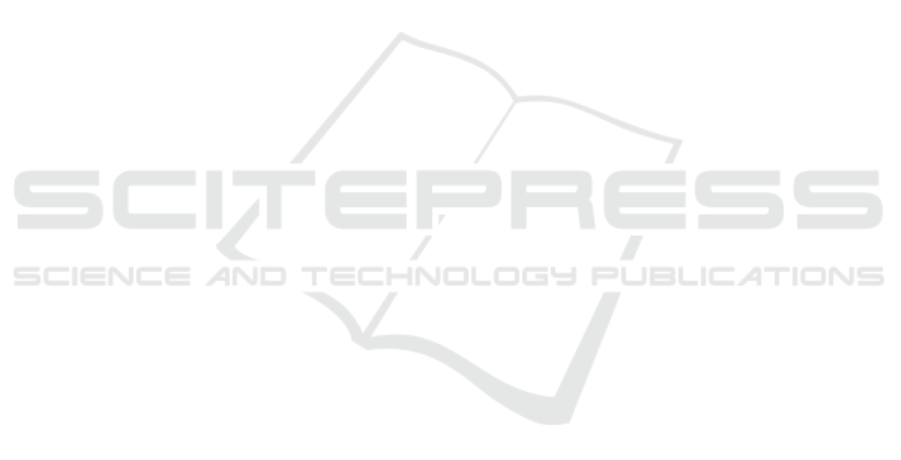
processing: A survey and future directions. CoRR,
abs/1709.01363.
Deng, X., Li, J., Liu, E., and Zhang, H. (2020). Task alloca-
tion algorithm and optimization model on edge collab-
oration. Journal of Systems Architecture, 110:101778.
Garcia Lopez, P., Montresor, A., Epema, D., Datta, A., Hi-
gashino, T., Iamnitchi, A., Barcellos, M., Felber, P.,
and Riviere, E. (2015). Edge-centric computing: Vi-
sion and challenges. SIGCOMM Comput. Commun.
Rev., 45(5):37–42.
Ghosh, R. and Simmhan, Y. (2018). Distributed scheduling
of event analytics across edge and cloud. ACM Trans.
Cyber-Phys. Syst., 2(4).
Helal, S., Delicato, F. C., Margi, C. B., Misra, S., and
Endler, M. (2020). Challenges and opportunities for
data science and machine learning in iot systems - a
timely debate: Part 1. IEEE Internet of Things Maga-
zine, 4(1):2–8.
Hernandez-Juarez, D., Chac
´
on, A., Espinosa, A., V
´
azquez,
D., Moure, J., and L
´
opez, A. (2016). Embedded real-
time stereo estimation via semi-global matching on
the gpu. Procedia Computer Science, 80:143–153.
Huh, J.-H. and Seo, Y.-S. (2019). Understanding edge com-
puting: Engineering evolution with artificial intelli-
gence. IEEE Access, 7:164229–164245.
Lahmar, I. B. and Boukadi, K. (2020). Resource alloca-
tion in fog computing: A systematic mapping study.
In 2020 Fifth International Conference on Fog and
Mobile Edge Computing (FMEC), pages 86–93, Paris,
France. IEEE.
Lee, Y.-L., Tsung, P.-K., and Wu, M. (2018). Techology
trend of edge ai. In 2018 International Symposium on
VLSI Design, Automation and Test (VLSI-DAT), pages
1–2.
Lera, I., Guerrero, C., and Juiz, C. (2019). Yafs: A simula-
tor for iot scenarios in fog computing. IEEE Access,
7:91745–91758.
Mohammadi, M., Al-Fuqaha, A., Sorour, S., and Guizani,
M. (2018). Deep learning for iot big data and stream-
ing analytics: A survey. IEEE Communications Sur-
veys Tutorials, 20:2923–2960.
Nakamura, E. F., Loureiro, A. A. F., and Frery, A. C.
(2007). Information fusion for wireless sensor net-
works: Methods, models, and classifications. ACM
Comput. Surv., 39(3):9–es.
Qiu, J., Wu, Q., Ding, G., Xu, Y., and Feng, S. (2016). A
survey of machine learning for big data processing.
EURASIP J. Adv. Sig. Proc., 2016:67.
Ramos, E., Morabito, R., and Kainulainen, J. (2019). Dis-
tributing intelligence to the edge and beyond [research
frontier]. IEEE Computational Intelligence Magazine,
14(4):65–92.
Rocha Neto, A., Silva, T. P., Batista, T., Delicato, F. C.,
Pires, P. F., and Lopes, F. (2021). Leveraging edge
intelligence for video analytics in smart city applica-
tions. Information, 12(1).
Rocha Neto, A., Silva, T. P., Batista, T. V., Delicato, F. C.,
Pires, P. F., and Lopes, F. (2020). An architecture for
distributed video stream processing in IoMT systems.
Open Journal of Internet Of Things (OJIOT), 6(1):89–
104.
R
¨
oger, H. and Mayer, R. (2019). A comprehensive survey
on parallelization and elasticity in stream processing.
ACM Comput. Surv., 52(2).
Tocz
´
e, K. and Nadjm-Tehrani, S. (2018). A taxonomy for
management and optimization of multiple resources
in edge computing. CoRR, abs/1801.05610.
Tsai, D. and Sang, H. (2010). Constructing a risk
dependency-based availability model. In 44th Annual
2010 IEEE International Carnahan Conference on
Security Technology, pages 218–220, San Jose, CA,
USA. IEEE.
Valera, M. and Velastin, S. A. (2005). Intelligent distributed
surveillance systems: a review. IEE Proceedings - Vi-
sion, Image and Signal Processing, 152(2):192–204.
Yang, M., Ma, H., Wei, S., Zeng, Y., Chen, Y., and Hu,
Y. (2020). A multi-objective task scheduling method
for fog computing in cyber-physical-social services.
IEEE Access, 8:65085–65095.
Zeng, D., Gu, L., Guo, S., Cheng, Z., and Yu, S. (2016).
Joint optimization of task scheduling and image place-
ment in fog computing supported software-defined
embedded system. IEEE Transactions on Computers,
65(12):3702–3712.
WEBIST 2021 - 17th International Conference on Web Information Systems and Technologies
166