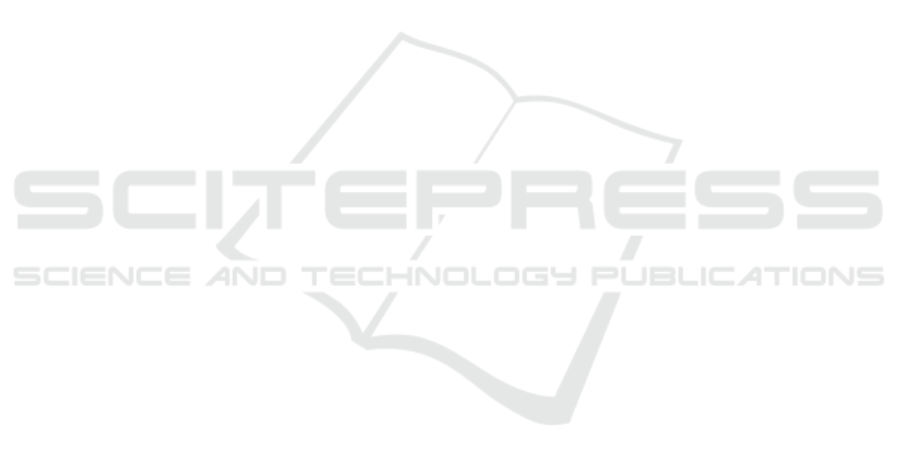
2018-00008), as well as grant NKFIH-1279-2/2020.
Both authors contributed equally.
REFERENCES
Alipanahi, B., Delong, A., Weirauch, M. T., and Frey, B. J.
(2015). Predicting the sequence specificities of DNA-
and rna-binding proteins by deep learning. Nature
biotechnology, 33(8):831–838.
Bernstein, B., Birney, E., Dunham, I., Green, E., Gunter, C.,
and Snyder, M. (2012). Consortium ep. an integrated
encyclopedia of DNA elements in the human genome.
Nature, 489(7414):57–74.
Brendel, W., Rauber, J., K
¨
ummerer, M., Ustyuzhaninov, I.,
and Bethge, M. (2019). Accurate, reliable and fast ro-
bustness evaluation. In Wallach, H., Larochelle, H.,
Beygelzimer, A., d Alch
´
e-Buc, F., Fox, E., and Gar-
nett, R., editors, Advances in Neural Information Pro-
cessing Systems, volume 32. Curran Associates, Inc.
Fornes, O., Castro-Mondragon, J. A., Khan, A.,
van der Lee, R., Zhang, X., Richmond, P. A., Modi,
B. P., Correard, S., Gheorghe, M., Barana
ˇ
si
´
c, D.,
Santana-Garcia, W., Tan, G., Ch
`
eneby, J., Ballester,
B., Parcy, F., Sandelin, A., Lenhard, B., Wasser-
man, W. W., and Mathelier, A. (2019). JASPAR
2020: update of the open-access database of transcrip-
tion factor binding profiles. Nucleic Acids Research,
48(D1):D87–D92.
Hassanzadeh, H. R. and Wang, M. D. (2016). Deeperbind:
Enhancing prediction of sequence specificities of dna
binding proteins. In 2016 IEEE International Con-
ference on Bioinformatics and Biomedicine (BIBM),
pages 178–183. IEEE.
Kauderer-Abrams, E. (2017). Quantifying translation-
invariance in convolutional neural networks. arXiv
preprint arXiv:1801.01450.
Koo, P. K. and Ploenzke, M. (2020). Deep learning for
inferring transcription factor binding sites. Current
Opinion in Systems Biology, 19:16–23.
Lanchantin, J., Singh, R., Wang, B., and Qi, Y. (2017).
Deep motif dashboard: Visualizing and understand-
ing genomic sequences using deep neural networks.
In Pacific Symposium on Biocomputing 2017, pages
254–265. World Scientific.
Morris, J., Lifland, E., Yoo, J. Y., Grigsby, J., Jin, D., and
Qi, Y. (2020). Textattack: A framework for adversar-
ial attacks, data augmentation, and adversarial train-
ing in nlp. In Proceedings of the 2020 Conference on
Empirical Methods in Natural Language Processing:
System Demonstrations, pages 119–126.
Park, S., Koh, Y., Jeon, H., Kim, H., Yeo, Y., and Kang, J.
(2020). Enhancing the interpretability of transcription
factor binding site prediction using attention mecha-
nism. Scientific Reports, 10(1):13413.
Quang, D. and Xie, X. (2019). Factornet: A deep learn-
ing framework for predicting cell type specific tran-
scription factor binding from nucleotide-resolution se-
quential data. Methods, 166:40–47. Deep Learning in
Bioinformatics.
Stormo, G. D. (2000). DNA binding sites: representation
and discovery. Bioinformatics, 16(1):16–23.
Zeng, H., Edwards, M., Liu, G., and Gifford, D.
(2016). Convolutional neural network architectures
for predicting DNA-protein binding. Bioinformatics,
32:i121–i127.
Zhang, X. (2013). Position weight matrices. In Dubitzky,
W., Wolkenhauer, O., Cho, K.-H., and Yokota, H., ed-
itors, Encyclopedia of Systems Biology, pages 1721–
1722. Springer New York, New York, NY.
Zhou, J. and Troyanskaya, O. (2015). Predicting effects
of noncoding variants with deep learning-based se-
quence model. Nature methods, 12.
Translational Robustness of Neural Networks Trained for Transcription Factor Binding Site Classification
45