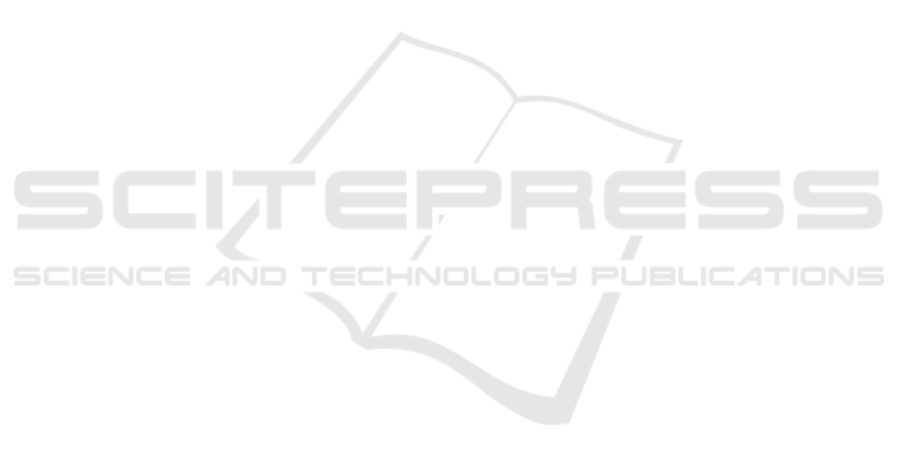
He, K., Gkioxari, G., Doll
´
ar, P., and Girshick, R. (2017).
Mask r-cnn. In Proceedings of the IEEE international
conference on computer vision, pages 2961–2969.
He, K., Zhang, X., Ren, S., and Sun, J. (2016). Deep resid-
ual learning for image recognition. In Proceedings of
the IEEE conference on computer vision and pattern
recognition, pages 770–778.
Huang, J., Rathod, V., Sun, C., Zhu, M., Korattikara, A.,
Fathi, A., Fischer, I., Wojna, Z., Song, Y., Guadar-
rama, S., et al. (2017). Speed/accuracy trade-offs for
modern convolutional object detectors. In Proceed-
ings of the IEEE conference on computer vision and
pattern recognition, pages 7310–7311.
Jeong, J., Park, H., and Kwak, N. (2017). Enhancement
of ssd by concatenating feature maps for object detec-
tion. arXiv preprint arXiv:1705.09587.
Li, J., Liang, X., Wei, Y., Xu, T., Feng, J., and Yan, S.
(2017). Perceptual generative adversarial networks for
small object detection. In Proceedings of the IEEE
conference on computer vision and pattern recogni-
tion, pages 1222–1230.
Lim, J.-S., Astrid, M., Yoon, H.-J., and Lee, S.-I. (2021).
Small object detection using context and attention.
In 2021 International Conference on Artificial Intel-
ligence in Information and Communication (ICAIIC),
pages 181–186. IEEE.
Lin, T.-Y., Doll
´
ar, P., Girshick, R., He, K., Hariharan, B.,
and Belongie, S. (2017a). Feature pyramid networks
for object detection. In Proceedings of the IEEE con-
ference on computer vision and pattern recognition,
pages 2117–2125.
Lin, T.-Y., Goyal, P., Girshick, R., He, K., and Doll
´
ar, P.
(2017b). Focal loss for dense object detection. In
Proceedings of the IEEE international conference on
computer vision, pages 2980–2988.
Liu, W., Anguelov, D., Erhan, D., Szegedy, C., Reed, S.,
Fu, C.-Y., and Berg, A. C. (2016). Ssd: Single shot
multibox detector. In European conference on com-
puter vision, pages 21–37. Springer.
Noh, J., Bae, W., Lee, W., Seo, J., and Kim, G. (2019). Bet-
ter to follow, follow to be better: Towards precise su-
pervision of feature super-resolution for small object
detection. In Proceedings of the IEEE/CVF Interna-
tional Conference on Computer Vision, pages 9725–
9734.
Pang, J., Li, C., Shi, J., Xu, Z., and Feng, H. (2019). R2-
cnn: Fast tiny object detection in large-scale remote
sensing images. IEEE Transactions on Geoscience
and Remote Sensing, 57(8):5512–5524.
Redmon, J., Divvala, S., Girshick, R., and Farhadi, A.
(2016). You only look once: Unified, real-time object
detection. In Proceedings of the IEEE conference on
computer vision and pattern recognition, pages 779–
788.
Ren, S., He, K., Girshick, R., and Sun, J. (2015). Faster
r-cnn: Towards real-time object detection with region
proposal networks. Advances in neural information
processing systems, 28:91–99.
Sun, S., Yin, Y., Wang, X., Xu, D., Zhao, Y., and
Shen, H. (2019). Multiple receptive fields and
small-object-focusing weakly-supervised segmenta-
tion network for fast object detection. arXiv preprint
arXiv:1904.12619.
Zhang, S., Zhu, X., Lei, Z., Shi, H., Wang, X., and Li, S. Z.
(2017). S3fd: Single shot scale-invariant face detector.
In Proceedings of the IEEE international conference
on computer vision, pages 192–201.
ICPRAM 2022 - 11th International Conference on Pattern Recognition Applications and Methods
504