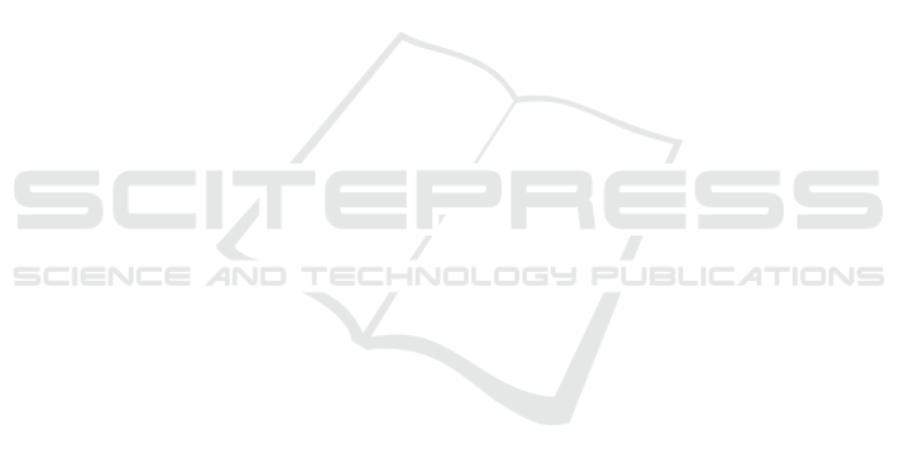
lize an open-source face detection framework Caffe
(Jia et al., 2014). Individual frames are then fed
into the face detector which detects and crops the
subject faces. We then utilise our trained Impres-
sionNet model in order to predict the attribute scores
on these face images and annotate the scores on the
original frame. The individual annotated frames are
merged back to form the video in real-time. Fig. 3
shows several frames from a processed video using
the above approach. We have also provided few an-
notated videos from diverse social settings as supple-
mentary material.
6 CONCLUSION
In this paper, we have proposed a fresh multi-view ap-
proach for analysis and prediction of social attributes
like trustworthiness and dominance based on facial
features. The proposed approach achieves superior
feature generalisation and diversification resulting in
improved coefficient of determination (R
2
) scores.
Our experiments validate that one can extract more di-
verse features using multiple views and subsequently
improve the performance by combining their results
in regressive tasks as well. To justify the diversifi-
cation and generalisation ability of our approach, we
have also performed the ablation study. The obtained
results clearly establish the effectiveness of this ap-
proach and also indicates that similar methods can
also be used for analogous tasks. At last, we also
proposed a method which enables the real-time video
analysis of multiple subject faces and can have several
applications in marketing, surveillance and more.
7 SUPPLEMENTAL MATERIAL
More results and evaluations on video sequences of
various test subjects can be seen in the supplemental
annotated videos from the link below. We show
examples of various social interactions and how it
affects the perception of the social attributes like
trustworthiness based on the facial expressions
analysed by our multi-view regression approach
(see, for example, the fluctuations in trustwor-
thiness scores associated in tense situations such
as in political interviews or broadcasting studios
when the subject is judged highly in Videos).
https://anonymous.4open.science/r/3a48498b-871f-
4484-93dc-c9982e11fd65/README.md
REFERENCES
A, T., R, D., JM, P., NN, O., and VB., F. (2013). Validation
of data-driven computational models of social percep-
tion of faces. Emotion.
Chollet, Francois, et al. (2015). Keras. GitHub.
Deng, Jiankang, Guo, Jia, Ververas, Evangelos, Kotsia,
Irene, Zafeiriou, and Stefanos (2020). Retinaface:
Single-shot multi-level face localisation in the wild.
In Proceedings of the IEEE/CVF Conference on Com-
puter Vision and Pattern Recognition (CVPR). IEEE
Computer Society.
Frith, C. (2009). Role of facial expressions in social inter-
actions. The Royal Society.
G, B. (2000). The OpenCV Library. Dr. Dobb’s Journal of
Software Tools.
Jia, Y., Shelhamer, E., Donahue, J., Karayev, S., Long,
J., Girshick, R., Guadarrama, S., Darrell, and Trevor
(2014). Caffe: Convolutional Architecture for Fast
Feature Embedding. ACM.
Keating, C. F., Mazur, A., Segall, M. H., Cysneiros, P. G.,
Kilbride, J. E., Leahy, P., Divale, W. T., Komin, S.,
Thurman, B., and Wirsing, R. (1981). Culture and
the perception of social dominance from facial expres-
sion. Journal of Personality and Social Psychology.
Liu, Z., Luo, P., Wang, X., , and Tang, X. (2015). Deep
learning face attributes in the wild. In Proceedings of
the IEEE International Conference on Computer Vi-
sion. IEEE Computer Society.
Ma, Correll, and Wittenbrink (2015). The Chicago Face
Database: A Free Stimulus Set of Faces and Norming
Data. Behavior Research Methods.
McCurrie, M., Beletti, F., Parzianello, L., Westendorp, A.,
Anthony, S., and Scheirer, W. (2017). Predicting first
impressions with deep learning. In 12th IEEE In-
ternational Conference on Automatic Face & Gesture
Recognition. IEEE Computer Society.
Niu, Xuesong, Han, Hu, Shan, Shiguang, Chen, and
Xilin (2019). Multi-label co-regularization for semi-
supervised facial action unit recognition. In Proceed-
ings of the Advances in Neural Information Process-
ing Systems (NeurIPS).
O, C., B, L., M, E., J, R., G, L., H, M., F, W., and S, L.
(2014). Rewards of Beauty: The Opioid System Medi-
ates Social Motivation in Humans. Mol Psychiatry.
Safra, L., Chevallier, C., Gr
`
ezes, J., and Baumard, N.
(2020). Tracking historical changes in trustworthi-
ness using machine learning analyses of facial cues in
painting. Nature Communications.
Tan, M. and Le, Q. V. (2019). Efficientnet: Rethinking
model scaling for convolutional neural networks. In
Proceedings of the 36th International Conference on
Machine Learning (ICML).
van der Maaten, L. and Hinton, G. (2008). Visualizing data
using t-SNE. Journal of machine learning research.
Xu, C., Tao, D., and Xu, C. (2013). A Survey on Multi-view
Learning. CoRR.
ICAART 2022 - 14th International Conference on Agents and Artificial Intelligence
38