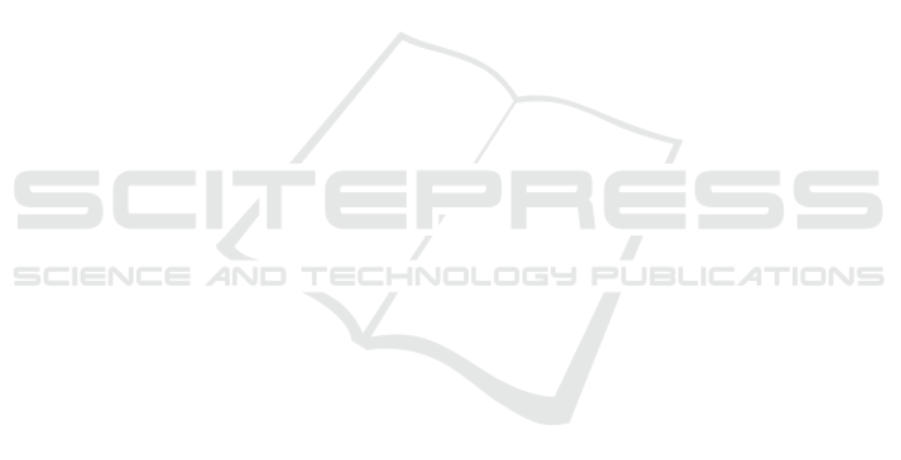
5 CONCLUSION
Traffic classification is a problem that can help im-
prove the security and quality of service aspects of
home networks. This paper presented a study consid-
ering two types of IoT and non-IoT (mobile and PCs).
We evaluated the performance of supervised models
in two tasks: learning the impact of different non-IoT
devices on traffic classification and ii) identifying a
new IoT device type. Our results suggest that models
trained with mixed non-IoT traffic benefit the classi-
fier. Despite the particularities of some IoT device
traffic, some algorithms could identify a new IoT de-
vice with reasonable accuracy. We intend to apply the
results in the anomaly detection problem and analyze
attack flows on different types of devices for future
work.
REFERENCES
Ahmed, M., Naser Mahmood, A., and Hu, J. (2016). A sur-
vey of network anomaly detection techniques. Journal
of Network and Computer Applications, 60:19–31.
Al Khater, N. and Overill, R. E. (2016). Network traffic
classification techniques and challenges. The 10th In-
ternational Conference on Digital Information Man-
agement, (Icdim):43–48.
Apthorpe, N., Reisman, D., and Feamster, N. (2017). A
smart home is no castle: Privacy vulnerabilities of en-
crypted iot traffic. arXiv preprint arXiv:1705.06805.
Cherif, I. L. and Kortebi, A. (2019). On using eXtreme Gra-
dient Boosting (XGBoost) Machine Learning algo-
rithm for Home Network Traffic Classification. IFIP
Wireless Days, pages 1–6.
Cviti
´
c, I., Perakovi
´
c, D., Peri
ˇ
sa, M., and Gupta, B.
(2021). Ensemble machine learning approach for clas-
sification of IoT devices in smart home. Interna-
tional Journal of Machine Learning and Cybernetics,
(0123456789).
Draper-Gil, G., Lashkari, A. H., Mamun, M. S. I., and Ghor-
bani, A. A. (2016). Characterization of encrypted and
VPN traffic using time-related features. Proceedings
of the 2nd International Conference on Information
Systems Security and Privacy, pages 407–414.
Holbrook, L. and Alamaniotis, M. (2019). Internet of things
security analytics and solutions with deep learning.
In Proceedings of the 31st IEEE International Con-
ference on Tools with Artificial Intelligence (ICTAI
2019), pages 178–185.
Karagiannis, T., Broido, A., Faloutsos, M., and Claffy, K.
(2004). Transport layer identification of P2P traffic.
Proceedings of the 2004 ACM SIGCOMM Internet
Measurement Conference, pages 121–134.
Kurose, J. F. and Ross, K. W. (2016). Computer Network-
ing: A Top-Down Approach. Pearson, 7th edition.
Lashkari, A. H., Gil, G. D., Mamun, M. S. I., and Ghorbani,
A. A. (2017). Characterization of tor traffic using time
based features. In Proceedings of the 3rd International
Conference on Information Systems Security and Pri-
vacy, pages 253–262.
Lashkari, A. H., Kadir, A. F. A., Taheri, L., and Ghor-
bani, A. A. (2018). Toward developing a system-
atic approach to generate benchmark android malware
datasets and classification. In Proceedings of the 2018
International Carnahan Conference on Security Tech-
nology, pages 1–7.
Liu, Y., Wang, J., Li, J., Niu, S., and Song, H. (2021). Ma-
chine Learning for the Detection and Identification of
Internet of Things (IoT) Devices: A Survey. IEEE
Internet of Things Journal, 7(5):1–23.
Ren, X., Gu, H., and Wei, W. (2021). Tree-RNN: Tree
structural recurrent neural network for network traf-
fic classification. Expert Systems with Applications,
167:114363.
Shahid, M. R., Blanc, G., Zhang, Z., and Debar, H. (2018).
IoT Devices Recognition Through Network Traffic
Analysis. Proceedings of the 2018 IEEE International
Conference on Big Data, pages 5187–5192.
Sivanathan, A., Gharakheili, H. H., Loi, F., Radford, A.,
Wijenayake, C., Vishwanath, A., and Sivaraman, V.
(2019). Classifying IoT devices in smart environments
using network traffic characteristics. IEEE Transac-
tions on Mobile Computing, 18(8):1745–1759.
Soysal, M. and Schmidt, E. G. (2010). Machine learning al-
gorithms for accurate flow-based network traffic clas-
sification: Evaluation and comparison. Performance
Evaluation, 67(6):451–467.
Sperotto, A., Schaffrath, G., Sadre, R., Morariu, C., Pras,
A., and Stiller, B. (2010). An overview of ip flow-
based intrusion detection. IEEE communications sur-
veys & tutorials, 12(3):343–356.
Tahaei, H., Afifi, F., Asemi, A., Zaki, F., and Anuar, N. B.
(2020). The rise of traffic classification in IoT net-
works: A survey. Journal of Network and Computer
Applications, 154.
ICAART 2022 - 14th International Conference on Agents and Artificial Intelligence
120