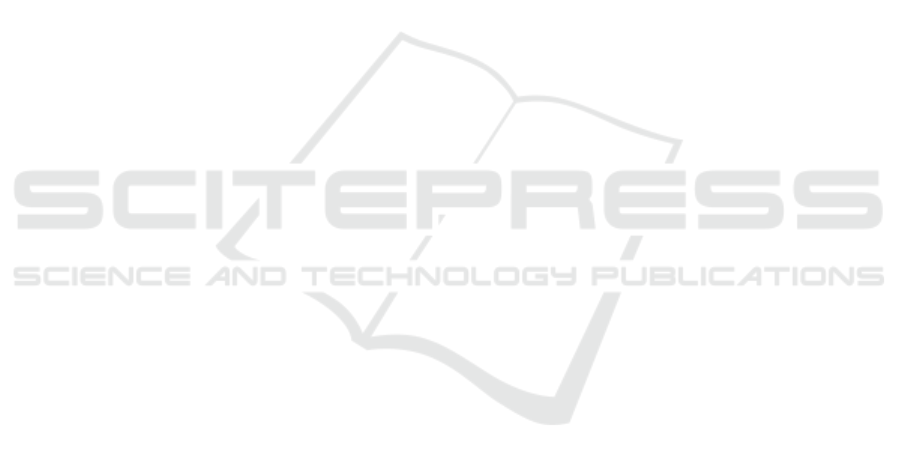
sign enabling direct load control for reserves. IEEE
Transactions on Power Systems, 31(3):2044–2054.
Kraning, M., Chu, E., Lavaei, J., and Boyd, S. (2014). Dy-
namic network energy management via proximal mes-
sage passing. Foundations and Trends in Optimiza-
tion, 1(2):73–126.
Liang, H. and Zhuang, W. (2014). Stochastic modeling
and optimization in a microgrid: A survey. Energies,
7(4):2027–2050.
Mahat, P., Escribano Jim
´
enez, J., Moldes, E. R., Haug,
S. I., Szczesny, I. G., Pollestad, K. E., and Totu, L. C.
(2013). A micro-grid battery storage management. In
2013 IEEE PES General Meeting.
McArthur, S. D., Davidson, E. M., Catterson, V. M.,
Dimeas, A. L., Hatziargyriou, N. D., Ponci, F., and
Funabashi, T. (2007a). Multi-agent systems for
power engineering applications-part i: Concepts, ap-
proaches, and technical challenges. IEEE Transac-
tions on Power systems, 22(4):1743–1752.
McArthur, S. D., Davidson, E. M., Catterson, V. M.,
Dimeas, A. L., Hatziargyriou, N. D., Ponci, F., and
Funabashi, T. (2007b). Multi-agent systems for power
engineering applications-part ii: Technologies, stan-
dards, and tools for building multi-agent systems.
IEEE Transactions on Power Systems, 22(4):1753–
1759.
McNamara, P. and McLoone, S. (2015). Hierarchical de-
mand response for peak minimization using dantzig–
wolfe decomposition. IEEE Transactions on Smart
Grid, 6(6):2807–2815.
Mesaric, P., Dukec, D., and Krajcar, S. (2017). Exploring
the potential of energy consumers in smart grid using
focus group methodology. Sustainability, 9(8):1463.
Meyn, S. P., Barooah, P., Bu
ˇ
si
´
c, A., Chen, Y., and Ehren,
J. (2015). Ancillary service to the grid using intel-
ligent deferrable loads. IEEE Transactions on Auto-
matic Control, 60(11):2847–2862.
Mihaylov, M., R
˘
adulescu, R., Razo-Zapata, I., Jurado, S.,
Arco, L., Avellana, N., and Now
´
e, A. (2019). Com-
paring stakeholder incentives across state-of-the-art
renewable support mechanisms. Renewable energy,
131:689–699.
Moghaddam, A. A., Seifi, A., Niknam, T., and Pahlavani,
M. R. A. (2011). Multi-objective operation manage-
ment of a renewable {MG} (micro-grid) with back-up
micro-turbine/fuel cell/battery hybrid power source.
Energy, 36(11):6490 – 6507.
Mohamed, F. A. and Koivo, H. N. (2007). System mod-
elling and online optimal management of microgrid
using multiobjective optimization. In Clean Electri-
cal Power, 2007. ICCEP’07. International Conference
on, pages 148–153. IEEE.
Moreno, R., Street, A., Arroyo, J. M., and Mancarella,
P. (2017). Planning low-carbon electricity systems
under uncertainty considering operational flexibility
and smart grid technologies. Phil. Trans. R. Soc. A,
375(2100):20160305.
National Renewable Energy Laboratory (2006a). So-
lar integration data sets. https://www.nrel.gov/grid/
solar-integration-data.html.
National Renewable Energy Laboratory (2006b). Wind
integration data sets. https://www.nrel.gov/grid/
wind-integration-data.html.
Paul M. Anderson, A. A. F. (2003). Power System Control
and Stability, 2nd. IEEE Press, New York.
Ramaswamy, P. and Deconinck, G. (2012). Smart grid
reconfiguration using simple genetic algorithm and
nsga-ii. In Innovative Smart Grid Technologies (ISGT
Europe), 2012 3rd IEEE PES International Confer-
ence and Exhibition on, pages 1–8.
Rivera, J., Goebel, C., and Jacobsen, H.-A. (2017). Dis-
tributed convex optimization for electric vehicle ag-
gregators. IEEE Transactions on Smart Grid.
Saad, W., Han, Z., Poor, H. V., and Basar, T. (2012). Game-
theoretic methods for the smart grid: An overview
of microgrid systems, demand-side management, and
smart grid communications. IEEE Signal Processing
Magazine, 29(5):86–105.
Sanseverino, E. R., Di Silvestre, M. L., Ippolito, M. G.,
De Paola, A., and Re, G. L. (2011). An execution,
monitoring and replanning approach for optimal en-
ergy management in microgrids. Energy, 36(5):3429–
3436.
Storn, R. and Price, K. (1997). Differential evolution–a
simple and efficient heuristic for global optimization
over continuous spaces. Journal of global optimiza-
tion, 11(4):341–359.
Tajalli, S. Z., Kavousi-Fard, A., Mardaneh, M., Khosravi,
A., and Razavi-Far, R. (2021). Uncertainty-aware
management of smart grids using cloud-based lstm-
prediction interval. IEEE Transactions on Cybernet-
ics.
Tuballa, M. L. and Abundo, M. L. (2016). A review of the
development of smart grid technologies. Renewable
and Sustainable Energy Reviews, 59:710 – 725.
Veldman, E., Gibescu, M., Slootweg, H. J., and Kling, W. L.
(2013). Scenario-based modelling of future residen-
tial electricity demands and assessing their impact on
distribution grids. Energy Policy, 56:233–247.
Zhang, J., Zhan, Z.-h., Lin, Y., Chen, N., Gong, Y.-j.,
Zhong, J.-h., Chung, H. S., Li, Y., and Shi, Y.-h.
(2011). Evolutionary computation meets machine
learning: A survey. IEEE Computational Intelligence
Magazine, 6(4):68–75.
Zhang, Y. and Giannakis, G. B. (2016). Distributed stochas-
tic market clearing with high-penetration wind power.
IEEE Transactions on Power Systems, 31(2):895–906.
Zhang, Y., Shen, S., and Mathieu, J. L. (2017). Distribu-
tionally robust chance-constrained optimal power flow
with uncertain renewables and uncertain reserves pro-
vided by loads. IEEE Transactions on Power Systems,
32(2):1378–1388.
Zitzler, E., Laumanns, M., and Thiele, L. (2001). SPEA2:
Improving the strength pareto evolutionary algorithm.
Technical Report 103, Computer Engineering and
Networks Laboratory (TIK), Swiss Federal Institute
of Technology (ETH), Zurich, Switzerland.
Towards a Robust, Distributed and Decentralised Smart Energy Management of Microgrids
25