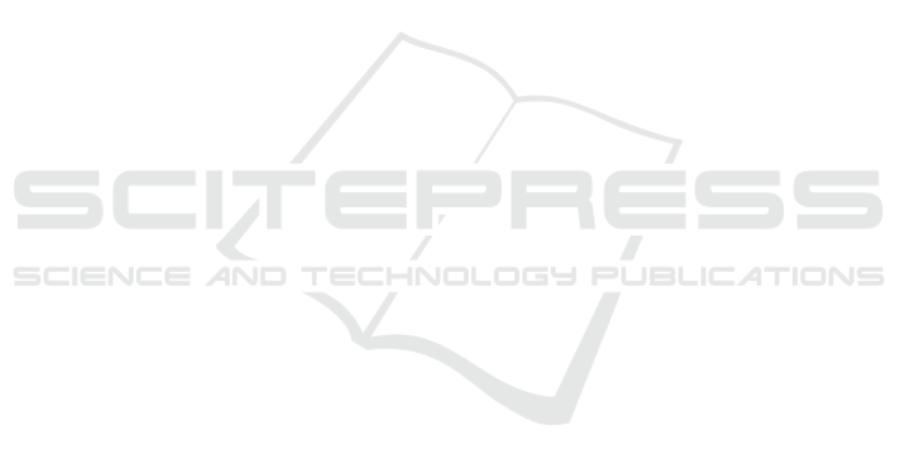
known metrics and others that capture the particu-
larities of CEILS that confirm the efficiency of our
method in generating feasible actions with respect to
its baseline counterfactual generator.
Despite the growing research on the field of coun-
terfactual explanations, there are a lot of open ques-
tions and challenges yet to be tackled (Verma et al.,
2020). In particular, we are interested in relaxing
the assumption of having a complete and reliable
causal graph and work with incomplete causal rela-
tions. Moreover, as for future work, we consider to
extend our evaluation by employing other counterfac-
tual generators as baselines to analyze how it could
contribute to the overall results, and also to compare
the explanations produced by other causal methods
with the ones found with CEILS, and possibly involv-
ing end users to obtain feedback that will guide to-
wards better explanations. The preprint (Crupi et al.,
2021) includes detailed evaluations and experiments
on additional datasets.
REFERENCES
Arrieta, A. B., D
´
ıaz-Rodr
´
ıguez, N., Del Ser, J., Bennetot,
A., Tabik, S., Barbado, A., Garc
´
ıa, S., Gil-L
´
opez, S.,
Molina, D., Benjamins, R., et al. (2020). Explainable
Artificial Intelligence (XAI): Concepts, taxonomies,
opportunities and challenges toward responsible AI.
Information Fusion, 58:82–115.
Barocas, S., Selbst, A. D., and Raghavan, M. (2020). The
hidden assumptions behind counterfactual explana-
tions and principal reasons. In Proceedings of the
2020 Conference on Fairness, Accountability, and
Transparency, pages 80–89.
Castelnovo, A., Crupi, R., Del Gamba, G., Greco, G.,
Naseer, A., Regoli, D., and Gonzalez, B. S. M. (2020).
Befair: Addressing fairness in the banking sector. In
2020 IEEE International Conference on Big Data (Big
Data), pages 3652–3661. IEEE.
Crupi, R., Castelnovo, A., Regoli, D., and San
Miguel Gonz
´
alez, B. (2021). Counterfactual expla-
nations as interventions in latent space. arXiv preprint
arXiv:2106.07754.
Eberhardt, F. and Scheines, R. (2007). Interventions and
causal inference. Philosophy of science, 74(5):981–
995.
Gunning, D. and Aha, D. (2019). DARPA’s explainable
artificial intelligence (XAI) program. AI Magazine,
40(2):44–58.
High-Level Expert Group on AI (2019). Ethics
guidelines for trustworthy AI. https:
//ec.europa.eu/digital-single-market/en/news/
ethics-guidelines-trustworthy-ai.
Joshi, S., Koyejo, O., Vijitbenjaronk, W., Kim, B.,
and Ghosh, J. (2019). Towards realistic individ-
ual recourse and actionable explanations in black-
box decision making systems. arXiv preprint
arXiv:1907.09615.
Kalainathan, D. and Goudet, O. (2019). Causal discov-
ery toolbox: Uncover causal relationships in python.
arXiv preprint arXiv:1903.02278.
Kalainathan, D., Goudet, O., and Dutta, R. (2020). Causal
Discovery Toolbox: Uncovering causal relationships
in Python. Journal of Machine Learning Research,
21(37):1–5.
Kalisch, M., M
¨
achler, M., Colombo, D., Maathuis, M. H.,
and B
¨
uhlmann, P. (2012). Causal inference using
graphical models with the R package pcalg. Journal
of Statistical Software, 47:1–26.
Karimi, A.-H., Sch
¨
olkopf, B., and Valera, I. (2020). Algo-
rithmic recourse: from counterfactual explanations to
interventions. arXiv preprint arXiv:2002.06278.
Klaise, J., Van Looveren, A., Vacanti, G., and Coca, A.
(2019). Alibi: Algorithms for monitoring and ex-
plaining machine learning models. https://github.com/
SeldonIO/alibi.
Mahajan, D., Tan, C., and Sharma, A. (2019). Pre-
serving causal constraints in counterfactual explana-
tions for machine learning classifiers. arXiv preprint
arXiv:1912.03277.
Mothilal, R. K., Sharma, A., and Tan, C. (2020). Explain-
ing machine learning classifiers through diverse coun-
terfactual explanations. In Proceedings of the 2020
Conference on Fairness, Accountability, and Trans-
parency, pages 607–617.
Pearl, J. et al. (2000). Models, reasoning and inference.
Cambridge, UK: CambridgeUniversityPress, 19.
Pearl, J., Glymour, M., and Jewell, N. P. (2016). Causal
inference in statistics: A primer. John Wiley & Sons.
Peters, J., Mooij, J. M., Janzing, D., and Sch
¨
olkopf, B.
(2014). Causal discovery with continuous additive
noise models. Journal of Machine Learning Research,
15(58).
Stepin, I., Alonso, J. M., Catala, A., and Pereira-Fari
˜
na, M.
(2021). A survey of contrastive and counterfactual ex-
planation generation methods for explainable artificial
intelligence. IEEE Access, 9:11974–12001.
The European Union (2016). EU General Data Protec-
tion Regulation (GDPR): Regulation (EU) 2016/679
of the European Parliament and of the Council of 27
April 2016 on the protection of natural persons with
regard to the processing of personal data and on the
free movement of such data, and repealing Directive
95/46/EC (General Data Protection Regulation). Offi-
cial Journal of the European Union. http://data.europa.
eu/eli/reg/2016/679/2016-05-04.
Ustun, B., Spangher, A., and Liu, Y. (2019). Actionable re-
course in linear classification. In Proceedings of the
Conference on Fairness, Accountability, and Trans-
parency, pages 10–19.
Van Looveren, A. and Klaise, J. (2019). Interpretable coun-
terfactual explanations guided by prototypes. arXiv
preprint arXiv:1907.02584.
Verma, S., Dickerson, J., and Hines, K. (2020). Counter-
factual explanations for machine learning: A review.
arXiv preprint arXiv:2010.10596.
Leveraging Causal Relations to Provide Counterfactual Explanations and Feasible Recommendations to End Users
31