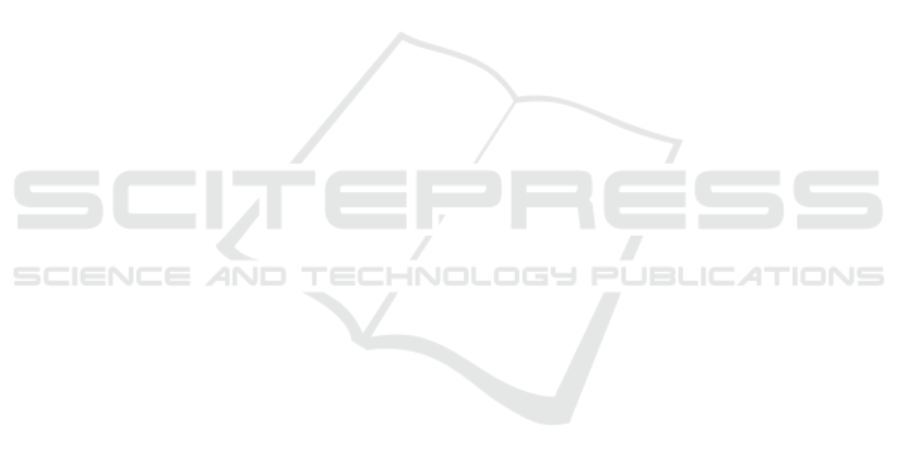
add the calculation logic of bilinear, and the parame-
ters of the model have also been relatively increased.
However, compared to the improvement of the model
performance, we believe that this conversion is cost-
effective.
6 CONCLUSION
We propose an end-to-end Bilinear Multi-Head At-
tention Graph Neural Network for Traffic Prediction,
which not only utilize the linear weighted neighbor
nodes to represent the target spatial and temporal
node, but also use the bilinear aggregator in spatial
and temporal representations. Extensive experiments
are carried out on two real-word traffic datasets, and
the results show that our proposed model achieves the
state-of-the-art performance in most scenes. For fu-
ture work, we will consider encoding high-order in-
teractions among multiple neighbors to represent the
target node and apply our model to other related ap-
plications.
ACKNOWLEDGEMENTS
This work was supported by the National Key R&D
Program of China under Grant No.2018AAA0101204
and No.2018AAA0101200. Kai Han is the corre-
sponding author.
REFERENCES
Atwood, J. and Towsley, D. (2015). Search-convolutional
neural networks. volume abs/1511.02136.
Beutel, A., Covington, P., Jain, S., Xu, C., Li, J., Gatto, V.,
and Chi, E. H. (2018). Latent cross: Making use of
context in recurrent recommender systems. In WSDM,
pages pages 46–54.
Bruna, J., Zaremba, W., Szlam, A., and Lecun, Y. (2014).
Spectral networks and locally connected networks on
graphs. In International Conference on Learning Rep-
resentations (ICLR2014), CBLS, April 2014.
Chen, W., Chen, L., Xie, Y., Cao, W., Gao, Y., and Feng,
X. (2019). Multi-range attentive bicomponent graph
convolutional network for traffic forecasting. volume
abs/1911.12093.
Chun-Hsin Wu, Jan-Ming Ho, and Lee, D. T. (2004).
Travel-time prediction with support vector regression.
volume 5, pages 276–281.
Chung, J., G
¨
ulc¸ehre, C¸ ., Cho, K., and Bengio, Y. (2014).
Empirical evaluation of gated recurrent neural net-
works on sequence modeling. volume abs/1412.3555.
D., N., N., N., Y., G., , and H., K. (2005). Univariate short-
term prediction of road travel times. In In Proceed-
ings of IEEE Intelligent Transportation Systems Con-
ference, pages 1074–1079.
Grover, A. and Leskovec, J. (2016). node2vec:
Scalable feature learning for networks. volume
abs/1607.00653.
J.D., H. (1994). Time series analysis. volume Volume 2,
pages 690–696.
Kingma, D. and Ba, J. (2014). Adam: A method for
stochastic optimization.
Li, Y., Yu, R., Shahabi, C., and Liu, Y. (2018). Diffusion
convolutional recurrent neural network: Data-driven
traffic forecasting. In International Conference on
Learning Representations (ICLR ’18).
Ma, X., Dai, Z., He, Z., and Wang, Y. (2017). Learning
traffic as images: A deep convolution neural network
for large-scale transportation network speed predic-
tion. volume abs/1701.04245.
Nikovski, D., Nishiuma, N., Goto, Y., and Kumazawa, H.
(2005). Univariate short-term prediction of road travel
times. In Proceedings. 2005 IEEE Intelligent Trans-
portation Systems, 2005., pages 1074–1079.
Oreshkin, B. N., Amini, A., Coyle, L., and Coates, M. J.
(2020). Fc-gaga: Fully connected gated graph archi-
tecture for spatio-temporal traffic forecasting.
Q., Z., J., C., G., M., S., X., and C., P. (2020). Spatio-
temporal graph structure learning for traffic forecast-
ing. In Proceedings of the AAAI Conference on Artifi-
cial Intelligence, pages 1177–1185.
Rendle, S. (2010). Factorization machines. In 2010 IEEE
International Conference on Data Mining, pages 995–
1000.
S., G., Y., L., N., F., C., S., and H., W. (2019). Attention
based spatial-temporal graph convolutional networks
for traffic flow forecasting. pages 922–929.
Shuman, D. I., Narang, S. K., Frossard, P., Ortega, A.,
and Vandergheynst, P. (2012). Signal processing on
graphs: Extending high-dimensional data analysis to
networks and other irregular data domains. volume
abs/1211.0053.
S.I.J., C. and C.M., K. (2003). Dynamic travel time pre-
diction with real-time and historic data. In Journal of
Transportation Engineering 129(6), pages 608–616.
Song, C., Lin, Y., Guo, S., and Wan, H. (2020). Spatial-
temporal synchronous graph convolutional networks:
A new framework for spatial-temporal network data
forecasting. volume 34, pages 914–921.
Sutskever, I., Vinyals, O., and Le, Q. V. (2014). Sequence to
sequence learning with neural networks. In Proceed-
ings of the 27th International Conference on Neural
Information Processing Systems - Volume 2, NIPS’14,
page 3104–3112, Cambridge, MA, USA. MIT Press.
Vaswani, A., Shazeer, N., Parmar, N., Uszkoreit, J., Jones,
L., Gomez, A. N., Kaiser, u., and Polosukhin, I.
(2017). Attention is all you need. In Proceedings of
the 31st International Conference on Neural Informa-
tion Processing Systems, NIPS’17, page 6000–6010,
Red Hook, NY, USA. Curran Associates Inc.
ICAART 2022 - 14th International Conference on Agents and Artificial Intelligence
42