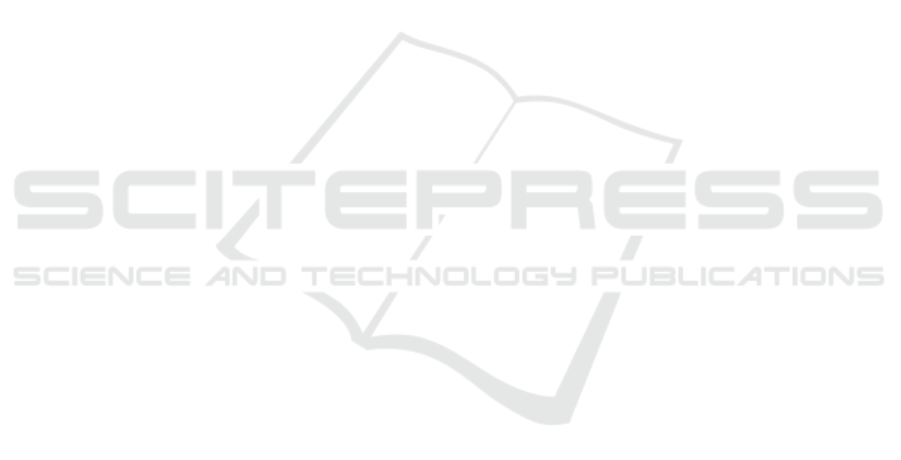
ACKNOWLEDGEMENTS
This work was supported by JSPS Kakenhi Grant
Number 20H04295, 20K20406, and 20K20625.
REFERENCES
Bishop, C. M. (2006). Pattern Recognition and Ma-
chine Learning (Information Science and Statistics).
Springer-Verlag.
Blei, D. M., Ng, A. Y., and Jordan, M. I. (2003). La-
tent dirichlet allocation. J. Mach. Learn. Res., 3:993–
1022.
Dang, T.-B., Nguyen, H.-T., and Nguyen, L.-M. (2020). La-
tent topic refinement based on distance metric learn-
ing and semantics-assisted non-negative matrix factor-
ization. In Proceedings of the 34th Pacific Asia Con-
ference on Language, Information and Computation,
pages 70–75, Hanoi, Vietnam. Association for Com-
putational Linguistics.
Deriu, J. M. and Cieliebak, M. (2017). SwissAlps at
SemEval-2017 task 3: Attention-based convolutional
neural network for community question answering.
In Proceedings of the 11th International Workshop
on Semantic Evaluation (SemEval-2017), pages 334–
338. Association for Computational Linguistics.
Devlin, J., Chang, M.-W., Lee, K., and Toutanova, K.
(2019). BERT: Pre-training of deep bidirectional
transformers for language understanding. In Proceed-
ings of the 2019 Conference of the North American
Chapter of the Association for Computational Lin-
guistics: Human Language Technologies, Volume 1
(Long and Short Papers), pages 4171–4186. Associ-
ation for Computational Linguistics.
Dolan, W. B. and Brockett, C. (2005). Automatically
constructing a corpus of sentential paraphrases. In
Proceedings of the Third International Workshop on
Paraphrasing (IWP2005).
Filice, S., Da San Martino, G., and Moschitti, A. (2017).
KeLP at SemEval-2017 task 3: Learning pairwise pat-
terns in community question answering. In Proceed-
ings of the 11th International Workshop on Semantic
Evaluation (SemEval-2017), pages 326–333. Associ-
ation for Computational Linguistics.
Hoffman, M., Bach, F., and Blei, D. (2010). Online learning
for latent dirichlet allocation. In Advances in Neural
Information Processing Systems, volume 23. Curran
Associates, Inc.
Humeau, S., Shuster, K., Lachaux, M.-A., and Weston, J.
(2020). Poly-encoders: Architectures and pre-training
strategies for fast and accurate multi-sentence scoring.
In International Conference on Learning Representa-
tions.
Nakov, P., Hoogeveen, D., M
`
arquez, L., Moschitti, A.,
Mubarak, H., Baldwin, T., and Verspoor, K. (2017).
SemEval-2017 task 3: Community question answer-
ing. In Proceedings of the 11th International Work-
shop on Semantic Evaluation (SemEval-2017), pages
27–48. Association for Computational Linguistics.
Nakov, P., M
`
arquez, L., Magdy, W., Moschitti, A., Glass,
J., and Randeree, B. (2015). SemEval-2015 task 3:
Answer selection in community question answering.
In Proceedings of the 9th International Workshop on
Semantic Evaluation (SemEval 2015), pages 269–281.
Association for Computational Linguistics.
Nakov, P., M
`
arquez, L., Moschitti, A., Magdy, W.,
Mubarak, H., Freihat, A. A., Glass, J., and Randeree,
B. (2016). SemEval-2016 task 3: Community ques-
tion answering. In Proceedings of the 10th Interna-
tional Workshop on Semantic Evaluation (SemEval-
2016), pages 525–545. Association for Computational
Linguistics.
Ovsjanikov, M. and Chen, Y. (2010). Topic modeling
for personalized recommendation of volatile items.
In Machine Learning and Knowledge Discovery in
Databases, pages 483–498. Springer Berlin Heidel-
berg.
Peinelt, N., Nguyen, D., and Liakata, M. (2020). tBERT:
Topic models and BERT joining forces for semantic
similarity detection. In Proceedings of the 58th An-
nual Meeting of the Association for Computational
Linguistics, pages 7047–7055, Online. Association
for Computational Linguistics.
Qin, Z., Thint, M., and Huang, Z. (2009). Ranking answers
by hierarchical topic models. In Next-Generation
Applied Intelligence, pages 103–112. Springer Berlin
Heidelberg.
Reimers, N. and Gurevych, I. (2019). Sentence-BERT:
Sentence embeddings using Siamese BERT-networks.
In Proceedings of the 2019 Conference on Empirical
Methods in Natural Language Processing and the 9th
International Joint Conference on Natural Language
Processing (EMNLP-IJCNLP), pages 3982–3992. As-
sociation for Computational Linguistics.
Schroff, F., Kalenichenko, D., and Philbin, J. (2015).
Facenet: A unified embedding for face recognition
and clustering. In 2015 IEEE Conference on Com-
puter Vision and Pattern Recognition (CVPR), pages
815–823.
Tan, C., Wei, F., Wang, W., Lv, W., and Zhou, M. (2018).
Multiway attention networks for modeling sentence
pairs. In Proceedings of the Twenty-Seventh Inter-
national Joint Conference on Artificial Intelligence,
IJCAI-18, pages 4411–4417. International Joint Con-
ferences on Artificial Intelligence Organization.
Tran, Q. H., Tran, V. D., Vu, T. T., Nguyen, M. L., and
Pham, S. B. (2015). JAIST: Combining multiple
features for answer selection in community question
answering. In Proceedings of the 9th International
Workshop on Semantic Evaluation (SemEval 2015),
pages 215–219, Denver, Colorado. Association for
Computational Linguistics.
Wang, Z., Hamza, W., and Florian, R. (2017). Bilateral
multi-perspective matching for natural language sen-
tences. In Proceedings of the Twenty-Sixth Inter-
national Joint Conference on Artificial Intelligence,
IJCAI-17, pages 4144–4150.
Wu, G., Sheng, Y., Lan, M., and Wu, Y. (2017). ECNU
at SemEval-2017 task 3: Using traditional and deep
ICAART 2022 - 14th International Conference on Agents and Artificial Intelligence
96