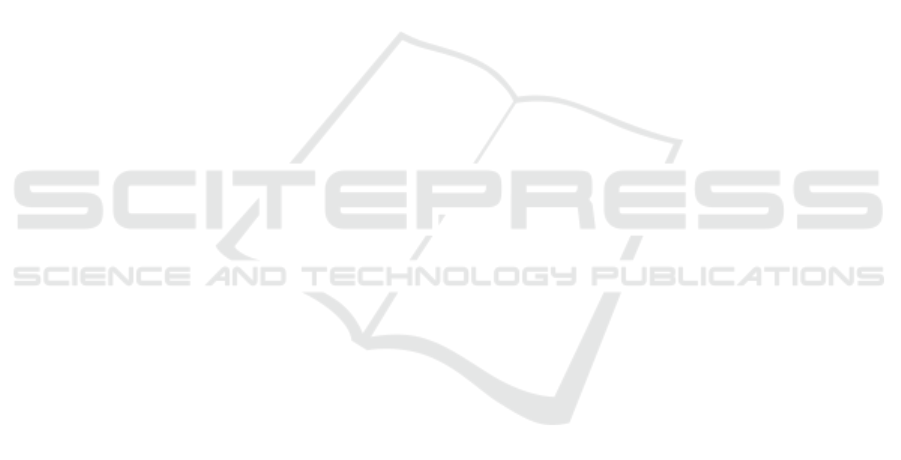
imal sciences: an overview. Frontiers in Veterinary
Science, 7:800.
French, G., Fisher, M., Mackiewicz, M., and Needle, C.
(2015). Convolutional neural networks for counting
fish in fisheries surveillance video.
Garcia, R., Prados, R., Quintana, J., Tempelaar, A., Gracias,
N., Rosen, S., V
˚
agstøl, H., and Løvall, K. (2020). Au-
tomatic segmentation of fish using deep learning with
application to fish size measurement. ICES Journal of
Marine Science, 77(4):1354–1366.
Grigorescu, S., Trasnea, B., Cocias, T., and Macesanu,
G. (2020). A survey of deep learning techniques
for autonomous driving. Journal of Field Robotics,
37(3):362–386.
He, K., Gkioxari, G., Doll
´
ar, P., and Girshick, R. B. (2017).
Mask R-CNN. CoRR, abs/1703.06870.
He, K., Zhang, X., Ren, S., and Sun, J. (2016). Deep resid-
ual learning for image recognition. In Proceedings of
the IEEE conference on computer vision and pattern
recognition, pages 770–778.
Konovalov, D. A., Saleh, A., Efremova, D. B., Domingos,
J. A., and Jerry, D. R. (2019). Automatic weight es-
timation of harvested fish from images. In 2019 Dig-
ital Image Computing: Techniques and Applications
(DICTA), pages 1–7. IEEE.
Kourou, K., Exarchos, T. P., Exarchos, K. P., Karamouzis,
M. V., and Fotiadis, D. I. (2015). Machine learning ap-
plications in cancer prognosis and prediction. Compu-
tational and structural biotechnology journal, 13:8–
17.
Kr
¨
ahenb
¨
uhl, P. and Koltun, V. (2011). Efficient inference
in fully connected crfs with gaussian edge potentials.
Advances in neural information processing systems,
24:109–117.
Li, L., Dong, B., Rigall, E., Zhou, T., Donga, J., and Chen,
G. (2021). Marine animal segmentation. IEEE Trans-
actions on Circuits and Systems for Video Technology.
Liu, S., De Mello, S., Gu, J., Zhong, G., Yang, M.-H., and
Kautz, J. (2017). Learning affinity via spatial propa-
gation networks. arXiv preprint arXiv:1710.01020.
Long, J., Shelhamer, E., and Darrell, T. (2015). Fully con-
volutional networks for semantic segmentation. In
Proceedings of the IEEE conference on computer vi-
sion and pattern recognition, pages 3431–3440.
Lopez-Marcano, S., Brown, C. J., Sievers, M., and Con-
nolly, R. M. (2021). The slow rise of technology:
Computer vision techniques in fish population con-
nectivity. Aquatic Conservation: Marine and Fresh-
water Ecosystems, 31(1):210–217.
Malde, K., Handegard, N. O., Eikvil, L., and Salberg, A.-B.
(2020). Machine intelligence and the data-driven fu-
ture of marine science. ICES Journal of Marine Sci-
ence, 77(4):1274–1285.
Mallet, D. and Pelletier, D. (2014). Underwater video tech-
niques for observing coastal marine biodiversity: a re-
view of sixty years of publications (1952–2012). Fish-
eries Research, 154:44–62.
Marchesan, M., Spoto, M., Verginella, L., and Ferrero,
E. A. (2005). Behavioural effects of artificial light
on fish species of commercial interest. Fisheries re-
search, 73(1-2):171–185.
Marmanis, D., Wegner, J. D., Galliani, S., Schindler, K.,
Datcu, M., and Stilla, U. (2016). Semantic segmenta-
tion of aerial images with an ensemble of cnss. ISPRS
Annals of the Photogrammetry, Remote Sensing and
Spatial Information Sciences, 2016, 3:473–480.
Qin, H., Peng, Y., and Li, X. (2014). Foreground extraction
of underwater videos via sparse and low-rank matrix
decomposition. In 2014 ICPR Workshop on Computer
Vision for Analysis of Underwater Imagery, pages 65–
72. IEEE.
Rawat, S., Benakappa, S., Kumar, J., Naik, K., Pandey,
G., and Pema, C. (2017). Identification of fish stocks
based on truss morphometric: A review. Journal of
Fisheries and Life Sciences, 2(1):9–14.
Ronneberger, O., Fischer, P., and Brox, T. (2015). U-net:
Convolutional networks for biomedical image seg-
mentation. In International Conference on Medical
image computing and computer-assisted intervention,
pages 234–241. Springer.
Rosen, S. and Holst, J. C. (2013). Deepvision in-trawl imag-
ing: Sampling the water column in four dimensions.
Fisheries Research, 148:64–73.
Sandler, M., Howard, A., Zhu, M., Zhmoginov, A., and
Chen, L.-C. (2018). Mobilenetv2: Inverted residu-
als and linear bottlenecks. In Proceedings of the IEEE
conference on computer vision and pattern recogni-
tion, pages 4510–4520.
Spampinato, C., Giordano, D., Di Salvo, R., Chen-Burger,
Y.-H. J., Fisher, R. B., and Nadarajan, G. (2010). Au-
tomatic fish classification for underwater species be-
havior understanding. In Proceedings of the first ACM
international workshop on Analysis and retrieval of
tracked events and motion in imagery streams, pages
45–50.
Storbeck, F. and Daan, B. (2001). Fish species recognition
using computer vision and a neural network. Fisheries
Research, 51(1):11–15.
Tchapmi, L., Choy, C., Armeni, I., Gwak, J., and Savarese,
S. (2017). Segcloud: Semantic segmentation of 3d
point clouds. In 2017 international conference on 3D
vision (3DV), pages 537–547. IEEE.
Wilhelms, I. et al. (2013). Atlas of length-weight relation-
ships of 93 fish and crustacean species from the north
sea and the north-east atlantic. Technical report, Jo-
hann Heinrich von Th
¨
unen Institute, Federal Research
Institute for Rural . . . .
Yang, X., Zeng, Z., Teo, S. G., Wang, L., Chandrasekhar, V.,
and Hoi, S. (2018). Deep learning for practical image
recognition: Case study on kaggle competitions. In
Proceedings of the 24th ACM SIGKDD international
conference on knowledge discovery & data mining,
pages 923–931.
Yu, C., Fan, X., Hu, Z., Xia, X., Zhao, Y., Li, R., and Bai,
Y. (2020). Segmentation and measurement scheme for
fish morphological features based on mask r-cnn. In-
formation Processing in Agriculture, 7(4):523–534.
Zhao, H., Shi, J., Qi, X., Wang, X., and Jia, J. (2017).
Pyramid scene parsing network. In Proceedings of
the IEEE conference on computer vision and pattern
recognition, pages 2881–2890.
ROBOVIS 2021 - 2nd International Conference on Robotics, Computer Vision and Intelligent Systems
164