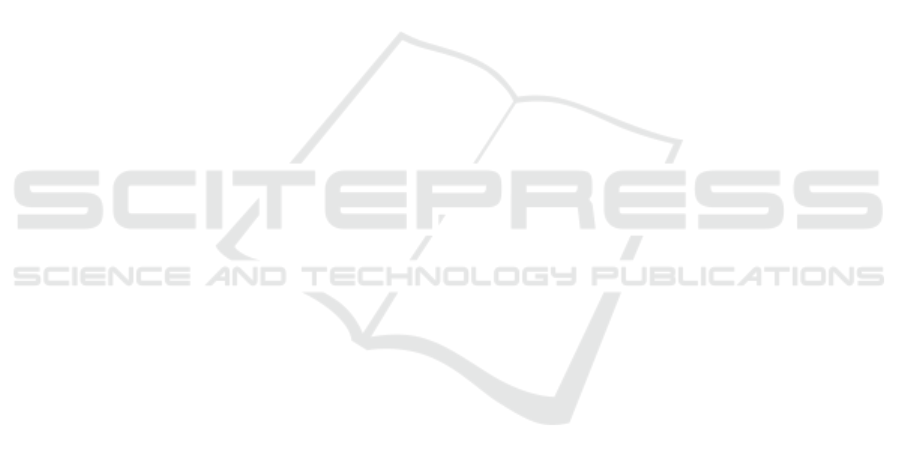
conpot (2018). conpot. https://github.com/mushorg/conpot.
[Online; accessed 13-July-2021].
Dionaea (2015). dionaea. https://github.com/DinoTools/
dionaea. [Online; accessed 13-July-2021].
Dowling, S., Schukat, M., and Barrett, E. (2018). Improv-
ing adaptive honeypot functionality with efficient re-
inforcement learning parameters for automated mal-
ware. Journal of Cyber Security Technology, 2(2):75–
91.
Fraunholz, D., Reti, D., Duque Anton, S., and Schotten,
H. D. (2018). Cloxy: A context-aware deception-as-a-
service reverse proxy for web services. In Proceedings
of the 5th ACM Workshop on Moving Target Defense,
pages 40–47.
Han, X., Kheir, N., and Balzarotti, D. (2017). Evaluation of
deception-based web attacks detection. In Proceed-
ings of the 2017 Workshop on Moving Target Defense,
pages 65–73.
Honeynet (2021). Sebek: kernel module based data capture.
[Online; accessed 13-July-2021].
Ishikawa, T. and Sakurai, K. (2017). Parameter manipula-
tion attack prevention and detection by using web ap-
plication deception proxy. In Proceedings of the 11th
International Conference on Ubiquitous Information
Management and Communication, pages 1–9.
Kippo (2014). Kippo. https://github.com/desaster/kippo.
[Online; accessed 13-July-2021].
Luo, T., Xu, Z., Jin, X., Jia, Y., and Ouyang, X. (2017). Iot-
candyjar: Towards an intelligent-interaction honeypot
for iot devices. Black Hat.
Mnih, V., Kavukcuoglu, K., Silver, D., Graves, A.,
Antonoglou, I., Wierstra, D., and Riedmiller, M.
(2013). Playing atari with deep reinforcement learn-
ing. arXiv preprint arXiv:1312.5602.
Mokube, I. and Adams, M. (2007). Honeypots: con-
cepts, approaches, and challenges. In Proceedings of
the 45th annual southeast regional conference, pages
321–326. ACM.
Morishita, S., Hoizumi, T., Ueno, W., Tanabe, R., Ga
˜
n
´
an,
C., van Eeten, M. J., Yoshioka, K., and Matsumoto, T.
(2019). Detect me if you. . . oh wait. an internet-wide
view of self-revealing honeypots. In 2019 IFIP/IEEE
Symposium on Integrated Network and Service Man-
agement (IM), pages 134–143.
Nawrocki, M., W
¨
ahlisch, M., Schmidt, T. C., Keil, C., and
Sch
¨
onfelder, J. (2016). A survey on honeypot software
and data analysis. arXiv preprint arXiv:1608.06249.
Ng, C. K., Pan, L., and Xiang, Y. (2018). Honeypot Frame-
works and Their Applications: A New Framework.
Springer.
Oosterhof, M. (2014). Cowrie. [Online; accessed 13-July-
2021].
Pa, Y. M. P., Suzuki, S., Yoshioka, K., Matsumoto, T.,
Kasama, T., and Rossow, C. (2015). Iotpot: analysing
the rise of iot compromises. In 9th {USENIX} Work-
shop on Offensive Technologies ({WOOT} 15).
Papalitsas, J., Rauti, S., Tammi, J., and Lepp
¨
anen, V.
(2018). A honeypot proxy framework for deceiving
attackers with fabricated content. In Cyber Threat In-
telligence, pages 239–258. Springer.
Pauna, A. and Bica, I. (2014). Rassh-reinforced adaptive
ssh honeypot. In Communications (COMM), 2014
10th International Conference on, pages 1–6. IEEE.
Pauna, A., Iacob, A.-C., and Bica, I. (2018). Qrassh-
a self-adaptive ssh honeypot driven by q-learning.
In 2018 international conference on communications
(COMM), pages 441–446. IEEE.
Portokalidis, G., Slowinska, A., and Bos, H. (2006). Argos:
an emulator for fingerprinting zero-day attacks for
advertised honeypots with automatic signature gen-
eration. ACM SIGOPS Operating Systems Review,
40(4):15–27.
Provos, N. (2003). Honeyd-a virtual honeypot daemon. In
10th DFN-CERT Workshop, Hamburg, Germany, vol-
ume 2, page 4.
Ramsbrock, D., Berthier, R., and Cukier, M. (2007). Pro-
filing attacker behavior following ssh compromises.
In Dependable Systems and Networks, 2007. DSN’07.
37th Annual IEEE/IFIP International Conference on,
pages 119–124. IEEE.
Schneier, B. (1999). Attack trees. Dr. Dobb’s journal,
24(12):21–29.
Seifert, C., Welch, I., and Komisarczuk, P. (2006). Taxon-
omy of honeypots. Technical report cs-tr-06/12, Vic-
toria University of Wellington, School of Mathemati-
cal and Computer Sciences.
Spitzner, L. (2003). Honeypots: Tracking Hackers, vol-
ume 1. Spitzner, Lance.
Surnin, O., Hussain, F., Hussain, R., Ostrovskaya, S.,
Polovinkin, A., Lee, J., and Fernando, X. (2019).
Probabilistic estimation of honeypot detection in in-
ternet of things environment. In 2019 International
Conference on Computing, Networking and Commu-
nications (ICNC), pages 191–196. IEEE.
Sutton, R. S. and Barto, A. G. (2018). Reinforcement learn-
ing: An introduction. MIT press.
Vetterl, A. and Clayton, R. (2018). Bitter harvest: Systemat-
ically fingerprinting low-and medium-interaction hon-
eypots at internet scale. In 12th {USENIX} Workshop
on Offensive Technologies ({WOOT} 18).
Wagener, G., Dulaunoy, A., Engel, T., et al. (2011a). He-
liza: talking dirty to the attackers. Journal in computer
virology, 7(3):221–232.
Wagener, G., State, R., Engel, T., and Dulaunoy, A.
(2011b). Adaptive and self-configurable honeypots.
In 12th IFIP/IEEE International Symposium on In-
tegrated Network Management (IM 2011) and Work-
shops, pages 345–352. IEEE.
Watkins, C. J. and Dayan, P. (1992). Q-learning. Machine
learning, 8(3-4):279–292.
DMMLACS 2021 - 2nd International Special Session on Data Mining and Machine Learning Applications for Cyber Security
574