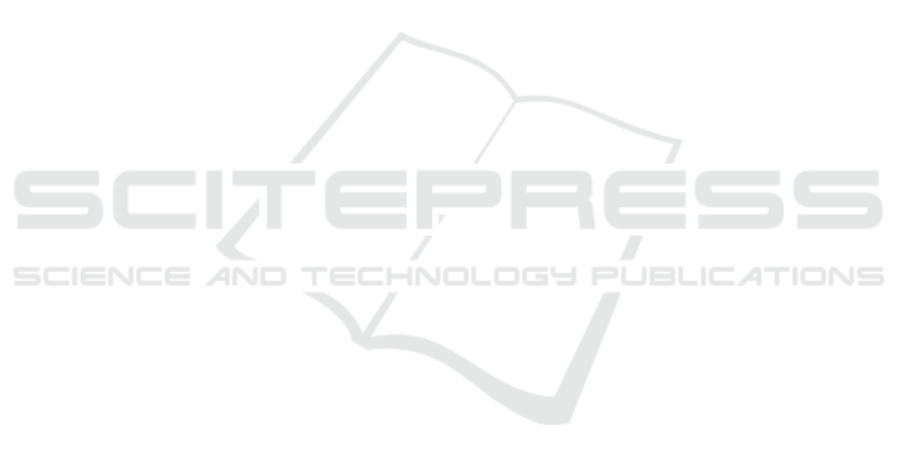
many with slime mold on 3-d terrains. IEEE Transac-
tions on Cybernetics, 44(1):126–136.
Adamatzky, A. and Prokopenko, M. (2012). Slime mould
evaluation of australian motorways. International
Journal of Parallel, Emergent and Distributed Sys-
tems, 27(4):275–295.
Clauset, A., Newman, M. E. J., and Moore, C. (2004). Find-
ing community structure in very large networks. Phys-
ical Review E, 70(6).
Duin, C. and Voß, S. (1994). Steiner tree heuristics — a sur-
vey. In Operations Research Proceedings 1993, pages
485–496, Berlin, Heidelberg. Springer Berlin Heidel-
berg.
Fowler, A. (2017). Improved qubo formulations for d-wave
quantum computing. Master’s thesis, University of
Auckland, Auckland, New Zealand.
Fritzke, B. (1994). A growing neural gas network learns
topologies. Advances in neural information process-
ing systems, 7:625–632.
Grover, L. K. (1996). A fast quantum mechanical algorithm
for database search. In Proceedings of the Twenty-
Eighth Annual ACM Symposium on Theory of Com-
puting, STOC ’96, page 212–219, New York, NY,
USA. Association for Computing Machinery.
Gupta, A. and K
¨
onemann, J. (2011). Approximation algo-
rithms for network design: A survey. Surveys in Op-
erations Research and Management Science, 16(1):3–
20.
Hagberg, A. A., Schult, D. A., and Swart, P. J. (2008). Ex-
ploring network structure, dynamics, and function us-
ing networkx. In Varoquaux, G., Vaught, T., and Mill-
man, J., editors, Proceedings of the 7th Python in Sci-
ence Conference, pages 11 – 15, Pasadena, CA USA.
Hwang, F. K. and Richards, D. S. (1992). Steiner tree prob-
lems. Networks, 22(1):55–89.
Kapsalis, A., Raywad-Smith, V., and Smith, G. D. (1993a).
Solving the graphical steiner tree problem using ge-
netic algorithms. Journal of the Operational Research
Society, 44(4):397–406.
Kapsalis, A., Rayward-Smith, V. J., and Smith, G. D.
(1993b). Solving the graphical steiner tree problem
using genetic algorithms. The Journal of the Opera-
tional Research Society, 44(4):397–406.
Lehner, B., Umlauf, G., and Hamann, B. (2008). Video
compression using data-dependent triangulations.
Leitner, M., Ljubi
´
c, I., Luipersbeck, M., and Resch, M.
(2014). A partition-based heuristic for the steiner tree
problem in large graphs. In International Workshop
on Hybrid Metaheuristics, pages 56–70. Springer.
Liu, L., Song, Y., Zhang, H., Ma, H., and Vasilakos, A.
(2015). Physarum optimization: A biology-inspired
algorithm for the steiner tree problem in networks.
IEEE Transactions on Computers, 64:819–832.
Lucas, A. (2014). Ising formulations of many np problems.
Frontiers in Physics, 2:5.
Madras, N. N. (2002). Lectures on monte carlo methods,
volume 16. American Mathematical Soc.
McGeoch, C. and Farr
´
e, P. (2020). The d-wave advantage
system: An overview. Technical Report 14-1049A-A,
D-Wave Systems Inc., Burnaby, BC, Canada.
Metropolis, N., Rosenbluth, A. W., Rosenbluth, M. N.,
Teller, A. H., and Teller, E. (1953). Equation of state
calculations by fast computing machines. The journal
of chemical physics, 21(6):1087–1092.
Miyamoto, M., Iwamura, M., Kise, K., and Gall, F. L.
(2020). Quantum speedup for the minimum steiner
tree problem. In Kim, D., Uma, R. N., Cai, Z., and
Lee, D. H., editors, Computing and Combinatorics,
pages 234–245, Cham. Springer International Pub-
lishing.
Promel, H. and Steger, A. (2002). The steiner tree problem:
A tour through graphs algorithms and complexity.
Pr
¨
omel, H. J. and Steger, A. (2012). The Steiner tree prob-
lem: a tour through graphs, algorithms, and complex-
ity. Springer Science & Business Media.
Rosenberg, M., French, T., Reynolds, M., and While, L.
(2021). A genetic algorithm approach for the eu-
clidean steiner tree problem with soft obstacles. In
Proceedings of the Genetic and Evolutionary Compu-
tation Conference, GECCO ’21, page 618–626, New
York, NY, USA. Association for Computing Machin-
ery.
Shi, J. and Malik, J. (2000). Normalized cuts and image
segmentation. IEEE Transactions on Pattern Analysis
and Machine Intelligence, 22(8):888–905.
Siebert, M., Ahmed, S., and Nemhauser, G. (2020). A sim-
ulated annealing algorithm for the directed steiner tree
problem.
Sun, Y. (2019). Solving the steiner tree problem in graphs
using physarum-inspired algorithms.
Venegas-Andraca, S. E., Cruz-Santos, W., McGeoch, C. C.,
and Lanzagorta, M. (2018). A cross-disciplinary in-
troduction to quantum annealing-based algorithms.
Contemporary Physics, 59(2):174–197.
Zelnik-Manor, L. and Perona, P. (2004). Self-tuning spec-
tral clustering. In Advances in Neural Information
Processing Systems 17, pages 1601–1608. MIT Press.
ICAART 2022 - 14th International Conference on Agents and Artificial Intelligence
32