysis of twitter feed. In 2017 6th Inter-
national Conference on Informatics, Electronics and
Vision & 2017 7th International Symposium in Com-
putational Medical and Health Technology (ICIEV-
ISCMHT), pages 1–6. IEEE.
Devenow, A. and Welch, I. (1996). Rational herding in
financial economics. European Economic Review,
40(3-5):603–615.
Fama, E. F. (1965). The behavior of stock-market prices.
The journal of Business, 38(1):34–105.
Fama, E. F. and French, K. R. (1993). Common risk factors
in the returns on stocks and bonds. Journal of financial
economics, 33(1):3–56.
Hagberg, A., Swart, P., and S Chult, D. (2008). Explor-
ing network structure, dynamics, and function using
networkx. Technical report, Los Alamos National
Lab.(LANL), Los Alamos, NM (United States).
H
´
ajek, P. (2018). Combining bag-of-words and senti-
ment features of annual reports to predict abnormal
stock returns. Neural Computing and Applications,
29(7):343–358.
Hochreiter, S. (1991). Investigations on dynamic neural net-
works. Diploma, Technical University, 91(1).
Hochreiter, S. and Schmidhuber, J. (1997). Long short-term
memory. Neural computation, 9(8):1735–1780.
Hutto, C. J. and Gilbert, E. (2014). Vader: A parsimonious
rule-based model for sentiment analysis of social me-
dia text. In Eighth international AAAI conference on
weblogs and social media.
Koukaras, P. and Tjortjis, C. (2019). Social media ana-
lytics, types and methodology. In Machine Learning
Paradigms, pages 401–427. Springer.
Koukaras, P., Tjortjis, C., and Rousidis, D. (2019). So-
cial media types: introducing a data driven taxonomy.
Computing, pages 1–46.
Kuepper, J. (2019). Timing trades with the commodity
channel index.
Malkiel, B. G. and Fama, E. F. (1970). Efficient capital
markets: A review of theory and empirical work. The
journal of Finance, 25(2):383–417.
Markowitz, H. M. (1991). Foundations of portfolio theory.
The journal of finance, 46(2):469–477.
Mitchell, C. (2019). Aroon oscillator definition and tactics.
Mittermayer, M.-a. and Knolmayer, G. (2006). News-
CATS: A News Categorization and Trading System.
In Sixth International Conference on Data Mining
(ICDM’06), pages 1002–1007, Hong Kong, China.
IEEE.
Oikonomou, L. and Tjortjis, C. (2018). A method for pre-
dicting the winner of the usa presidential elections us-
ing data extracted from twitter. In 2018 South-Eastern
European Design Automation, Computer Engineer-
ing, Computer Networks and Society Media Confer-
ence (SEEDA
CECNSM), pages 1–8. IEEE.
Page, L., Brin, S., Motwani, R., and Winograd, T. (1999).
The pagerank citation ranking: Bringing order to the
web. Technical report, Stanford InfoLab.
Rousidis, D., Koukaras, P., and Tjortjis, C. (2019). So-
cial media prediction: A literature review. Multimedia
Tools and Applications.
See-To, E. W. K. and Yang, Y. (2017). Market sentiment
dispersion and its effects on stock return and volatility.
Electronic Markets, 27(3):283–296.
Sharpe, W. F. (1964). Capital asset prices: A theory of mar-
ket equilibrium under conditions of risk. The journal
of finance, 19(3):425–442.
Sohangir, S., Petty, N., and Wang, D. (2018). Financial Sen-
timent Lexicon Analysis. In 2018 IEEE 12th Inter-
national Conference on Semantic Computing (ICSC),
pages 286–289, Laguna Hills, CA, USA. IEEE.
Staff, I. (2019). On-balance volume: The way to smart
money.
Tjortjis, C. and Keane, J. (2002). T3: a classification algo-
rithm for data mining. In International Conference on
Intelligent Data Engineering and Automated Learn-
ing, pages 50–55. Springer.
Treynor, J. L. (1962). Jack treynor’s’ toward a theory
of market value of risky assets’. Available at SSRN
628187.
Tzirakis, P. and Tjortjis, C. (2017). T3c: improving a de-
cision tree classification algorithm’s interval splits on
continuous attributes. Advances in Data Analysis and
Classification, 11(2):353–370.
Predicting Stock Market Movements with Social Media and Machine Learning
443